Unsupervised Image Enhancement Via Contrastive Learning
2024 IEEE International Symposium on Circuits and Systems (ISCAS)(2024)
摘要
Recent years have witnessed significant achievements for image enhancement tasks. However, many advanced algorithms are trained in a supervised manner and thus rely on a huge collection of paired data, for which the collection is itself a challenge especially for real-world scenarios. We address this issue by proposing a novel GAN framework designed for unsupervised training. To be specific, our approach introduces a contrastive loss to ensure that the content remains consistent across multiple scales in both input and output representations. In addition, we propose a multi-scale discriminator to strengthen the adversarial learning. Extensive experiments conducted in this paper showed that our algorithm achieved state-of-the-art performance on MIT-Adobe-FiveK dataset both quantitively and qualitatively.
更多查看译文
关键词
Image enhancement,unsupervised learning,contrastive learning,generative adversarial nets
AI 理解论文
溯源树
样例
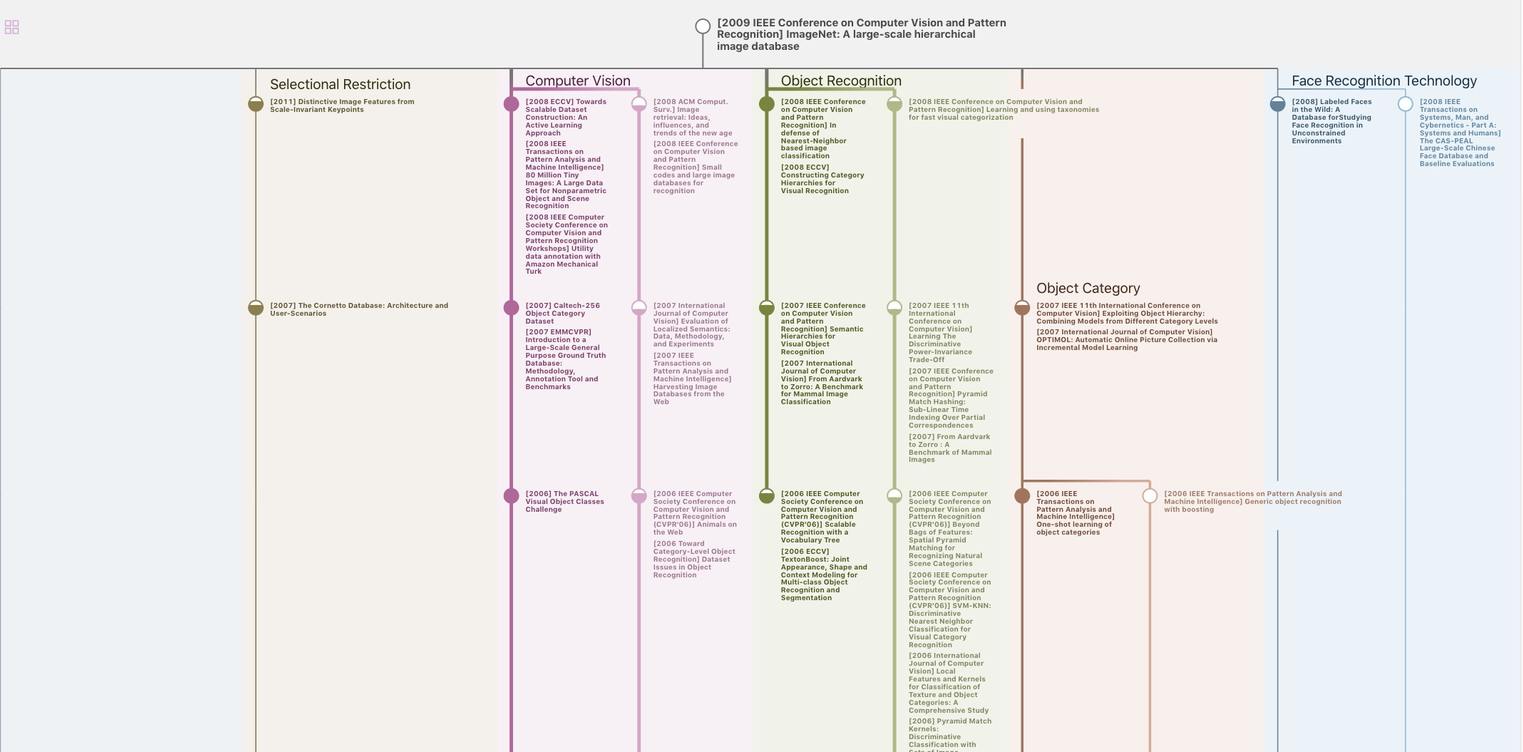
生成溯源树,研究论文发展脉络
Chat Paper
正在生成论文摘要