Active Parallel Teacher for Human-in-the-Loop Sim2Real Object Detection in Autonomous Haulage Trucks
IEEE Transactions on Intelligent Vehicles(2024)
摘要
Object detection for autonomous haulage trucks in open-pit mines is challenging due to unstructured environments. Collecting and annotating large-scale real-world images in these harsh conditions is extremely expensive. Inspired by parallel vision, virtual images have been used to supplement real-world data. However, models trained on virtual data exhibit poor performance in real mines due to the simulation-to-real (sim2real) domain shift. To address this issue, a human-in-the-loop algorithm called Active Parallel Teacher (APT) is introduced to select and annotate real images for sim2real object detection, in which the gradient information of a mean-teacher architecture is formulated as an important indicator for sample selection. Based on the gradient embeddings, three active sampling metrics are designed to measure sample importance from different perspectives including uncertainty, inter-domain difference, and diversity of target domain. Following that, an adaptive budget allocation strategy is presented to achieve the trade-off between uncertainty and difference along with different model training stages, with the purpose of maximizing the performance gain of human annotations. Finally, a Mean Teacher based domain adaptation process is developed to leverage fine-tuning and mix-training techniques to full utilize both labeled and unlabeled real data. Experimental results on the open-pit mine dataset demonstrate that our method can outperform other domain adaptation methods and attains 91% of the performance achieved under fully supervised conditions with only 7.5% of the real data labeled.
更多查看译文
关键词
Parallel vision,Object detection,Autonomous mining trucks,Active domain adaptation
AI 理解论文
溯源树
样例
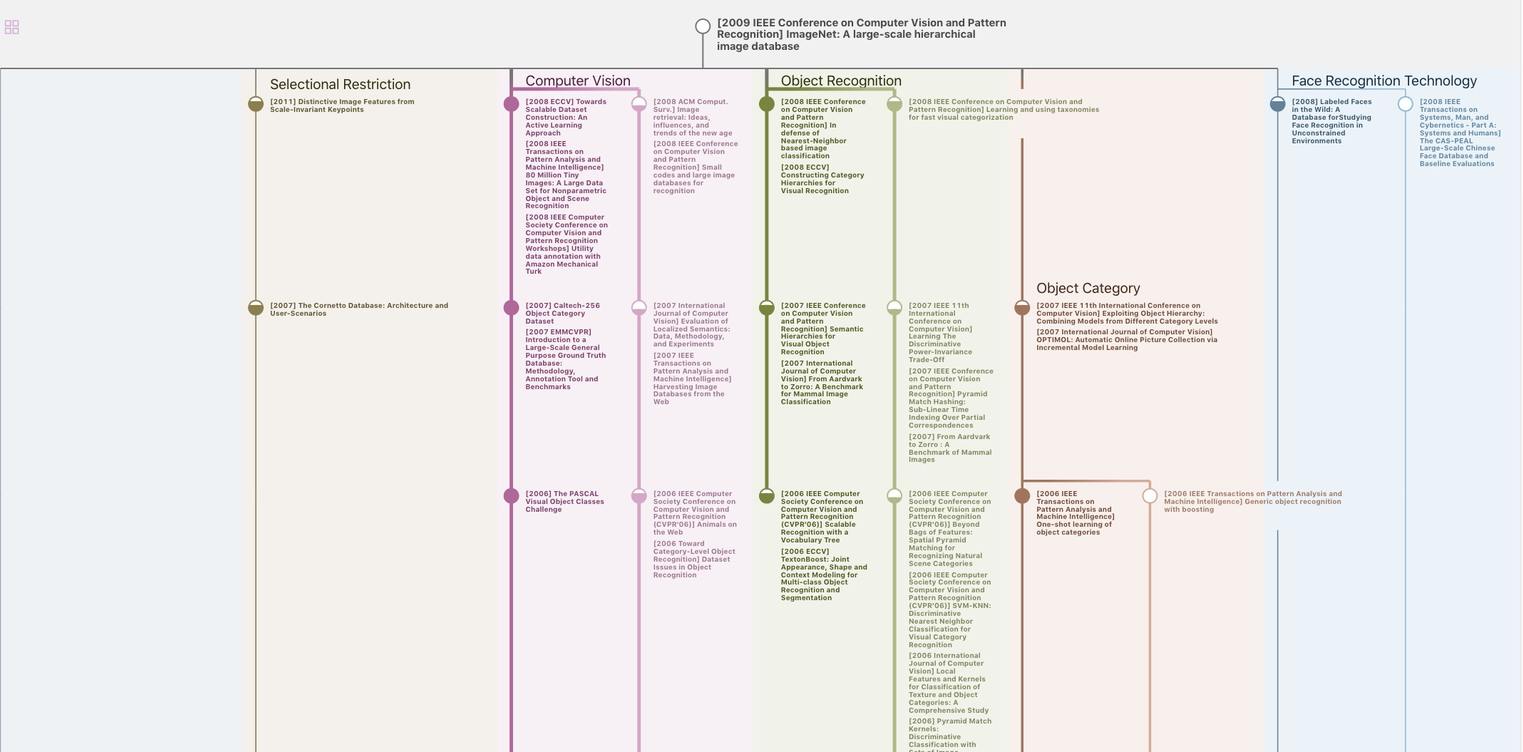
生成溯源树,研究论文发展脉络
Chat Paper
正在生成论文摘要