Heterogeneous Hypergraph Embedding for Recommendation Systems
arxiv(2024)
Abstract
Recent advancements in recommender systems have focused on integrating
knowledge graphs (KGs) to leverage their auxiliary information. The core idea
of KG-enhanced recommenders is to incorporate rich semantic information for
more accurate recommendations. However, two main challenges persist: i)
Neglecting complex higher-order interactions in the KG-based user-item network,
potentially leading to sub-optimal recommendations, and ii) Dealing with the
heterogeneous modalities of input sources, such as user-item bipartite graphs
and KGs, which may introduce noise and inaccuracies. To address these issues,
we present a novel Knowledge-enhanced Heterogeneous Hypergraph Recommender
System (KHGRec). KHGRec captures group-wise characteristics of both the
interaction network and the KG, modeling complex connections in the KG. Using a
collaborative knowledge heterogeneous hypergraph (CKHG), it employs two
hypergraph encoders to model group-wise interdependencies and ensure
explainability. Additionally, it fuses signals from the input graphs with
cross-view self-supervised learning and attention mechanisms. Extensive
experiments on four real-world datasets show our model's superiority over
various state-of-the-art baselines, with an average 5.18% relative
improvement. Additional tests on noise resilience, missing data, and cold-start
problems demonstrate the robustness of our KHGRec framework. Our model and
evaluation datasets are publicly available at
.
MoreTranslated text
AI Read Science
Must-Reading Tree
Example
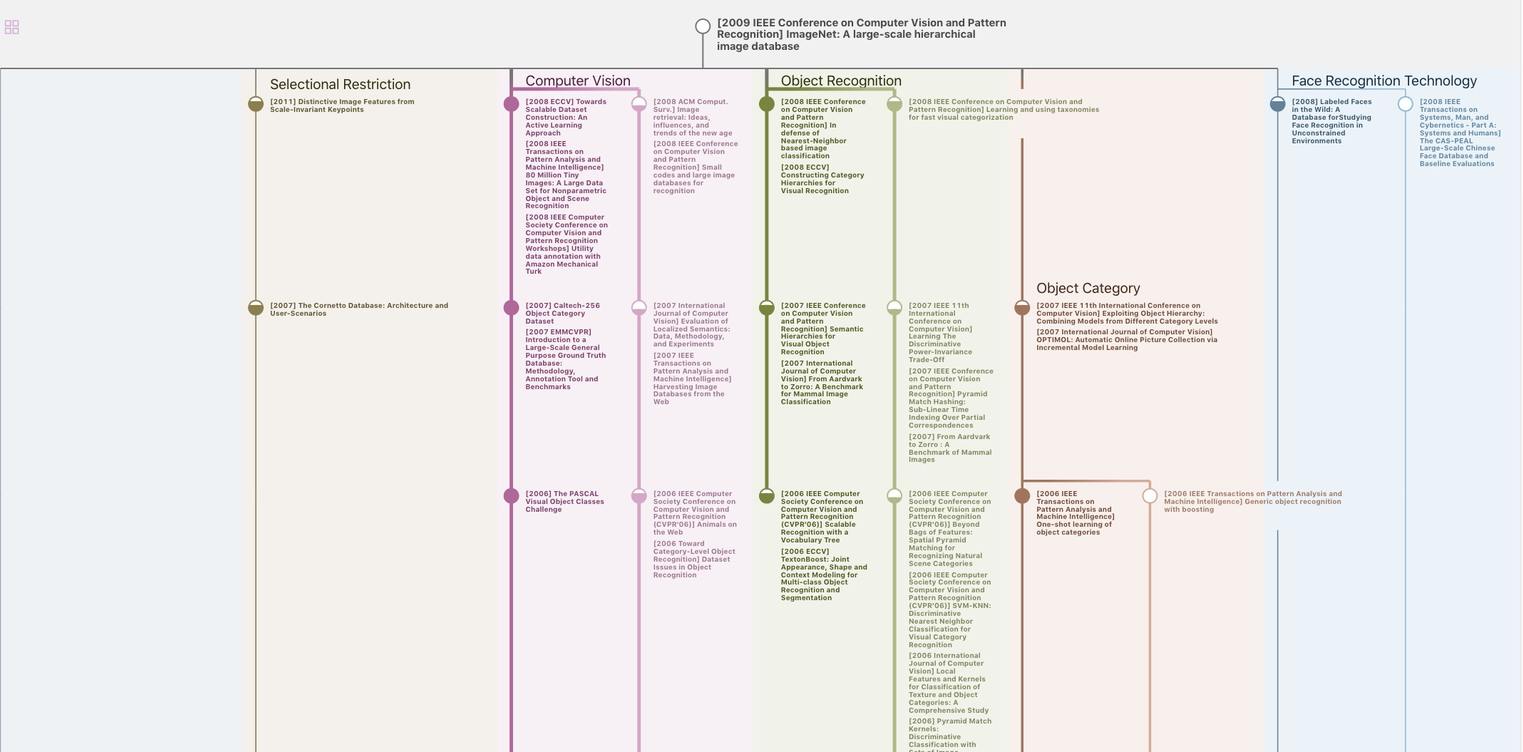
Generate MRT to find the research sequence of this paper
Chat Paper
Summary is being generated by the instructions you defined