Bias-Variance Decomposition: an Effective Tool to Improve Generalization of Genetic Programming-based Evolutionary Feature Construction for Regression
GECCO '24 Proceedings of the Genetic and Evolutionary Computation Conference(2024)
Abstract
Evolutionary feature construction is a technique that has been widely studied in the domain of automated machine learning. A key challenge that needs to be addressed in feature construction is its tendency to overfit the training data. Instead of the traditional approach to control overfitting by reducing model complexity, this paper proposes to control overfitting based on bias-variance decomposition. Specifically, this paper proposes reducing the variance of a model, i.e., reducing the variance of predictions when exposed to data with injected noise, to improve its generalization performance within a multi-objective optimization framework. Experiments conducted on 42 datasets demonstrate that the proposed method effectively controls overfitting and outperforms six model complexity measures for overfitting control. Moreover, further analysis reveals that controlling overfitting adhering to bias-variance decomposition outperforms several plausible variants, highlighting the importance of controlling overfitting based on solid machine learning theory.
MoreTranslated text
AI Read Science
Must-Reading Tree
Example
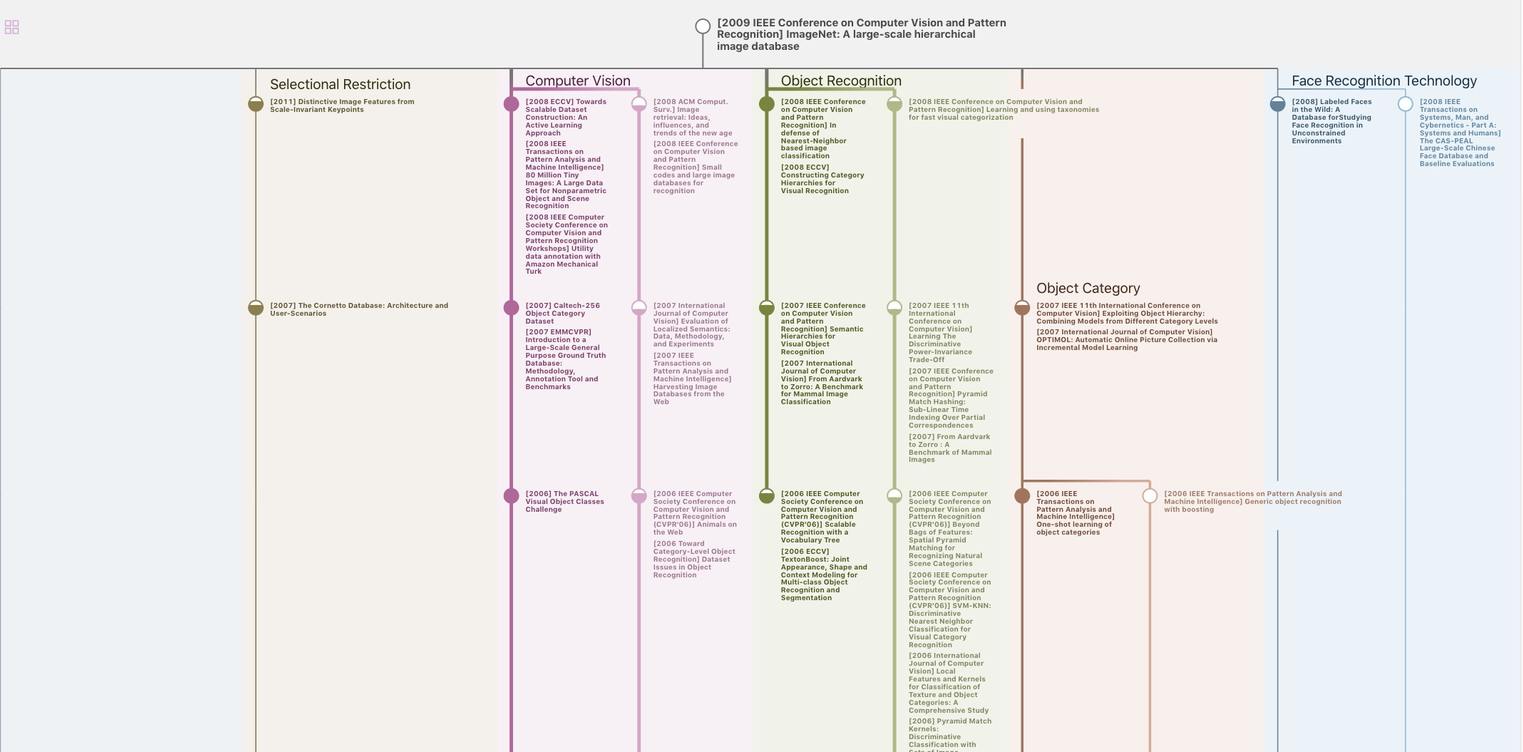
Generate MRT to find the research sequence of this paper
Chat Paper
Summary is being generated by the instructions you defined