KidSat: satellite imagery to map childhood poverty dataset and benchmark
arxiv(2024)
Abstract
Satellite imagery has emerged as an important tool to analyse demographic,
health, and development indicators. While various deep learning models have
been built for these tasks, each is specific to a particular problem, with few
standard benchmarks available. We propose a new dataset pairing satellite
imagery and high-quality survey data on child poverty to benchmark satellite
feature representations. Our dataset consists of 33,608 images, each 10 km
× 10 km, from 19 countries in Eastern and Southern Africa in the time
period 1997-2022. As defined by UNICEF, multidimensional child poverty covers
six dimensions and it can be calculated from the face-to-face Demographic and
Health Surveys (DHS) Program . As part of the benchmark, we test spatial as
well as temporal generalization, by testing on unseen locations, and on data
after the training years. Using our dataset we benchmark multiple models, from
low-level satellite imagery models such as MOSAIKS , to deep learning
foundation models, which include both generic vision models such as
Self-Distillation with no Labels (DINOv2) models and specific satellite imagery
models such as SatMAE. We provide open source code for building the satellite
dataset, obtaining ground truth data from DHS and running various models
assessed in our work.
MoreTranslated text
AI Read Science
Must-Reading Tree
Example
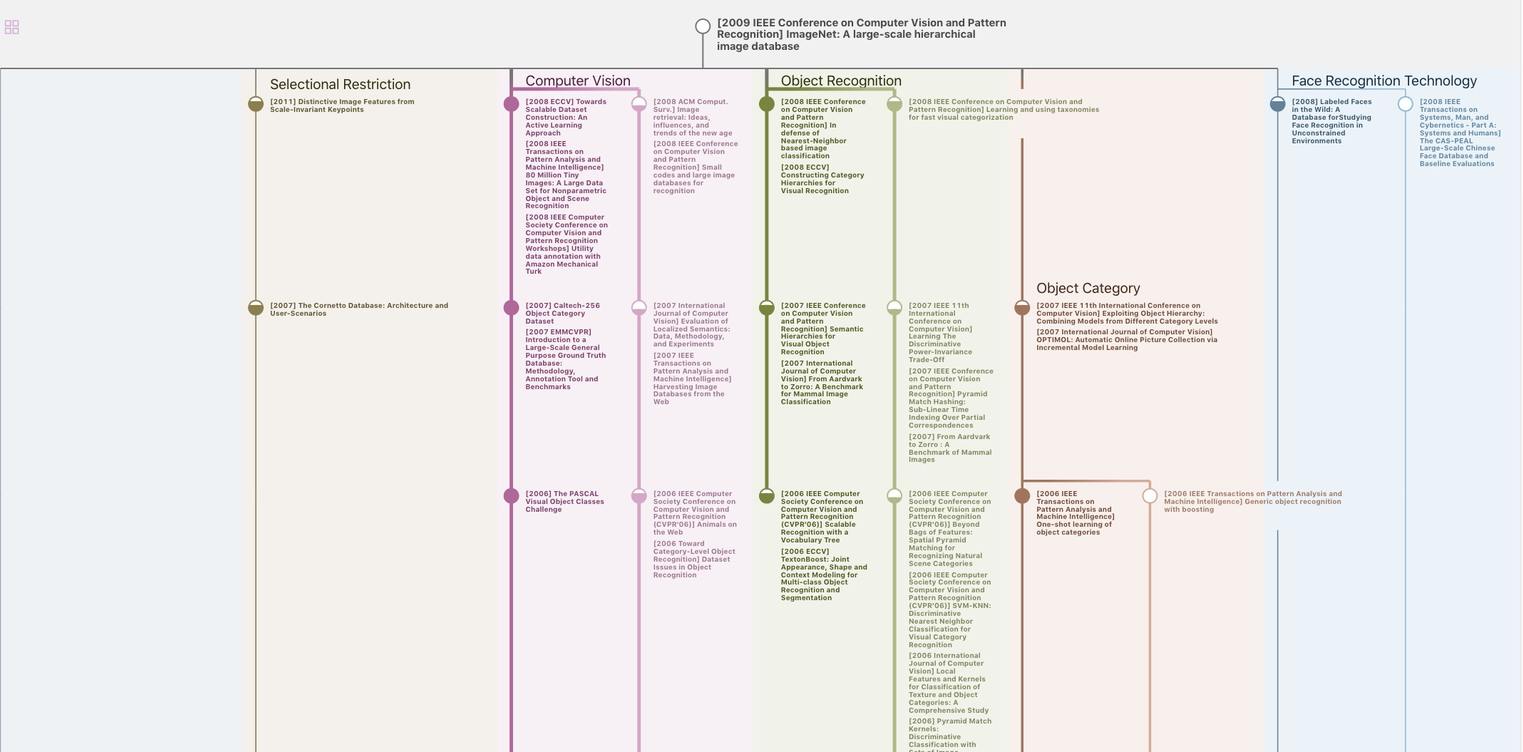
Generate MRT to find the research sequence of this paper
Chat Paper
Summary is being generated by the instructions you defined