Self-Supervised Learning on Small In-Domain Datasets Can Overcome Supervised Learning in Remote Sensing
IEEE Journal of Selected Topics in Applied Earth Observations and Remote Sensing(2024)
Abstract
The availability of high-resolution satellite images has accelerated the creation of new datasets designed to tackle broader remote sensing (RS) problems. Although popular tasks like scene classification have received significant attention, the recent release of the Land-1.0 RS dataset (https://doi.org/10.5281/zenodo.7858952) marks the initiation of endeavors to estimate land-use and land-cover (LULC) fraction values per RGB satellite image. This challenging problem involves estimating LULC composition, i.e., the proportion of different LULC classes from satellite imagery, with major applications in environmental monitoring, agricultural/urban planning, and climate change studies. Currently, supervised deep learning models—the state-of-the-art in image classification—require large volumes of labeled training data to provide good generalization. To face the challenges posed by the scarcity of labeled RS data, self-supervised learning (SSL) models have recently emerged, learning directly from unlabeled data by leveraging the underlying structure. This is the first paper to investigate the performance of SSL in LULC fraction estimation on RGB satellite patches using in-domain knowledge. We also performed a complementary analysis on LULC scene classification. Specifically, we pretrained Barlow Twins, MoCov2, SimCLR, and SimSiam SSL models with ResNet-18 using the Sentinel2GlobalLULC small RS dataset (https://doi.org/10.5281/zenodo.6941662) and then performed transfer learning to downstream tasks on Land-1.0. Our experiments demonstrate that SSL achieves competitive or slightly better results when trained on a smaller high-quality in-domain dataset of 194,877 samples compared to the supervised model trained on ImageNet-1k with 1,281,167 samples. This outcome highlights the effectiveness of SSL using in-distribution datasets, demonstrating efficient learning with fewer but more relevant data.
MoreTranslated text
Key words
Remote sensing (RS),deep learning,self-supervised learning (SSL),land use and land cover (LULC),fraction estimation,multispectral data,RGB satellite images
AI Read Science
Must-Reading Tree
Example
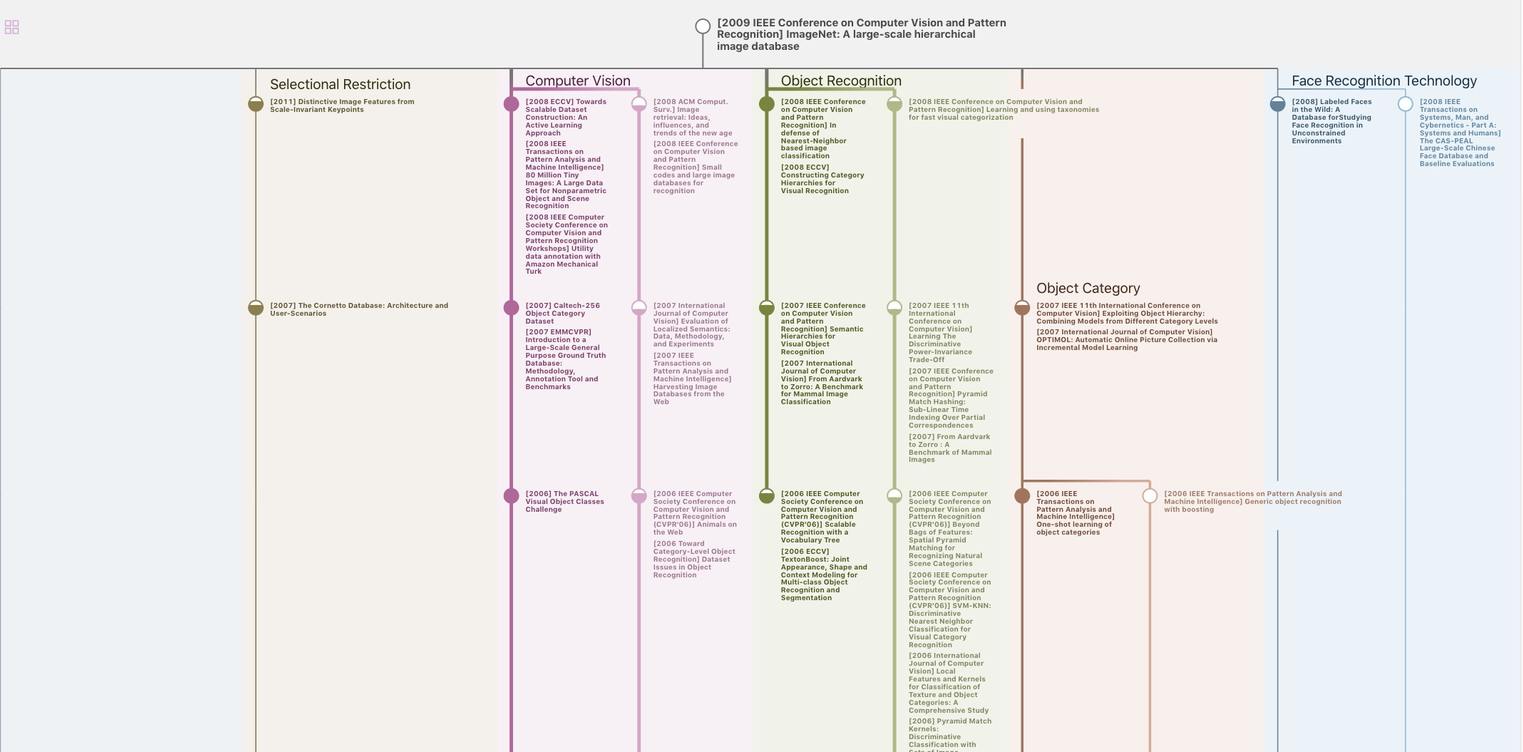
Generate MRT to find the research sequence of this paper
Chat Paper
Summary is being generated by the instructions you defined