Survival-LCS: A Rule-Based Machine Learning Approach to Survival Analysis
GECCO '24 Proceedings of the Genetic and Evolutionary Computation Conference(2024)
Abstract
Survival analysis is a critical aspect of modeling time-to-event data in fields such as epidemiology, engineering, and econometrics. Traditional survival methods rely heavily on assumptions and are limited in their application to real-world datasets. To overcome these challenges, we introduce the survival learning classifier system (Survival-LCS) as a more flexible approach. Survival-LCS extends the capabilities of ExSTraCS, a rule-based machine learning algorithm optimized for biomedical applications, to handle survival (time-to-event) data. In addition to accounting for right-censored observations, Survival-LCS handles multiple feature types and missing data, and makes no assumptions about baseline hazard or survival distributions. As proof of concept, we evaluated the Survival-LCS on simulated genetic survival datasets of increasing complexity derived from the GAMETES software. The four genetic models included univariate, epistatic, additive, and heterogeneous models, simulated across a range of censoring proportions, minor allele frequencies, and number of features. The results of this sensitivity analysis demonstrated the ability of Survival-LCS to identify complex patterns of association in survival data. Using the integrated Brier score as the key performance metric, Survival-LCS demonstrated reliable survival time and distribution predictions, potentially useful for clinical applications such as informing self-controls in clinical trials.
MoreTranslated text
AI Read Science
Must-Reading Tree
Example
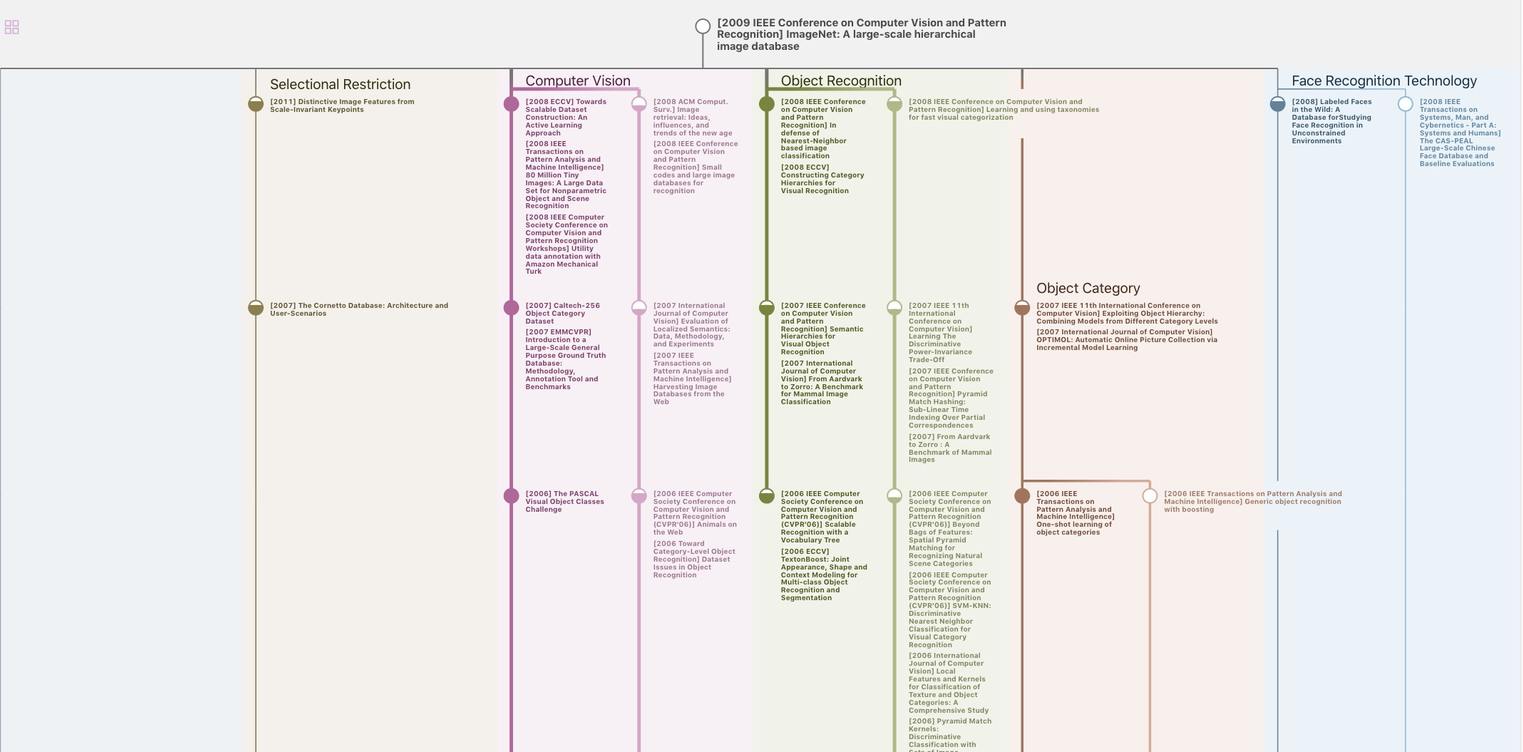
Generate MRT to find the research sequence of this paper
Chat Paper
Summary is being generated by the instructions you defined