On the performance of sequential Bayesian update for database of diverse tsunami scenarios
arxiv(2024)
Abstract
Although the sequential tsunami scenario detection framework was validated in
our previous work, several tasks remain to be resolved from a practical point
of view. This study aims to evaluate the performance of the previous tsunami
scenario detection framework using a diverse database consisting of complex
fault rupture patterns with heterogeneous slip distributions. Specifically, we
compare the effectiveness of scenario superposition to that of the previous
most likely scenario detection method. Additionally, how the length of the
observation time window influences the accuracy of both methods is analyzed. We
utilize an existing database comprising 1771 tsunami scenarios targeting the
city Westport (WA, U.S.), which includes synthetic wave height records and
inundation distributions as the result of fault rupture in the Cascadia
subduction zone. The heterogeneous patterns of slips used in the database
increase the diversity of the scenarios and thus make it a proper database for
evaluating the performance of scenario superposition. To assess the
performance, we consider various observation time windows shorter than 15
minutes and divide the database into five testing and learning sets. The
evaluation accuracy of the maximum offshore wave, inundation depth, and its
distribution is analyzed to examine the advantages of the scenario
superposition method over the previous method. We introduce the dynamic time
warping (DTW) method as an additional benchmark and compare its results to that
of the Bayesian scenario detection method.
MoreTranslated text
AI Read Science
Must-Reading Tree
Example
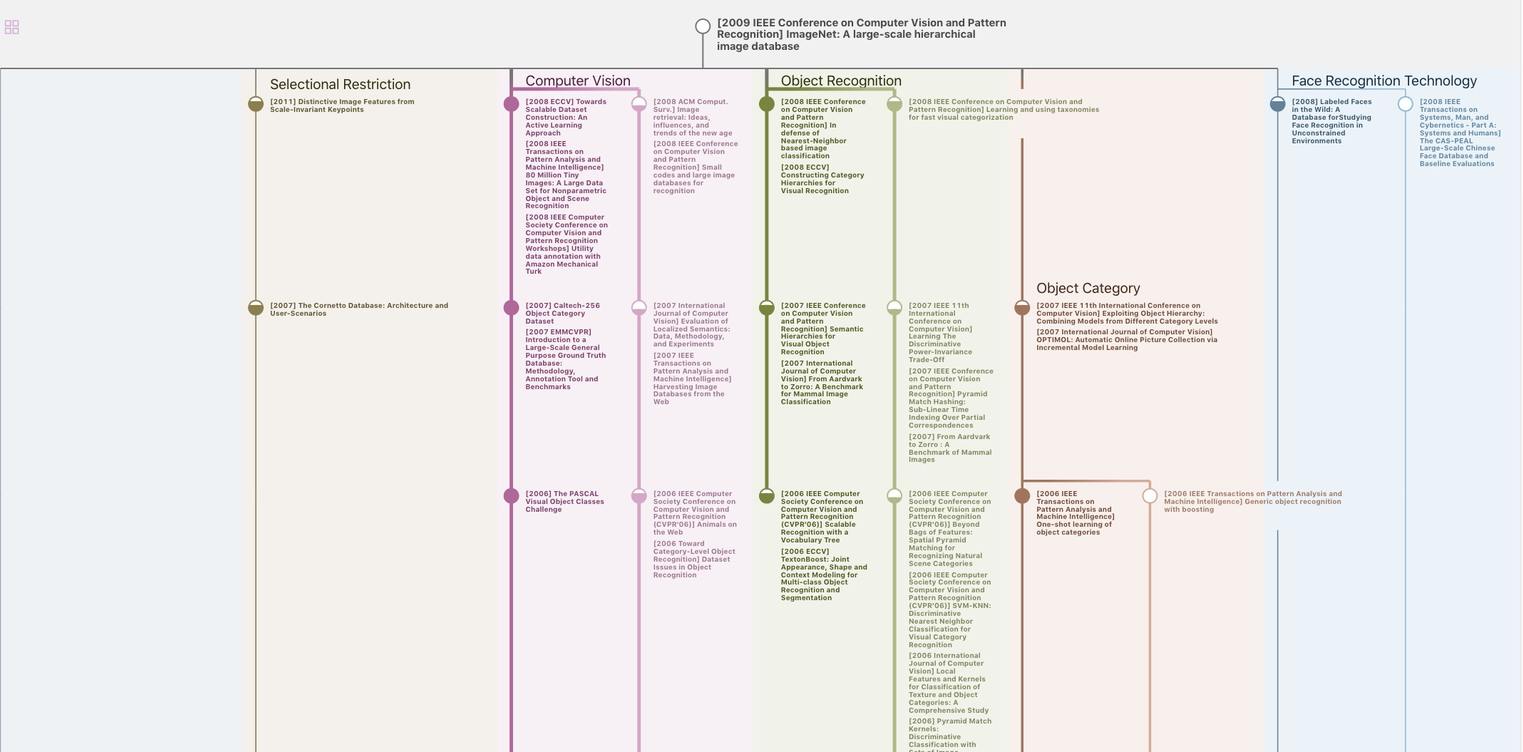
Generate MRT to find the research sequence of this paper
Chat Paper
Summary is being generated by the instructions you defined