Reuse, Don't Retrain: A Recipe for Continued Pretraining of Language Models
arxiv(2024)
摘要
As language models have scaled both their number of parameters and
pretraining dataset sizes, the computational cost for pretraining has become
intractable except for the most well-resourced teams. This increasing cost
makes it ever more important to be able to reuse a model after it has completed
pretraining; allowing for a model's abilities to further improve without
needing to train from scratch. In this work, we detail a set of guidelines that
cover how to design efficacious data distributions and learning rate schedules
for continued pretraining of language models. When applying these findings
within a continued pretraining run on top of a well-trained 15B parameter
model, we show an improvement of 9% in average model accuracy compared to the
baseline of continued training on the pretraining set. The resulting recipe
provides a practical starting point with which to begin developing language
models through reuse rather than retraining.
更多查看译文
AI 理解论文
溯源树
样例
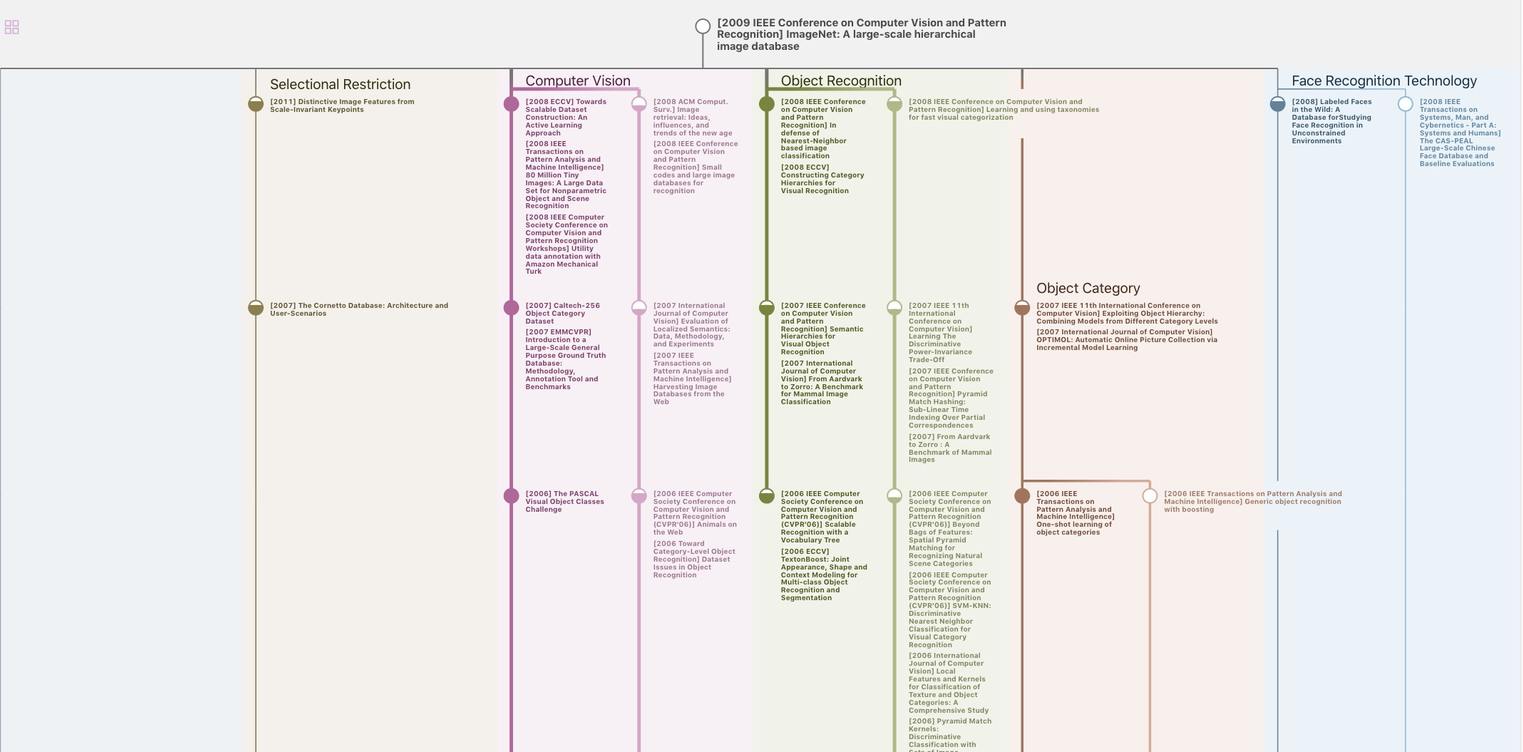
生成溯源树,研究论文发展脉络
Chat Paper
正在生成论文摘要