Representative Kernels-based CNN for Faster Transmission in Federated Learning
IEEE Transactions on Mobile Computing(2024)
摘要
Federated Learning (FL) has attracted many attentions because of its ability to ensure data privacy and security. In FL, due to the contradiction between limited bandwidth and huge transmission parameters, it has been an ongoing challenge to reduce the model parameters that need to be transmitted to the server in the clients for fast transmission. Existing works that attempt to reduce the amount of transmitted parameters have limitations: 1) the reduced number of parameters is not significant; 2) the performance of the global model is limited. In this paper, we propose a novel method called
Fed-KGF
that significantly reduces the amount of model parameters that need to be transferred while improving the performance of the global model in FL. Since convolution kernels in a Convolution Neural Network (CNN) account for most parameters, our goal is to reduce the parameter transmission between the clients and the server by reducing the number of convolution kernels. Specifically, we construct an incomplete model with a few representative kernels, referred to the convolution kernels, that are solely updated during training. We propose Kernel Generation Function (KGF) to generate convolution kernels to render the incomplete model to be a complete one, and discard those generated kernels after training local models. The parameters that need to be transmitted only reside in the representative kernels, which are significantly reduced. Furthermore, there is a client-drift in the traditional FL because it adopts the averaging method, which hurts the global model performance. We innovatively select one or few modules (a module indicates a convolution function + several non-convolution functions) from all client models in a permutation way, and only aggregate the uploaded modules rather than averaging them in server to reduce client-drift, thereby improving the performance of the global model and further reducing the transmitted parameters. Experimental results on both non-Independent and Identically Distributed (non-IID) and IID scenarios for image classification and object detection tasks demonstrate that our
Fed-KGF
outperforms the SOTA methods.
Fed-KGF
achieves approximately 11% higher classification accuracy and roughly 33% fewer parameters than the recent FedCAMS model on CIFAR-10, and gains approximately 3.64% higher detection precision and around 37% fewer parameters than the SOTA SmartIdx model on COCO2017 datasets.
更多查看译文
关键词
Federated learning,Convolution neural network,Representative kernels,Kernel generation function,Parameter reduction,Module selection
AI 理解论文
溯源树
样例
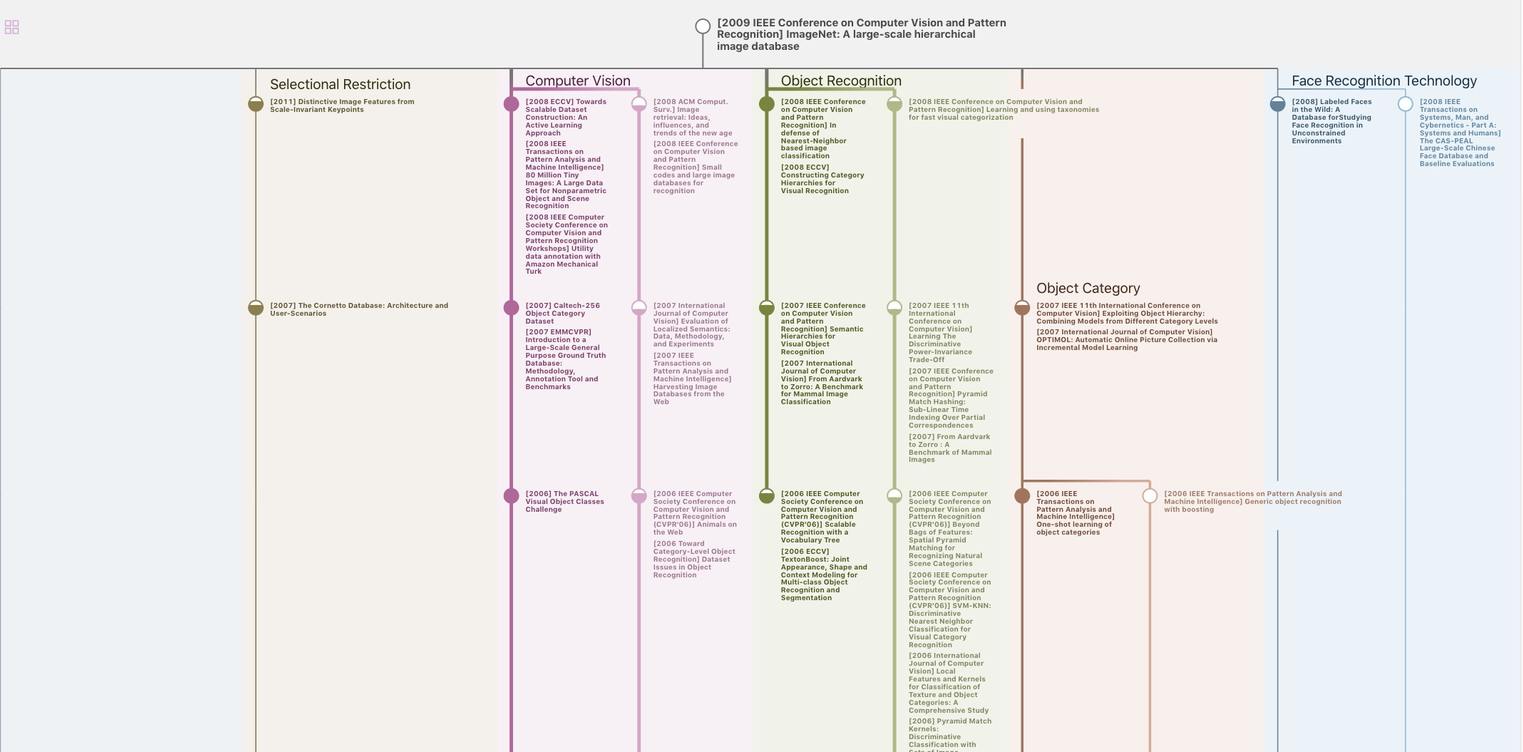
生成溯源树,研究论文发展脉络
Chat Paper
正在生成论文摘要