SPMLD: A skin pathological image dataset for non-melanoma with detailed lesion area annotation.
Computers in biology and medicine(2024)
Abstract
Skin tumors are the most common tumors in humans and the clinical characteristics of three common non-melanoma tumors (IDN, SK, BCC) are similar, resulting in a high misdiagnosis rate. The accurate differential diagnosis of these tumors needs to be judged based on pathological images. However, a shortage of experienced dermatological pathologists leads to bias in the diagnostic accuracy of these skin tumors in China. In this paper, we establish a skin pathological image dataset, SPMLD, for three non-melanoma to achieve automatic and accurate intelligent identification for them. Meanwhile, we propose a lesion-area-based enhanced classification network with the KLS module and an attention module. Specifically, we first collect thousands of H&E-stained tissue sections from patients with clinically and pathologically confirmed IDN, SK, and BCC from a single-center hospital. Then, we scan them to construct a pathological image dataset of these three skin tumors. Furthermore, we mark the complete lesion area of the entire pathology image to better learn the pathologist's diagnosis process. In addition, we applied the proposed network for lesion classification prediction on the SPMLD dataset. Finally, we conduct a series of experiments to demonstrate that this annotation and our network can effectively improve the classification results of various networks. The source dataset and code are available at https://github.com/efss24/SPMLD.git.
MoreTranslated text
AI Read Science
Must-Reading Tree
Example
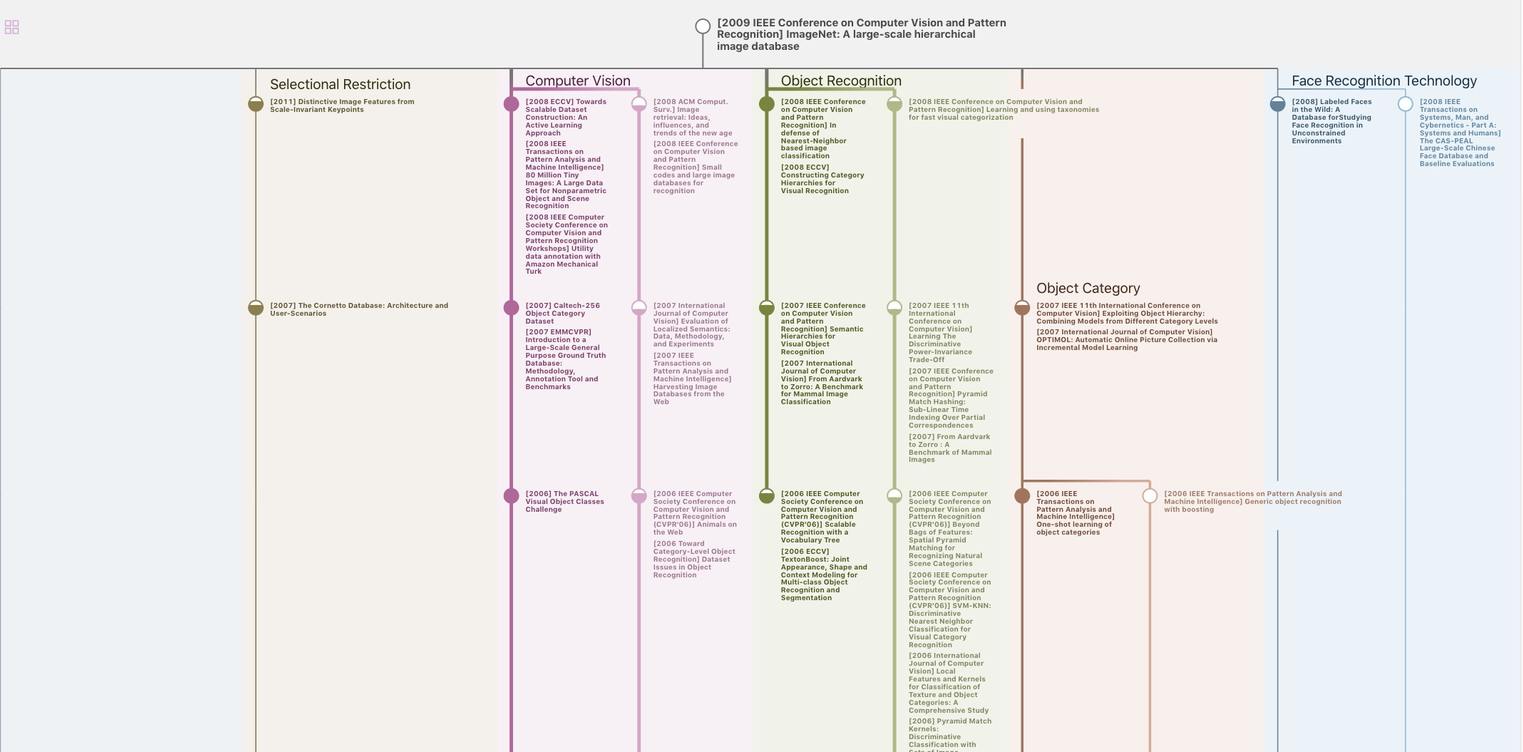
Generate MRT to find the research sequence of this paper
Chat Paper
Summary is being generated by the instructions you defined