Intention to treat and per protocol analyses: differences and similarities
Journal of Clinical Epidemiology(2024)
Abstract
Randomized trials can take more explanatory or more pragmatic approaches. Pragmatic studies, conducted closer to real-world conditions, assess treatment effectiveness while considering factors like protocol adherence. In these studies, intention-to-treat (ITT) analysis is fundamental, comparing outcomes regardless of the actual treatment received. Explanatory trials, conducted closer to optimal conditions, evaluate treatment efficacy, commonly with a per protocol (PP) analysis, which includes only outcomes from adherent participants.ITT and PP are strategies used in the conception, design, conduct (protocol execution), analysis, and interpretation of trials. Each serves distinct objectives. While both can be valid, when bias is controlled, and complementary, each has its own limitations. By excluding non-adherent participants, PP analyses can lose the benefits of randomization, resulting in group differences in factors (influencing adherence and outcomes) that were present at baseline. Additionally, clinical and social factors affecting adherence can also operate during follow-up, i.e., after randomization. Therefore, incomplete adherence may introduce postrandomization confounding. Conversely, ITT analysis, including all participants regardless of adherence, may dilute treatment effects. Moreover, varying adherence levels could limit the applicability of ITT findings in settings with diverse adherence patterns. Both ITT and PP analyses can be affected by selection bias due to differential losses and nonresponse (i.e., missing data) during follow-up.Combining high quality and comprehensive data with advanced statistical methods, known as g-methods, like inverse probability weighting, may help address postrandomization confounding in PP analysis, as well as selection bias in both ITT and PP analyses.
MoreTranslated text
AI Read Science
Must-Reading Tree
Example
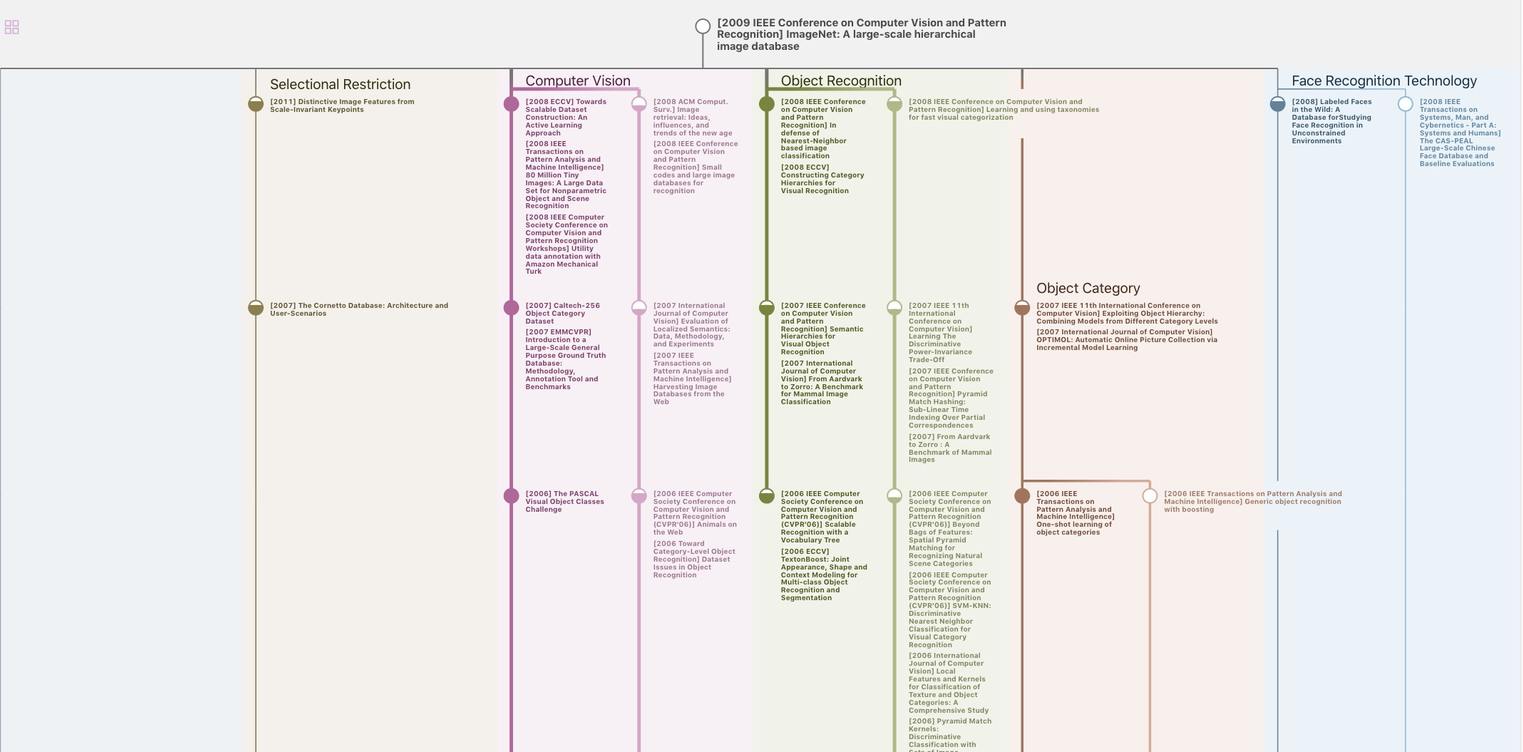
Generate MRT to find the research sequence of this paper
Chat Paper
Summary is being generated by the instructions you defined