Efficient Statistical Linear Precoding for Downlink Massive MIMO Systems
IEEE Transactions on Wireless Communications(2024)
Abstract
In this paper, we study low-complexity linear precoding for downlink massive multiple-input multiple-output (MIMO) systems, exploiting a statistical method. In sharp contrast to traditional linear precoding algorithms, our proposed efficient randomized iterative precoding algorithm (ERIPA) not only avoids costly matrix inversion but also considers the complexity reduction of matrix multiplication involved, thus enabling more efficient linear precoding. Additionally, ERIPA is demonstrated to have both exponentially fast and global convergence, making it adaptable to various practical scenarios of massive MIMO. We also investigate the convergence phenomenon of ERIPA in relation to the selection of the sampling distribution during random iterations. After that, the concept of conditional sampling is introduced to ERIPA such that significant system potential can be beneficially exploited in terms of both precoding performance and computational complexity. Finally, simulation results regarding the downlink massive MIMO are presented to confirm the superiorities of the proposed ERIPA.
MoreTranslated text
Key words
Massive MIMO,linear precoding,low complexity,global convergence,iterative methods,convergence analysis and enhancement
AI Read Science
Must-Reading Tree
Example
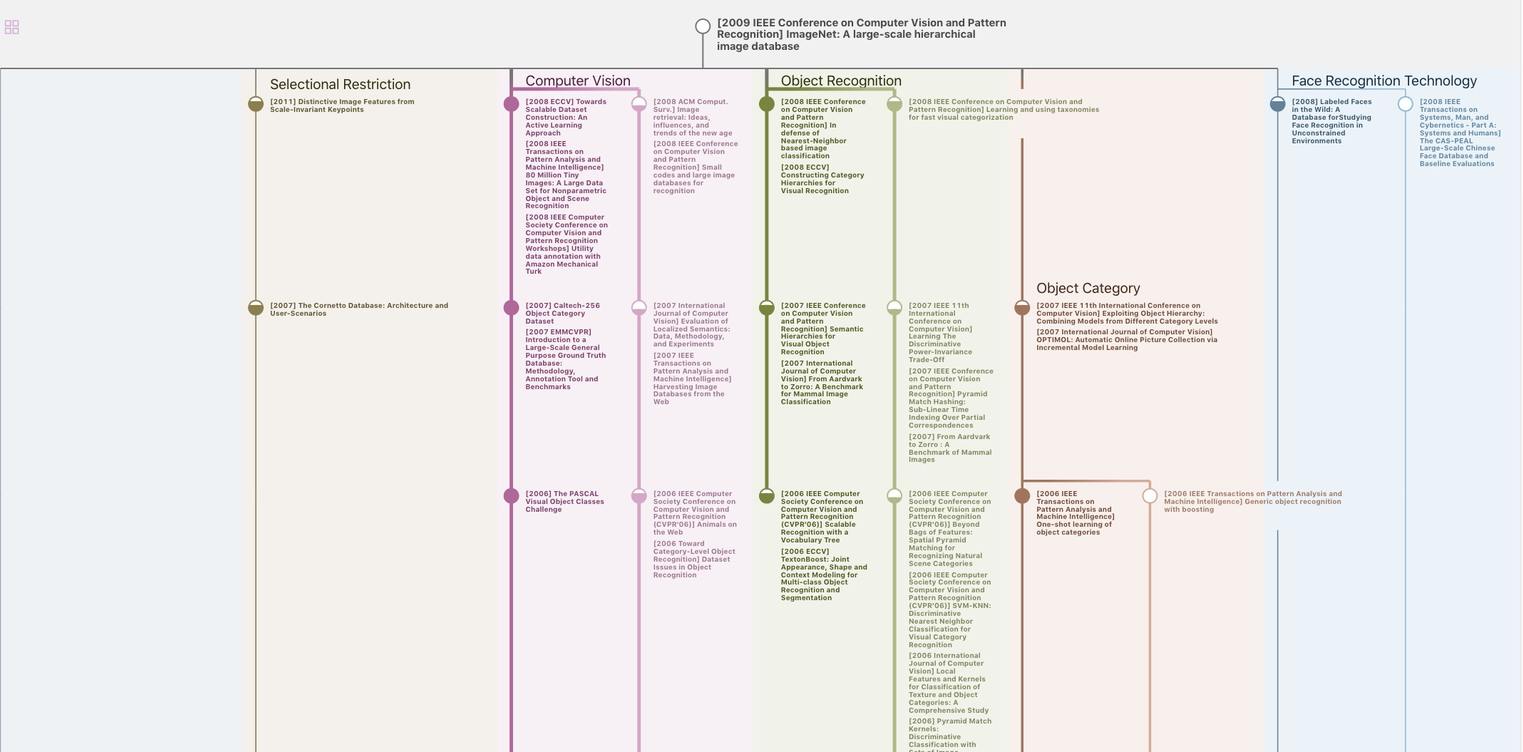
Generate MRT to find the research sequence of this paper
Chat Paper
Summary is being generated by the instructions you defined