LATextSpotter: Empowering Transformer Decoder with Length Perception Ability.
IEEE International Symposium on Circuits and Systems(2024)
摘要
Scene text spotting aims to integrate scene text detection and recognition into a unified framework. The existing transformer-based methods lack fine-grained positional information and linguistic information, limiting the convergence and performance of the model. In this paper, we propose a Length-Awear Text Spotter (LATextSpotter) to alleviate this problem by explicitly introducing two types of prior knowledge. First, the location of each character is initialized by coarsely locating the text instance and predicting the length, which provides effective guidance for the subsequent position-sensitive decoder. It is worth noting that the model requires only word-level supervision to achieve decent performance in the absence of expensive character-level annotations. Second, we design a mask prediction strategy based on the length information that masks character information at the feature level, and guides the model to predict the missing part. It empowers the decoder with language modeling capability without introducing extra modules. Additionally, considering the coordination between each module, a multi-stage training strategy is proposed to optimize the convergence process. Quantitative experiments demonstrate that LATextSpotter achieves the optimal end-to-end performance on arbitrary-shaped benchmarks by 76.6% and competitive spotting performance on multi-oriented datasets.
更多查看译文
关键词
component,formatting,style,styling,insert
AI 理解论文
溯源树
样例
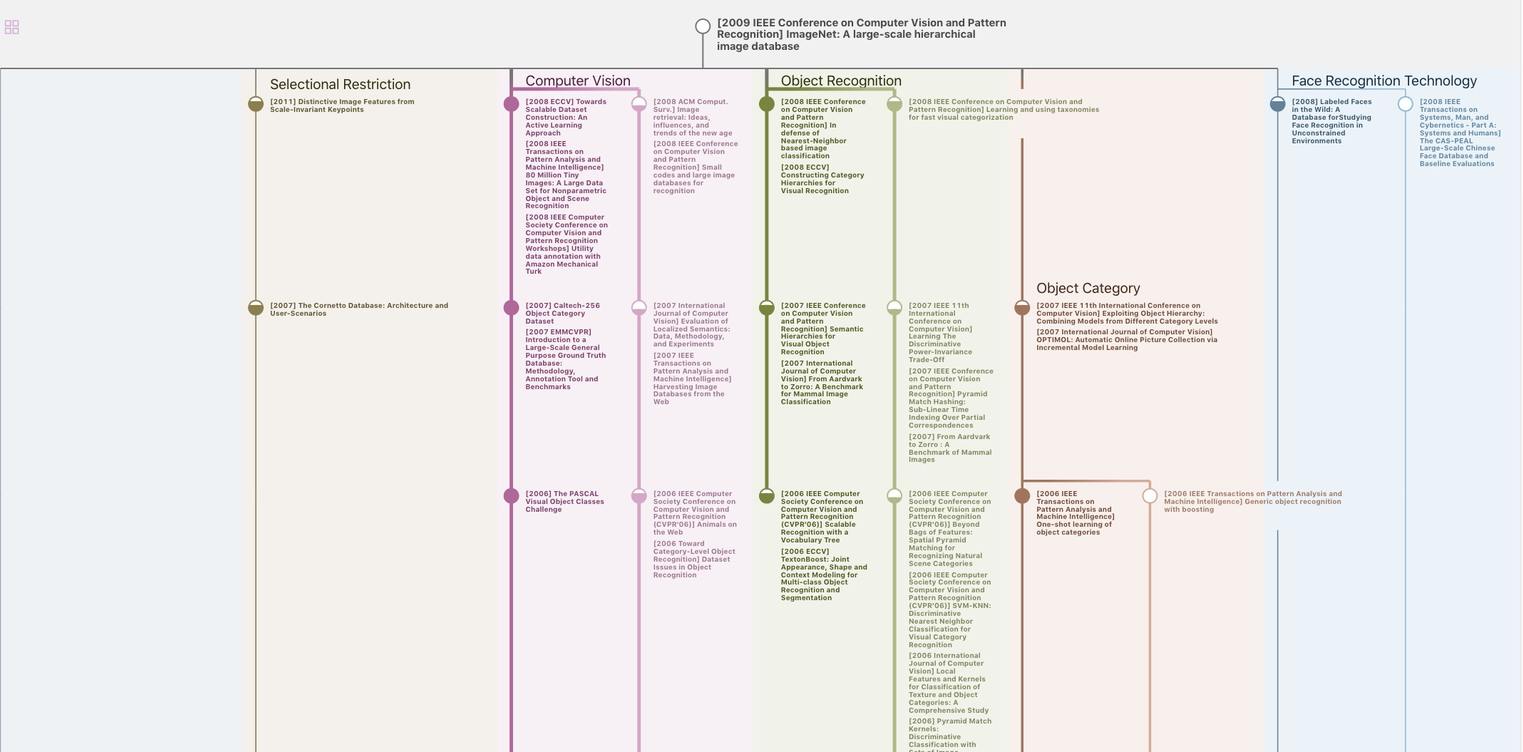
生成溯源树,研究论文发展脉络
Chat Paper
正在生成论文摘要