MALT Powers Up Adversarial Attacks
arxiv(2024)
Abstract
Current adversarial attacks for multi-class classifiers choose the target
class for a given input naively, based on the classifier's confidence levels
for various target classes. We present a novel adversarial targeting method,
MALT - Mesoscopic Almost Linearity Targeting, based on medium-scale
almost linearity assumptions. Our attack wins over the current state of the art
AutoAttack on the standard benchmark datasets CIFAR-100 and ImageNet and for a
variety of robust models. In particular, our attack is five times faster
than AutoAttack, while successfully matching all of AutoAttack's successes and
attacking additional samples that were previously out of reach. We then prove
formally and demonstrate empirically that our targeting method, although
inspired by linear predictors, also applies to standard non-linear models.
MoreTranslated text
AI Read Science
Must-Reading Tree
Example
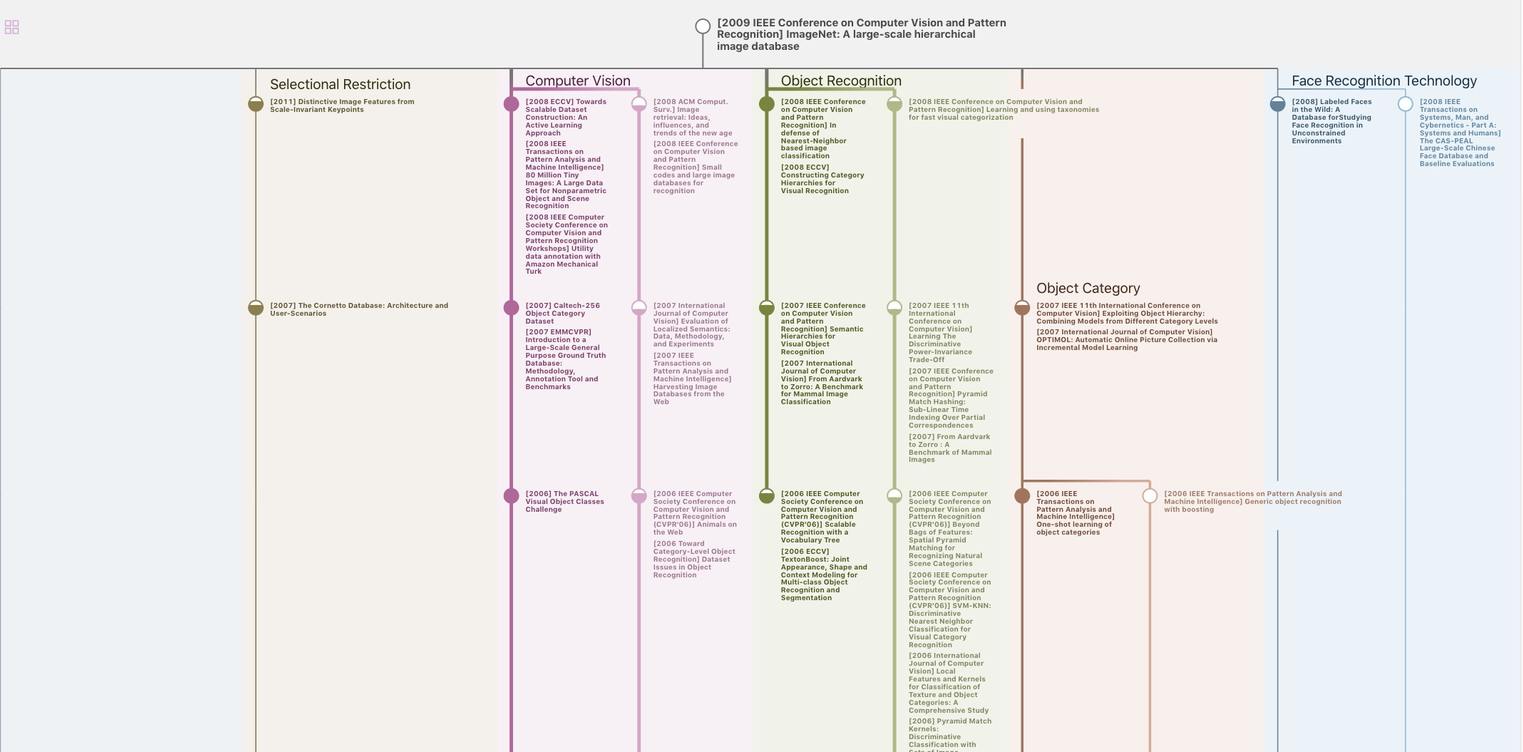
Generate MRT to find the research sequence of this paper
Chat Paper
Summary is being generated by the instructions you defined