PSTNet: Enhanced Polyp Segmentation With Multi-Scale Alignment and Frequency Domain Integration.
IEEE journal of biomedical and health informatics(2024)
摘要
Accurate segmentation of colorectal polyps in colonoscopy images is crucial for effective diagnosis and management of colorectal cancer (CRC). However, current deep learning-based methods primarily rely on fusing RGB information across multiple scales, leading to limitations in accurately identifying polyps due to restricted RGB domain information and challenges in feature misalignment during multi-scale aggregation. To address these limitations, we propose the Polyp Segmentation Network with Shunted Transformer (PSTNet), a novel approach that integrates both RGB and frequency domain cues present in the images. PSTNet comprises three key modules: the Frequency Characterization Attention Module (FCAM) for extracting frequency cues and capturing polyp characteristics, the Feature Supplementary Alignment Module (FSAM) for aligning semantic information and reducing misalignment noise, and the Cross Perception localization Module (CPM) for synergizing frequency cues with high-level semantics to achieve efficient polyp segmentation. Extensive experiments on challenging datasets demonstrate PSTNet's significant improvement in polyp segmentation accuracy across various metrics, consistently outperforming state-of-the-art methods. The integration of frequency domain cues and the novel architectural design of PSTNet contribute to advancing computer-assisted polyp segmentation, facilitating more accurate diagnosis and management of CRC. Our source code is available for reference at https://github.com/clearxu/PSTNet.
更多查看译文
关键词
Polyp segmentation,shunted transformer,multi-scale fusion
AI 理解论文
溯源树
样例
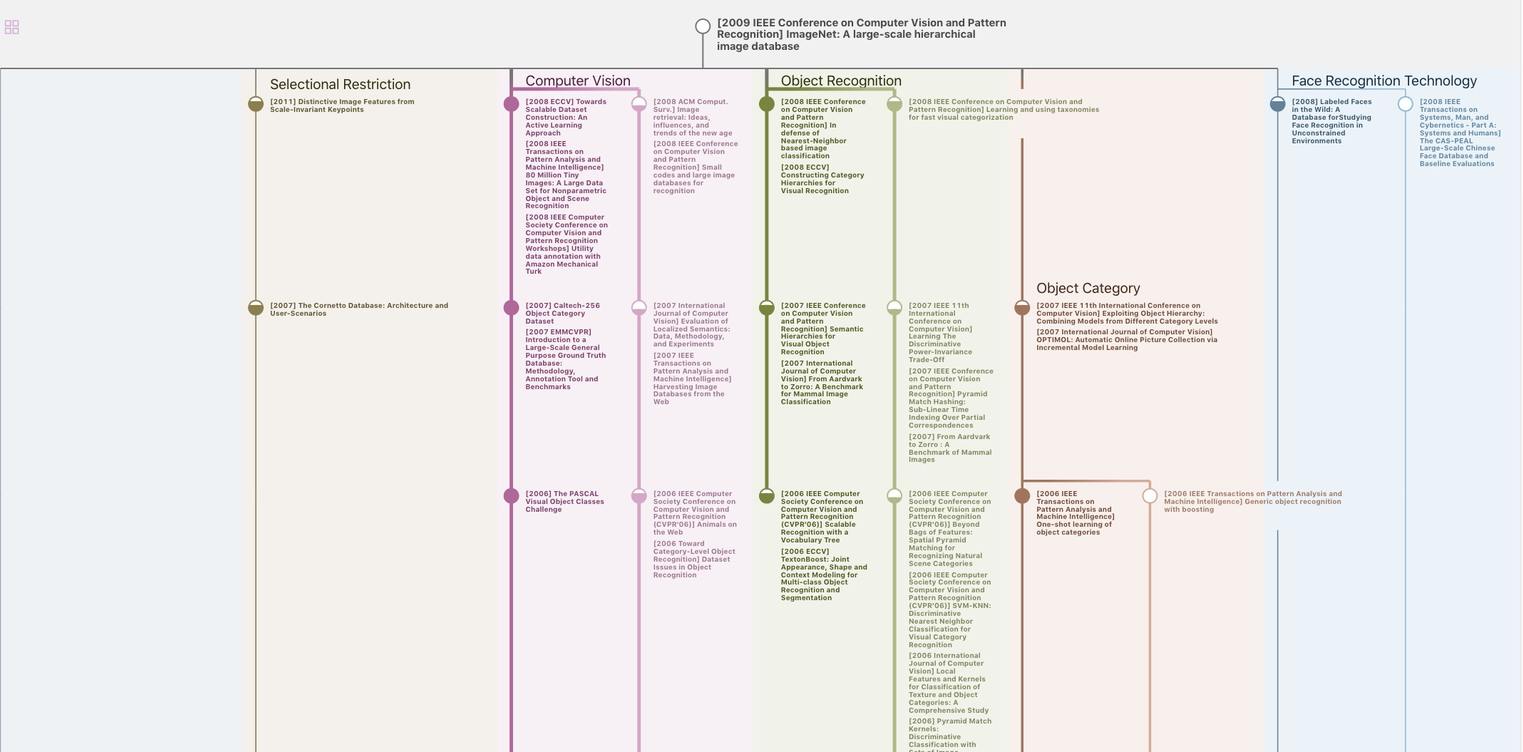
生成溯源树,研究论文发展脉络
Chat Paper
正在生成论文摘要