SPAT: FPGA-based Sparsity-Optimized Spiking Neural Network Training Accelerator with Temporal Parallel Dataflow
2024 IEEE International Symposium on Circuits and Systems (ISCAS)(2024)
摘要
Spiking neural networks (SNNs), as biologically inspired computational models, possess significant advantages in energy efficiency due to their event-driven operations. However, challenges remain in attaining high computational efficiency for SNN training. In this work, we propose a novel SNN training accelerator employing temporal parallelism and sparsity optimizations to achieve superior efficiency. A temporal parallel dataflow is designed to concurrently integrate spikes across multiple time steps, enhancing throughput and data reuse. To reduce latency and improve energy efficiency, we leverage the sparsity of SNNs and employ methods such as zero gating and zero skipping. Implemented on a field-programmable gate array (FPGA), the proposed training accelerator demonstrates 2.3-fold speedup and 15.7-fold energy reduction compared to NVIDIA A100 GPU on N-MNIST dataset.
更多查看译文
关键词
Training,Spiking Neural Networks,FPGA,Accelerator
AI 理解论文
溯源树
样例
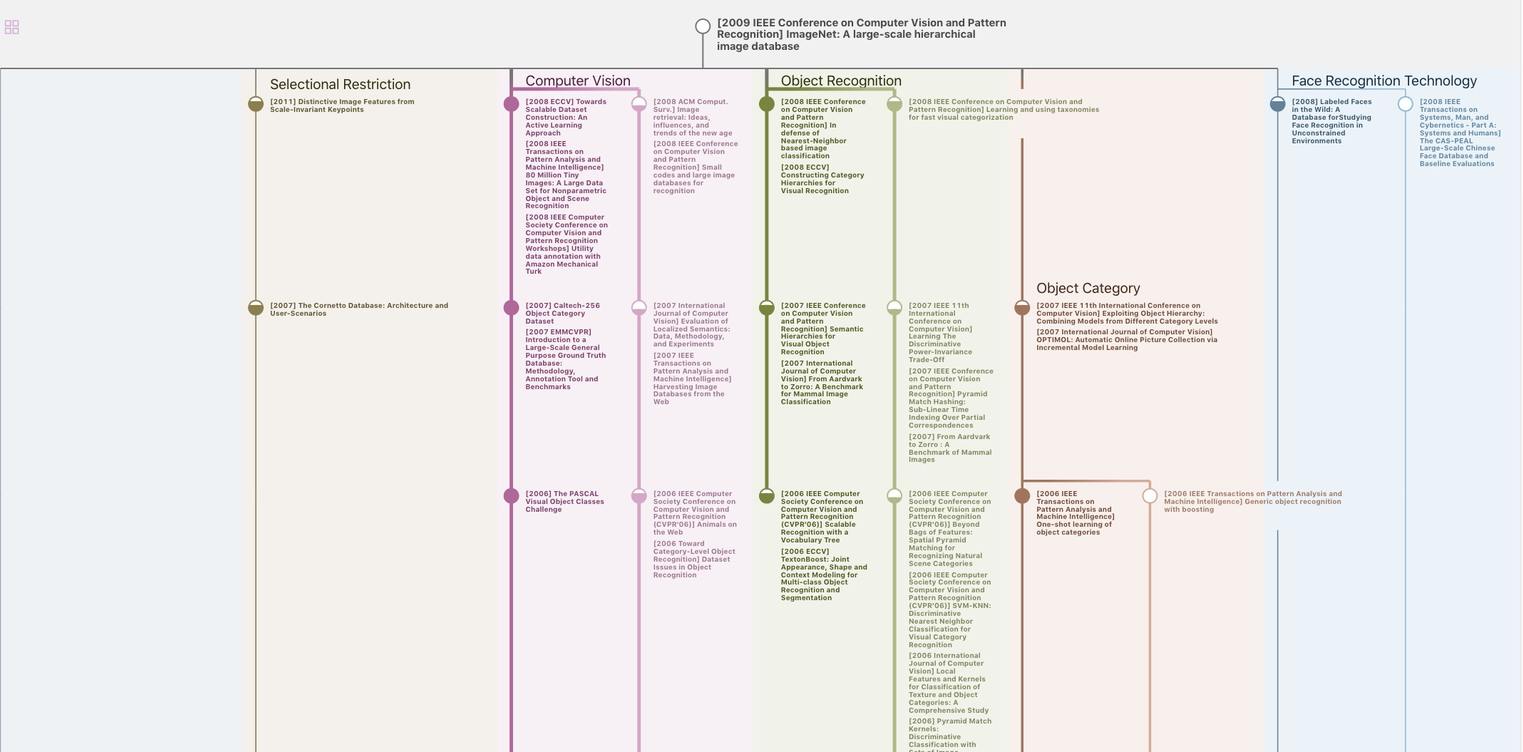
生成溯源树,研究论文发展脉络
Chat Paper
正在生成论文摘要