Ensemble based fully convolutional transformer network for time series classification
Applied Intelligence(2024)
摘要
Currently, multivariate time series classification is widely used in various fields, including industrial process control, action recognition, and health monitoring. Due to their sequential modeling capabilities, Transformer networks with self-attention mechanisms have been successfully applied to multivariate time series classification tasks. However, the original transformer network can only calculate the relative dependence between two adjacent time steps, which makes it difficult to find local continuous dependence from scattered time steps. To address this issue, we propose an ensemble model called Ensemble Based Fully Convolutional Transformer Network (E-FCTN) that combines a gated transformer network (GTN) and a fully convolutional neural network (FCN). In the E-FCTN ensemble model, the GTN calculates the attention matrix between channels and time steps in a multivariate time series. Meanwhile, the FCN is also applied to extract local features to construct the basic belief assignment. Using the Proportional Conflict Redistribution rule no. 5 (PCR5) in the Dezert-Smarandache theory (DSmT), the output of the FCN and GTN are fused at the decision level, and the final decision is made according to the maximum plausibility. The information extracted by the FCN compensates for the deficiency of the GTN in local feature extraction. Finally, the proposed method is validated on eight publicly available datasets to demonstrate its effectiveness.
更多查看译文
关键词
Time series classification,Transformer,GTN,FCN,Decision-level fusion
AI 理解论文
溯源树
样例
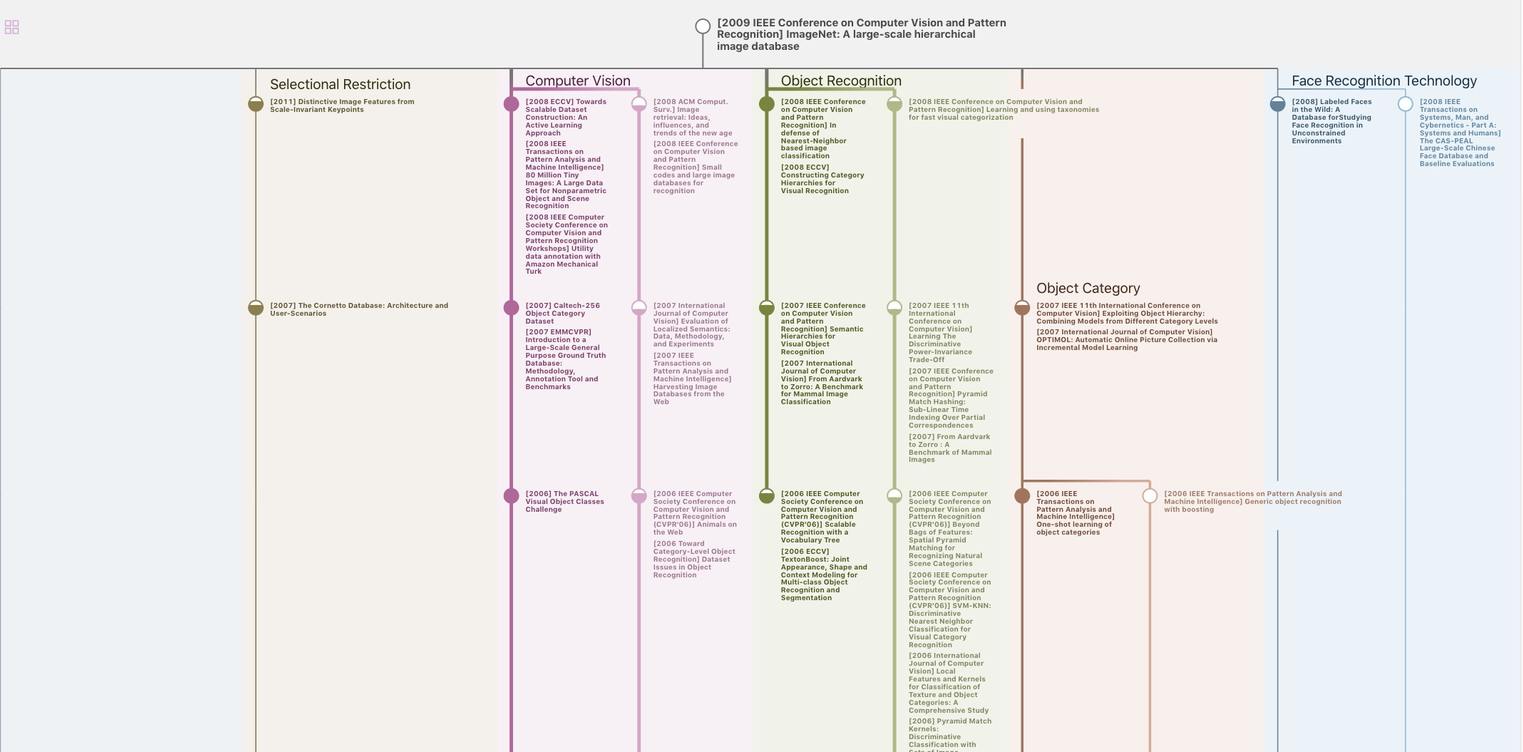
生成溯源树,研究论文发展脉络
Chat Paper
正在生成论文摘要