Late Breaking Results: Fortifying Neural Networks: Safeguarding Against Adversarial Attacks with Stochastic Computing
arxiv(2024)
摘要
In neural network (NN) security, safeguarding model integrity and resilience against adversarial attacks has become paramount. This study investigates the application of stochastic computing (SC) as a novel mechanism to fortify NN models. The primary objective is to assess the efficacy of SC to mitigate the deleterious impact of attacks on NN results. Through a series of rigorous experiments and evaluations, we explore the resilience of NNs employing SC when subjected to adversarial attacks. Our findings reveal that SC introduces a robust layer of defense, significantly reducing the susceptibility of networks to attack-induced alterations in their outcomes. This research contributes novel insights into the development of more secure and reliable NN systems, essential for applications in sensitive domains where data integrity is of utmost concern.
更多查看译文
AI 理解论文
溯源树
样例
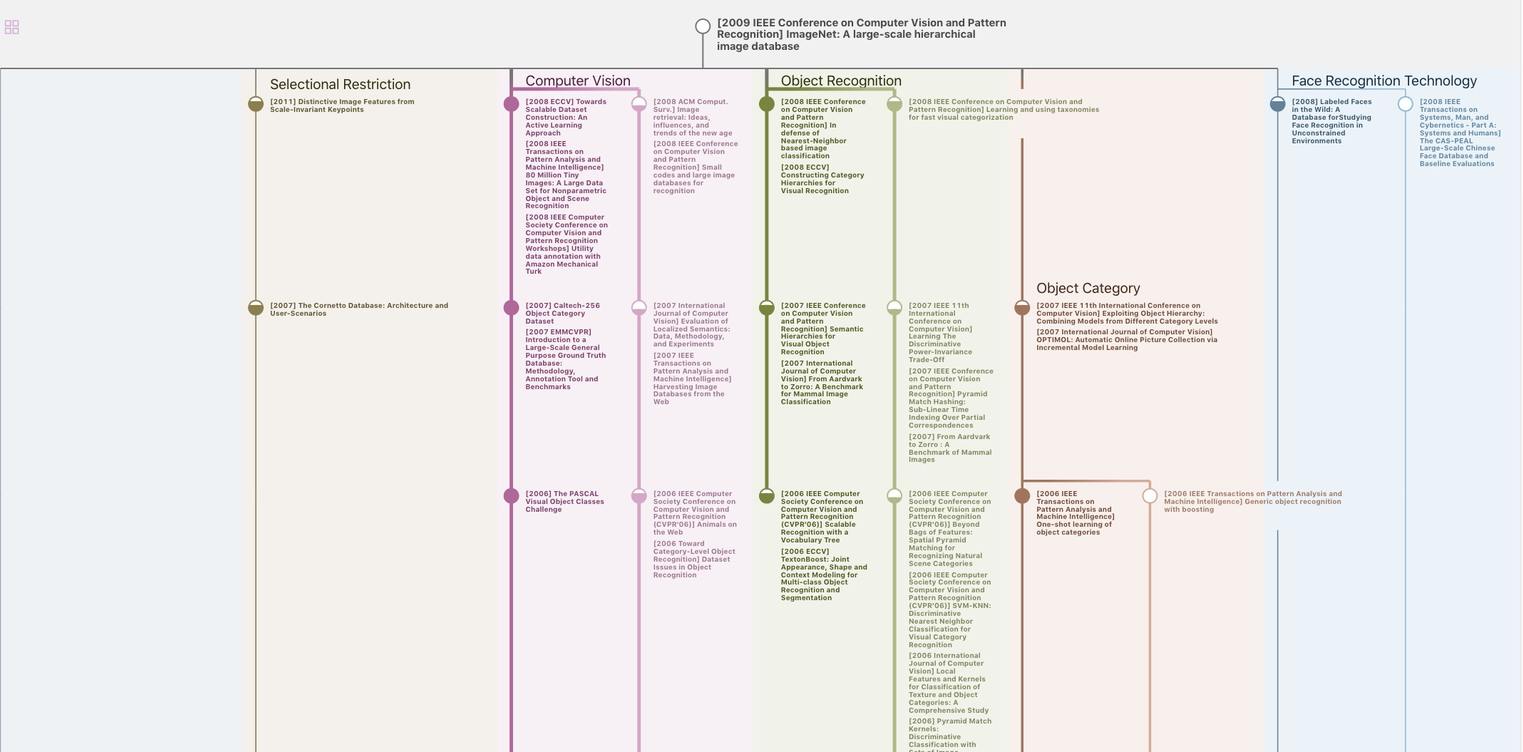
生成溯源树,研究论文发展脉络
Chat Paper
正在生成论文摘要