Consistency Flow Matching: Defining Straight Flows with Velocity Consistency
arxiv(2024)
Abstract
Flow matching (FM) is a general framework for defining probability paths via
Ordinary Differential Equations (ODEs) to transform between noise and data
samples. Recent approaches attempt to straighten these flow trajectories to
generate high-quality samples with fewer function evaluations, typically
through iterative rectification methods or optimal transport solutions. In this
paper, we introduce Consistency Flow Matching (Consistency-FM), a novel FM
method that explicitly enforces self-consistency in the velocity field.
Consistency-FM directly defines straight flows starting from different times to
the same endpoint, imposing constraints on their velocity values. Additionally,
we propose a multi-segment training approach for Consistency-FM to enhance
expressiveness, achieving a better trade-off between sampling quality and
speed. Preliminary experiments demonstrate that our Consistency-FM
significantly improves training efficiency by converging 4.4x faster than
consistency models and 1.7x faster than rectified flow models while achieving
better generation quality. Our code is available at:
https://github.com/YangLing0818/consistency_flow_matching
MoreTranslated text
AI Read Science
Must-Reading Tree
Example
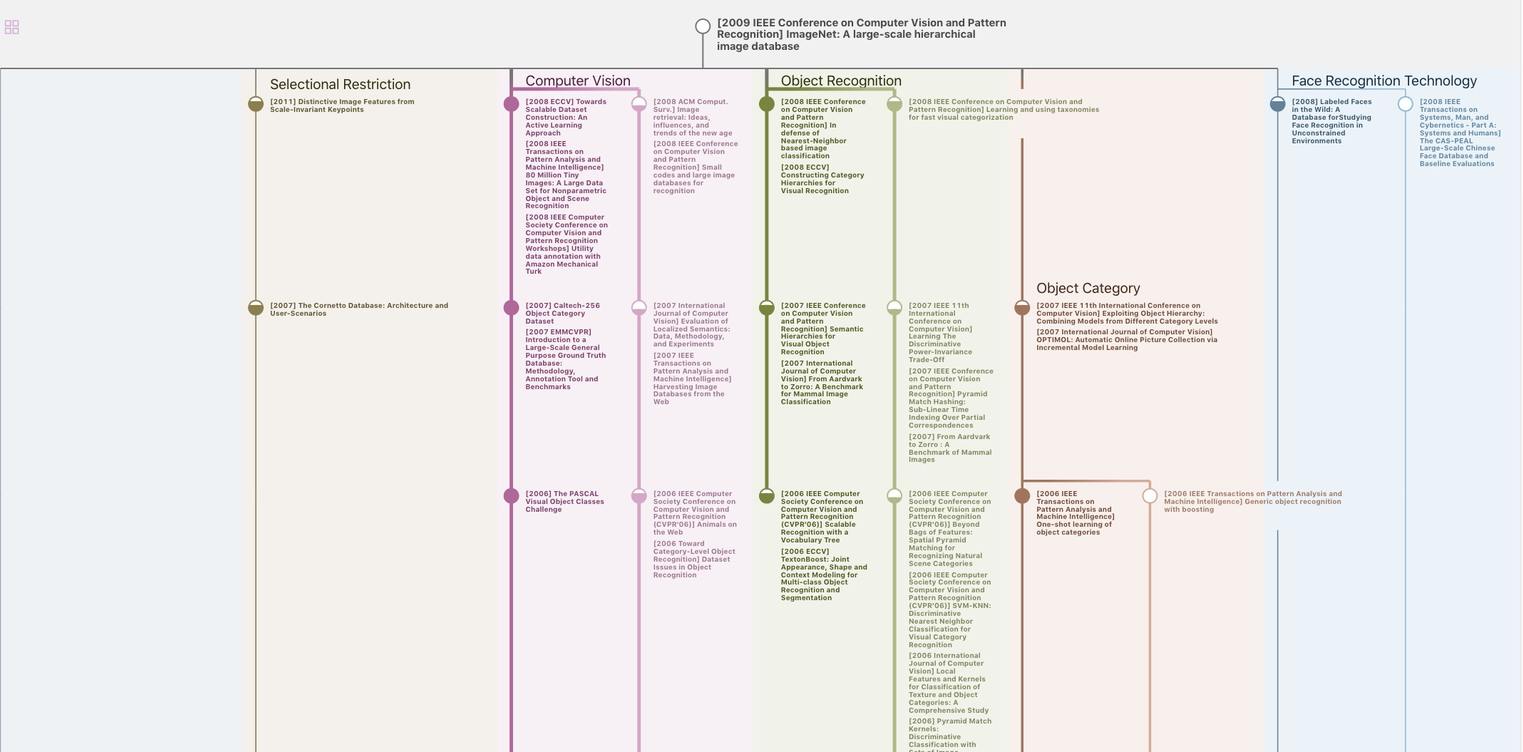
Generate MRT to find the research sequence of this paper
Chat Paper
Summary is being generated by the instructions you defined