Long-Term Airport Network Performance Forecasting With Linear Diffusion Graph Networks
IEEE Transactions on Intelligent Transportation Systems(2024)
摘要
Precise forecasting of airport performances, such as landing rates and delays, is essential for the smooth operation of air traffic management systems and for improving the passenger experience. While current efforts predominantly address short-term predictions, the imperative for long-term forecasting is undeniable, particularly for strategic operational planning and resource management. Equally important is the explainability of these forecasts, which is critical for effective decision-making. To meet these needs, our study introduces an innovative approach to airport performance forecasting with the Linear-Diffusion Graph Network (LDGN), an explainable and probabilistic model. The LDGN is intricately structured, comprising stacked temporal linear layers and graph diffusion layers that harness the clarity of linear time series models. This configuration adeptly captures the nuanced interactions between graph-based diffusion processes and the dynamic spread of conditions across airport performances. Departing from conventional point forecasts, the LDGN produces a probabilistic output, prioritizing predictability and a strong capacity for generalization. The model’s pre-training is enhanced with stochastic mask reconstruction, a technique that significantly improves its ability to generalize. Through rigorous testing on real-world datasets, we have validated the LDGN’s superior performance in both long-term and very long-term forecasting. Our results demonstrate not only high accuracy and explainability but also a robust capacity for uncertainty quantification.
更多查看译文
关键词
Airport networks,time series forecasting,graph neural networks
AI 理解论文
溯源树
样例
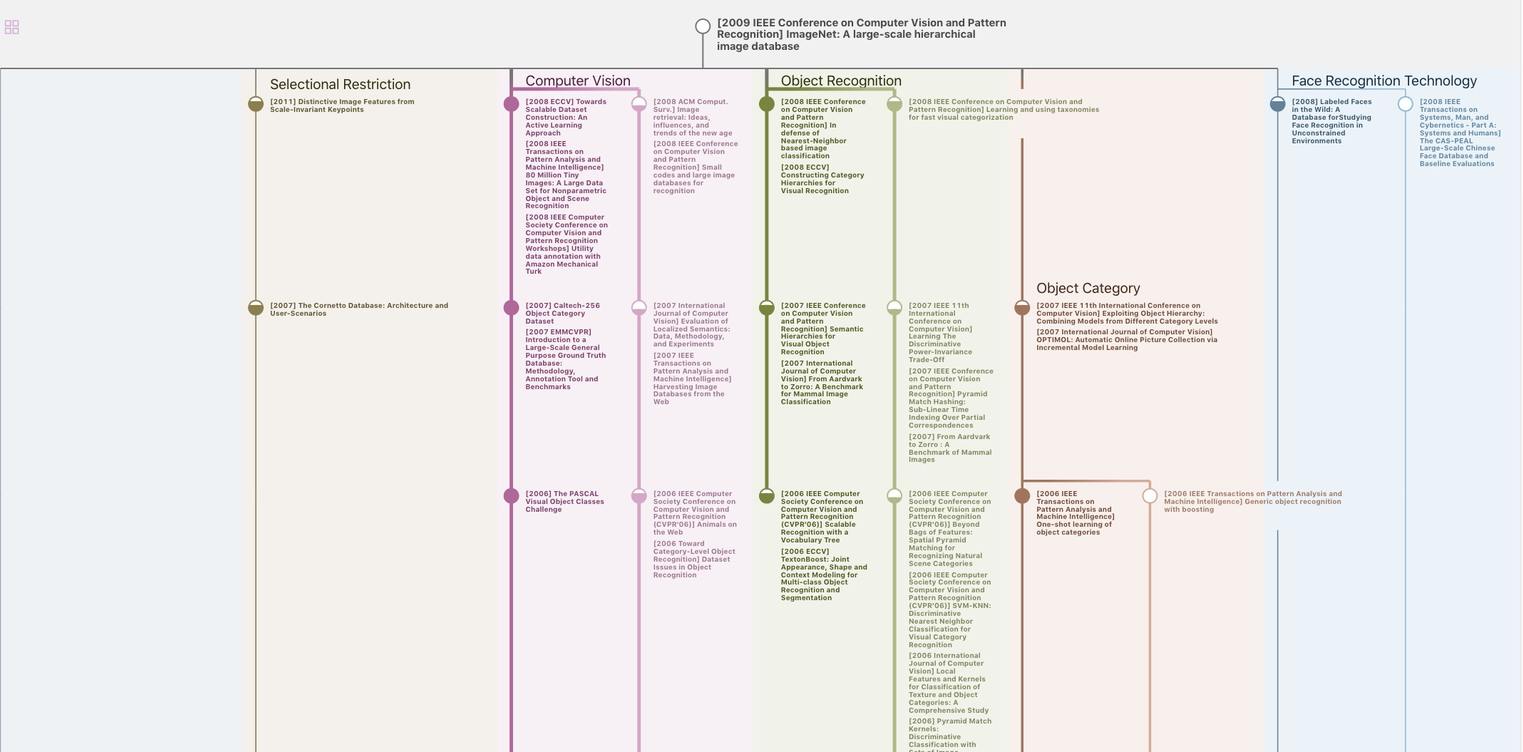
生成溯源树,研究论文发展脉络
Chat Paper
正在生成论文摘要