ADMM Based Fourier Phase Retrieval with Untrained Generative Prior
Journal of computational and applied mathematics(2024)
Abstract
Fourier phase retrieval (FPR) is an inverse problem that aims to recover a signal from its Fourier magnitude measurement. It is an ill-posed problem, especially when the number of measurements is limited. In this paper, we introduce an untrained generative network that learns image priors without training on a large dataset to alleviate the ill-posedness. Based on the alternating direction method of multipliers (ADMM), an algorithm called Net-ADM that utilizes the untrained generative network is proposed to solve the FPR problem. Firstly, the dimension of the variable is increased and the objective function is modified to facilitate calculation. Then, an untrained generative network is embedded in the iterative process of ADMM to project an estimated signal into the generative space that captures some image characteristics. We theoretically prove the existence of a solution to the established model and analyze the convergence of the algorithm. Furthermore, one projection of Net-ADM makes the objective function non-increasing, while the other can bring the estimation closer to the optimal solution (or at least maintain the same distance) under certain conditions. Numerical experiments show that the proposed algorithm achieves superior accuracy and robustness compared to prior works, especially when the number of measurements is limited.
MoreTranslated text
Key words
Fourier phase retrieval,Untrained generative prior,Alternating direction method of multipliers
AI Read Science
Must-Reading Tree
Example
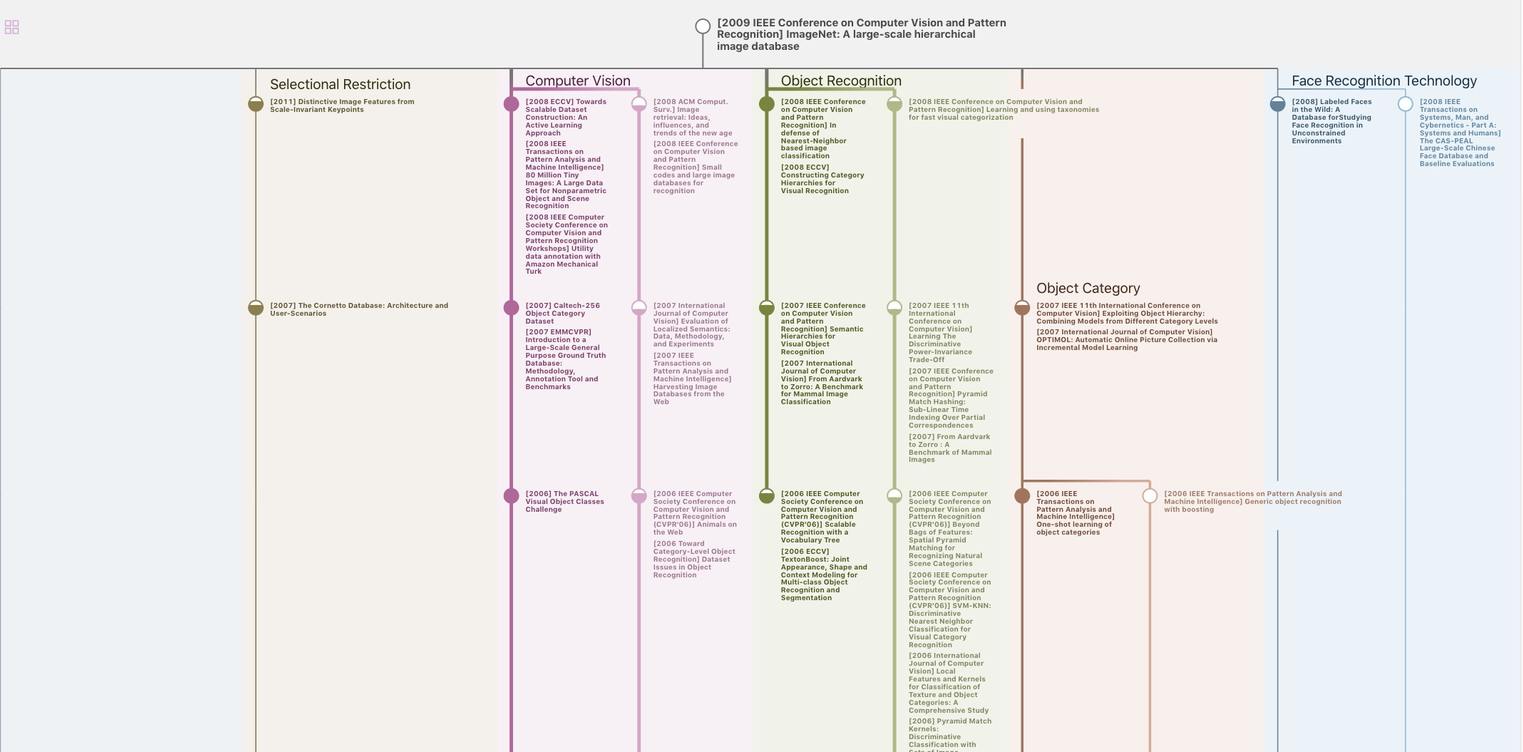
Generate MRT to find the research sequence of this paper
Chat Paper
Summary is being generated by the instructions you defined