Open-ICL: Open-Set Modulation Classification via Incremental Contrastive Learning.
IEEE transactions on neural networks and learning systems(2024)
摘要
Open-set modulation classification (OMC) of signals is a challenging task for handling "unknown" modulation types that are not included in the training dataset. This article proposes an incremental contrastive learning method for OMC, called Open-ICL, to accurately identify unknown modulation types of signals. First, a dual-path 1-D network (DONet) with a classification path (CLP) and a contrast path (COP) is designed to learn discriminative signal features cooperatively. In the COP, the deep features of the input signal are compared with the semantic feature centers (SFCs) of known classes calculated from the network, to infer its signal novelty. An unknown signal bank (USB) is defined to store unknown signals, and a novel moving intersection algorithm (MIA) is proposed to dynamically select reliable unknown signals for the USB. The "unknown" instances, together with SFCs, are continuously optimized and updated, facilitating the process of incremental learning. Furthermore, a dynamic adaptive threshold (DAT) strategy is proposed to enable Open-ICL to adaptively learn changing signal distributions. Extensive experiments are performed on two benchmark datasets, and the results demonstrate the effectiveness of Open-ICL for OMC.
更多查看译文
关键词
Adaptive threshold,incremental contrastive learning,modulation classification,moving intersection,open set,signal novelty
AI 理解论文
溯源树
样例
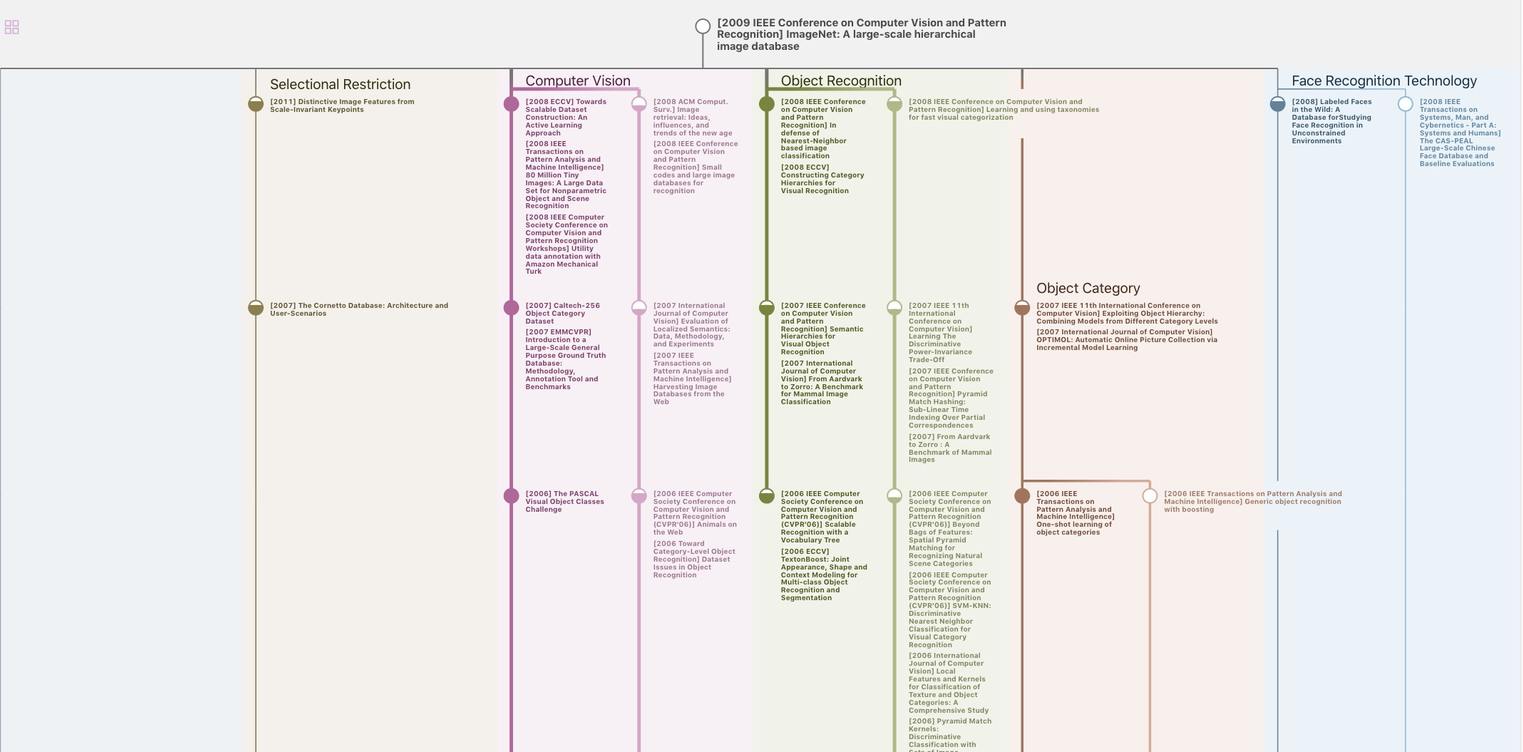
生成溯源树,研究论文发展脉络
Chat Paper
正在生成论文摘要