Efficient and Scalable Prediction of Stochastic Reaction–diffusion Processes Using Graph Neural Networks
Mathematical Biosciences(2024)
摘要
The dynamics of locally interacting particles that are distributed in space give rise to a multitude of complex behaviours. However the simulation of reaction–diffusion processes which model such systems is highly computationally expensive, the cost increasing rapidly with the size of space. Here, we devise a graph neural network based approach that uses cheap Monte Carlo simulations of reaction–diffusion processes in a small space to cast predictions of the dynamics of the same processes in a much larger and complex space, including spaces modelled by networks with heterogeneous topology. By applying the method to two biological examples, we show that it leads to accurate results in a small fraction of the computation time of standard stochastic simulation methods. The scalability and accuracy of the method suggest it is a promising approach for studying reaction–diffusion processes in complex spatial domains such as those modelling biochemical reactions, population evolution and epidemic spreading.
更多查看译文
关键词
Reaction–diffusion,Master equation,Neural networks
AI 理解论文
溯源树
样例
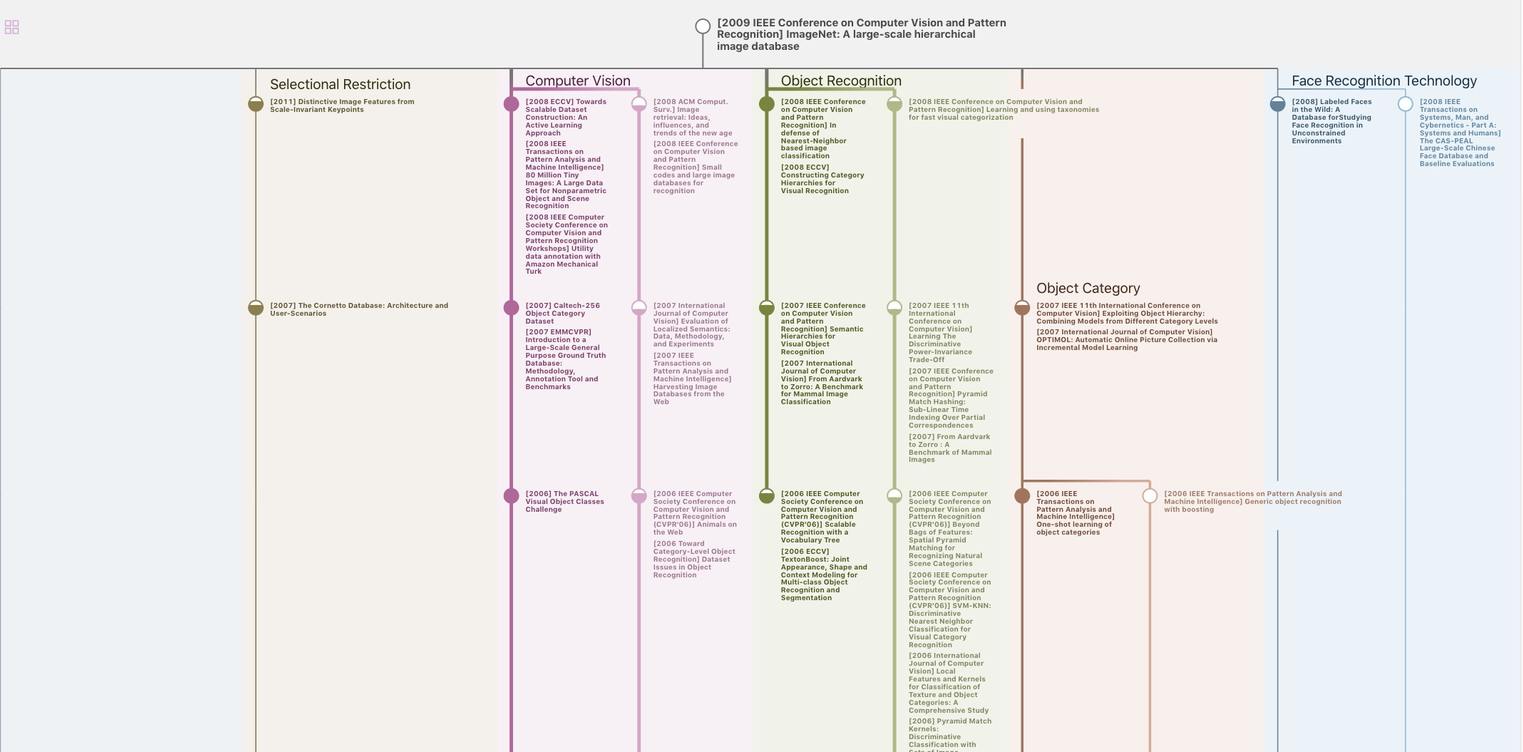
生成溯源树,研究论文发展脉络
Chat Paper
正在生成论文摘要