Physics‐Informed Convolutional Decoder (PICD): A Novel Approach for Direct Inversion of Heterogeneous Subsurface Flow
Geophysical Research Letters(2024)
Abstract
Abstract We propose a physics‐informed convolutional decoder (PICD) framework for inverse modeling of heterogenous groundwater flow. PICD stands out as a direct inversion method, eliminating the need for repeated forward model simulations. The framework combines data‐driven and physics‐driven approaches by integrating monitoring data and domain knowledge into the inversion process. PICD utilizes a convolutional decoder to effectively approximate the spatial distribution of hydraulic heads, while Karhunen–Loève expansion (KLE) is employed to parameterize hydraulic conductivities. During the training process, the stochastic vector in KLE and the parameters of the convolutional decoder are adjusted simultaneously to minimize the data‐mismatch and the physical violation. The final optimized stochastic vectors correspond to the estimation of hydraulic conductivities, and the trained convolutional decoder can predict the evolution and distribution of hydraulic heads. Various scenarios of groundwater flow are examined and results demonstrate the framework's capability to accurately estimate heterogeneous hydraulic conductivities and to deliver satisfactory predictions of hydraulic heads, even with sparse measurements.
MoreTranslated text
Key words
physics‐informed convolutional decoder,inversion method,subsurface flow
AI Read Science
Must-Reading Tree
Example
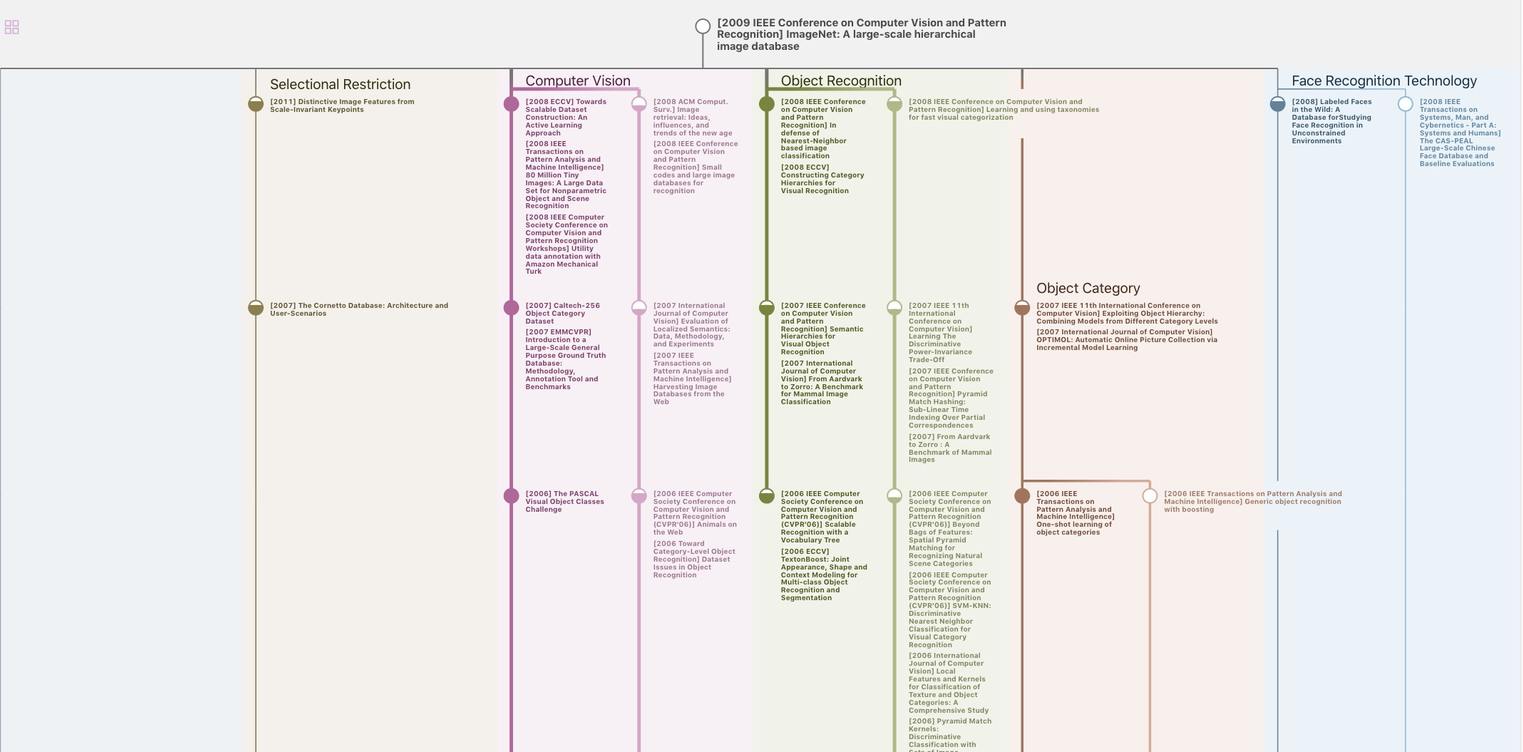
Generate MRT to find the research sequence of this paper
Chat Paper
Summary is being generated by the instructions you defined