Machine Learning-Based Design of Superhard High-Entropy Nitride Coatings.
ACS applied materials & interfaces(2024)
摘要
Limited by the inefficiency of the conventional trial-and-error method and the boundless compositional design space of high-entropy alloys (HEAs), accelerating the discovery of superior-performing high-entropy nitride (HEN) coatings remains a formidable challenge. Herein, the superhard HEN coatings were designed and prepared using the rapidly developing data-driven model machine learning (ML). A database containing hardness and different features of HEN coatings was established and categorized into four subsets covering the information on composition, composition-physical descriptors, composition-technique parameters, and composition-physical descriptors-technique parameters. Feature engineering was employed to reduce dimensionality and interpret the impact of features on the evolution of hardness. Both root mean squared error (RMSE) and decision coefficient (R2) were applied to assess the predictive accuracy of ML models with different subsets, proportions of test set, and algorithms. The model with best predicted performance was used to explore superhard HEN coatings in a predefined virtual space. Among the generated 5-/6-/7-/8-component (excluding N) systems, the coating possessing highest hardness was individually selected for further preparation. Four newly prepared coatings achieved the superhard level with an average prediction error of 7.83%. The morphology, chemical composition, structure, and hardness of the newly prepared coatings were discussed. The nanocrystal-amorphous nanocomposite structure of the novel AlCrNbSiTiN coating with the highest hardness of 45.77 GPa was revealed. The results demonstrated that ML can effectively guide the design and composition optimization of superb-performance protective HEN coatings.
更多查看译文
AI 理解论文
溯源树
样例
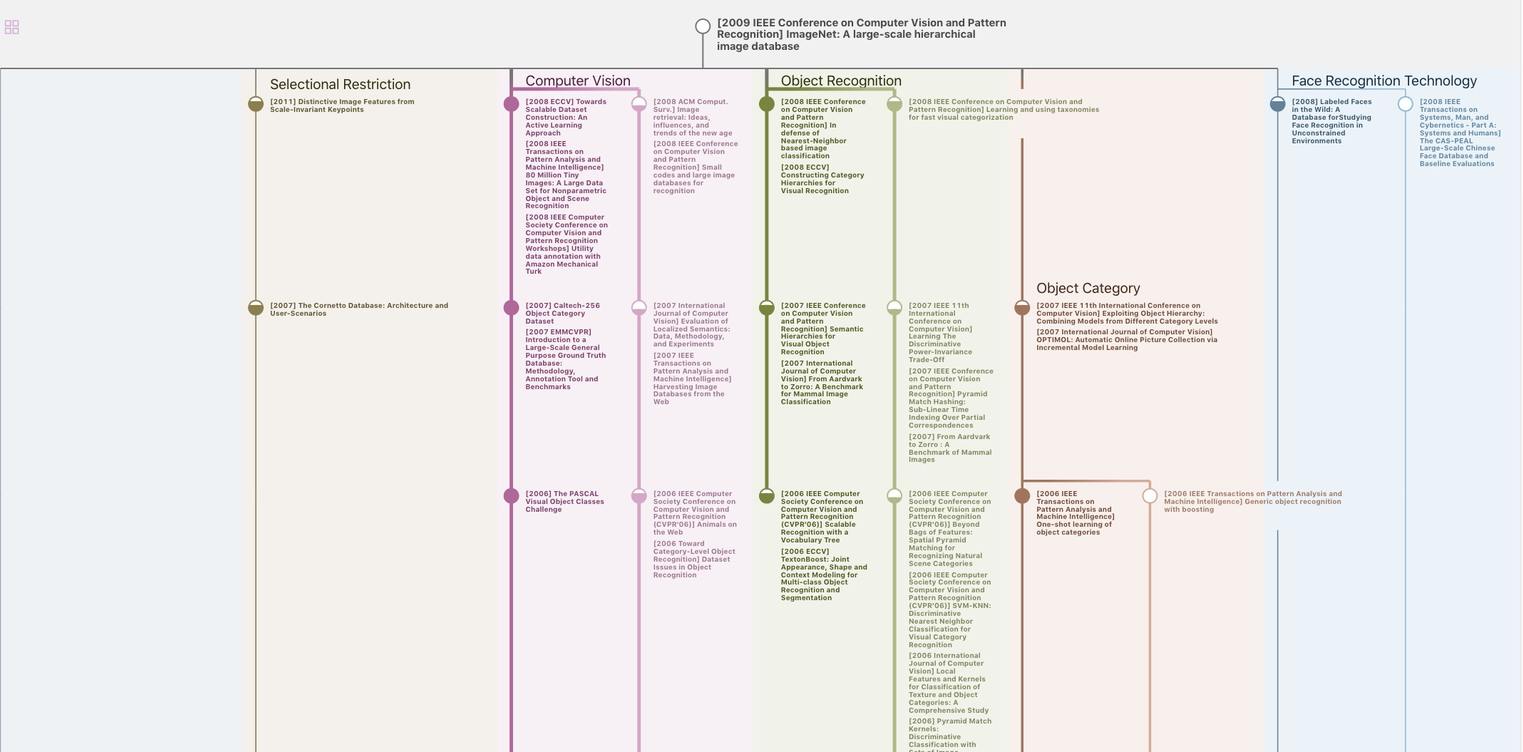
生成溯源树,研究论文发展脉络
Chat Paper
正在生成论文摘要