A hybrid prediction framework combining deep neural network and modified optimization algorithm for water vapor prediction
Theoretical and Applied Climatology(2024)
摘要
As a global issue, water shortage has attracted much attention from the society. Artificial rain enhancement (ARE) is an effective way to exploit cloud water resources and solve water shortage, but the timing of operation is always a key problem that ARE is facing. The fluctuating properties of water vapor content (WVC) are intricately tied to the choice of operational timing, so accurately predicting the evolution of WVC holds paramount importance when determining the optimal operational timing. However, most of the proposed forecasting methods are limited to simple time series forecasting, and do not pay attention to the complex characteristics of the original data and the shortcomings of a single model prediction. Therefore, the prediction accuracy is difficult to meet the requirements of increasingly refined meteorological services. To tackle this challenge, a new hybrid prediction model, including data reconstruction strategy, benchmark model and improved multi-objective optimization algorithm, is proposed in our research by combining advanced theoretical research of artificial intelligence and data preprocessing ideas. The microwave radiometer WVC observation data at high altitude of Qilian Mountains in China is taken as a case study. By comparing 12 mainstream models, it can be concluded that: The model developed in this study achieves the highest prediction accuracy, and the mean MAPE of the three data sets at 2, 4, 6 and 8 prediction steps is 1.23
更多查看译文
AI 理解论文
溯源树
样例
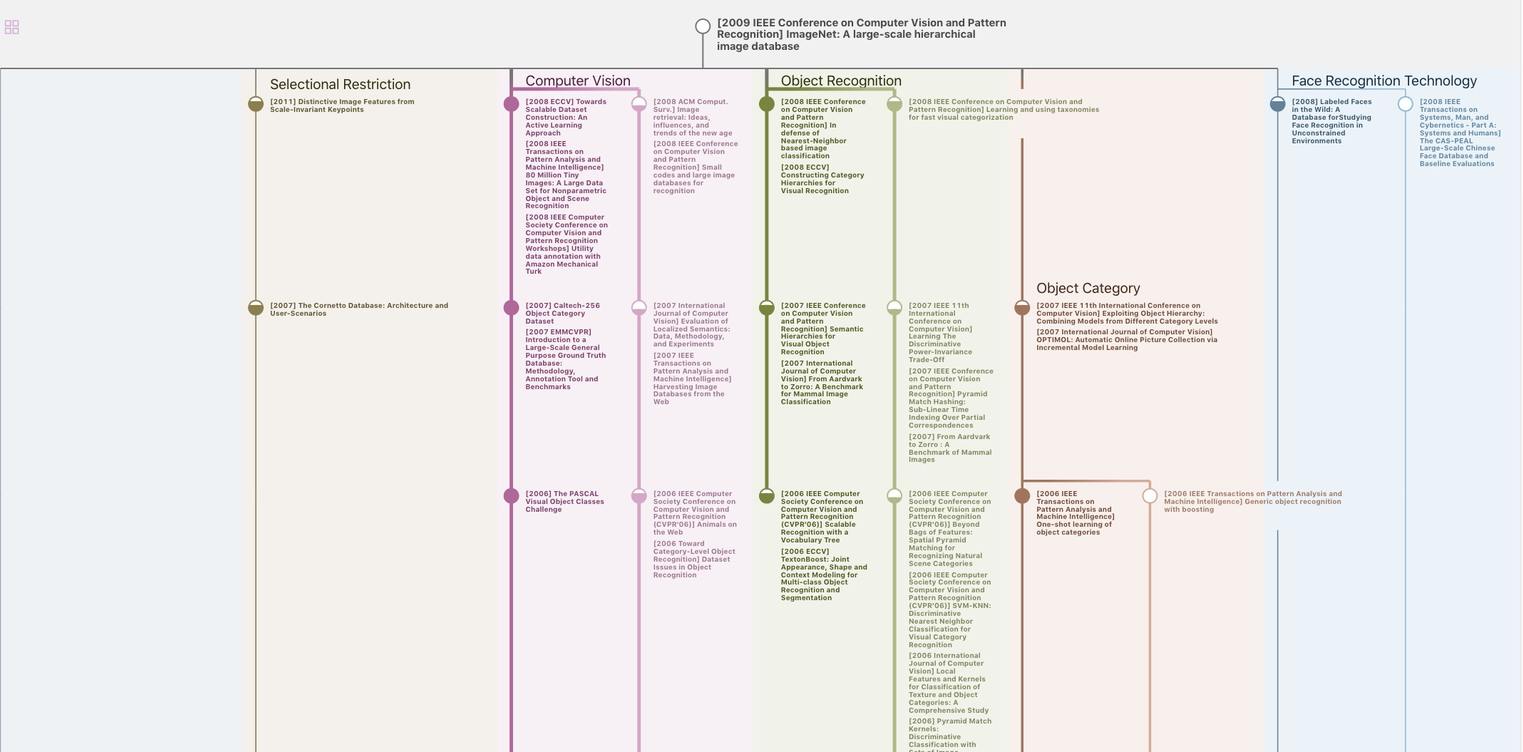
生成溯源树,研究论文发展脉络
Chat Paper
正在生成论文摘要