A Convolutional and Recurrent Neural Network-Based Control Algorithm for ankle exoskeleton: Validation of performance using IMU-based gait analysis.
Workshop on Metrology for Industry 4.0 and IoT(2024)
摘要
The development of exoskeletons control algorithms is a challenging task due to the inevitable interaction between robot and human. Data-driven approaches represent a promising alternative and complement to traditional methods, offering new avenues for discerning user intentions and enhancing controller design. Machine learning models have shown significant efficacy in interpreting data from wearable sensors, leading to advancements in this field. In the present work, we present a Convolutional and Recurrent Neural Network (CRNN) to predict continuous gait phase (GP) based on data coming from a single shank-mounted intertial measurment unit (IMU). Eleven healthy subject were enrolled in the training data collection. The validation employed a verified IMU-based algorithm that detects four primary gait events: heel strike, mid-stance, toe-off, and midswing. Fourteen healthy subjects participated in the validation. The CRNN accurately predicted the continuous GP with an overall RMSE of 0.7% throughout the gait cycle. Compared to the validation algorithm, the CRNN achieved a mean difference of 0.4% and a standard deviation of 3.2% with the algorithm output within all four gait phases, best performing on mid-swing. The results demonstrate the feasibility of the proposed method for implementation in an exoskeleton control algorithm.
更多查看译文
关键词
convolutional recurrent neural networks,IMU,wearables sensors,gait analysis,deep learning,ankle exoskeleton
AI 理解论文
溯源树
样例
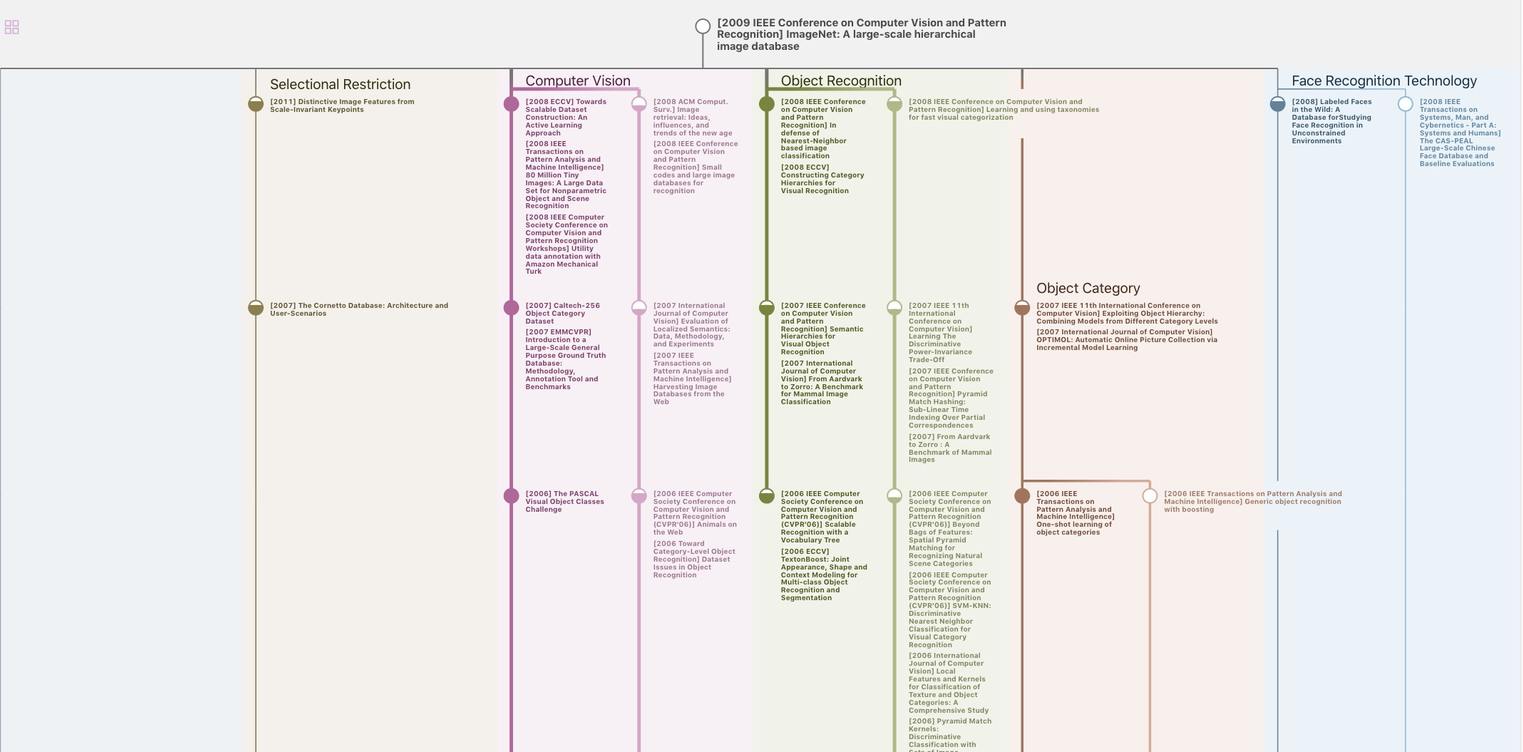
生成溯源树,研究论文发展脉络
Chat Paper
正在生成论文摘要