Applications of XGBoost in Water Resources Engineering
Environmental Modelling & Software(2024)
Abstract
Applications of Machine Learning methods make a paradigm shift in the domain of water resources engineering. This study not only presents the story of emerging eXtreme Gradient Boosting (XGBoost) but also encompasses a thorough review XGBoost utilization to problems in hydrology, hydraulics, and hydroclimatology. According to the literature, XGBoost was employed for hydrological modelling, forecasting water quantity and quality, and groundwater management. In the context of hydraulic modelling, the review assessed XGBoost performances for estimating scouring and sediment transport, reservoir modelling, open channel and pressurized flow predictions, and hydraulic structure. Additionally, the role of XGBoost in forecasting hydroclimatic variables, drought assessment, and statistical downscaling was discussed. The review revealed that in 74% of papers, XGBoost or a hybrid XGBoost-based model resulted in the best results among other ML models in diverse applications. Finally, the study presents some suggestions for future studies in the context of XGBoost applications.
MoreTranslated text
Key words
eXtreme Gradient Boosting,XGBoost,Machine learning,Water resources
AI Read Science
Must-Reading Tree
Example
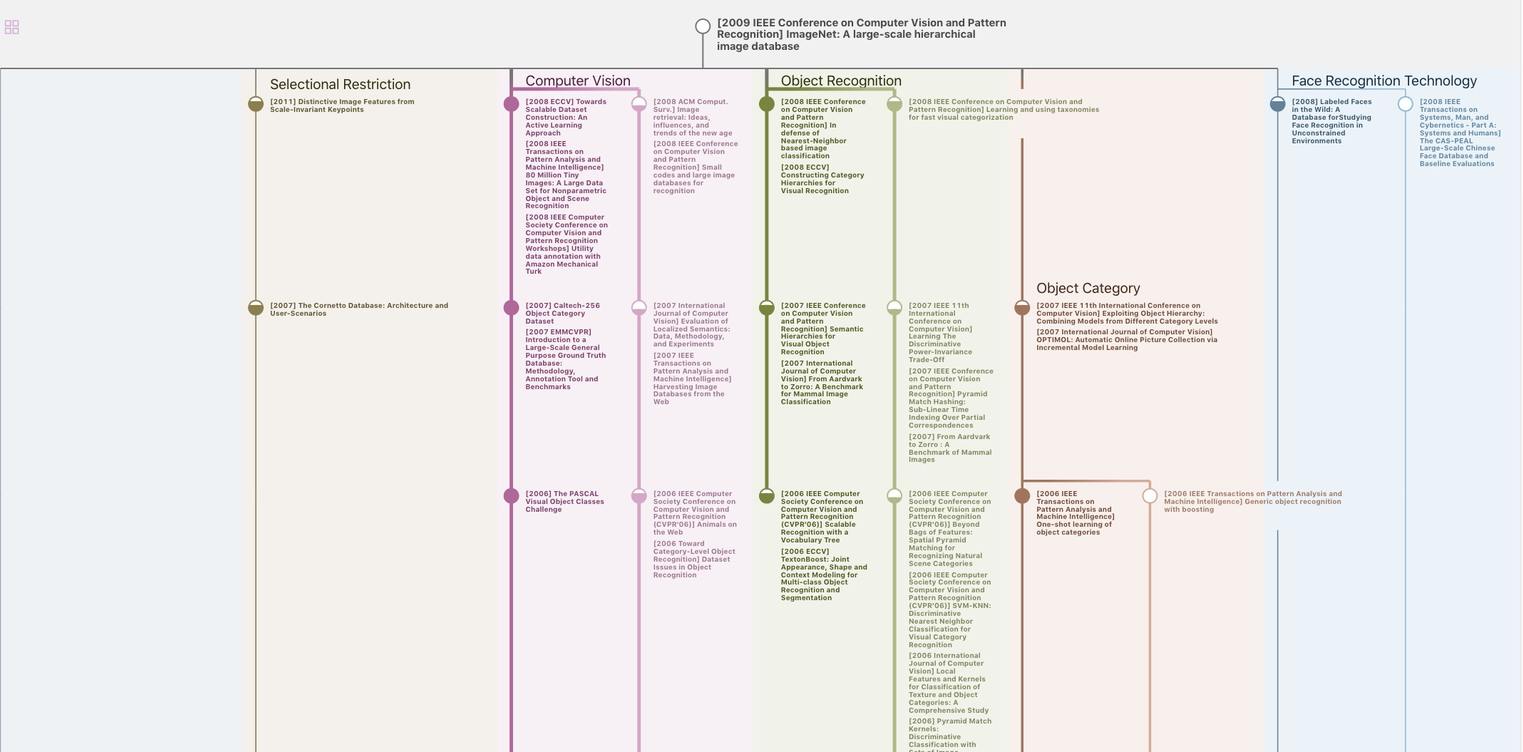
Generate MRT to find the research sequence of this paper
Chat Paper
Summary is being generated by the instructions you defined