Coderivative-Based Newton Methods with Wolfe Linesearch for Nonsmooth Optimization
arxiv(2024)
摘要
This paper introduces and develops novel coderivative-based Newton methods
with Wolfe linesearch conditions to solve various classes of problems in
nonsmooth optimization. We first propose a generalized regularized Newton
method with Wolfe linesearch (GRNM-W) for unconstrained C^1,1 minimization
problems (which are second-order nonsmooth) and establish global as well as
local superlinear convergence of their iterates. To deal with convex composite
minimization problems (which are first-order nonsmooth and can be constrained),
we combine the proposed GRNM-W with two algorithmic frameworks: the
forward-backward envelope and the augmented Lagrangian method resulting in the
two new algorithms called CNFB and CNAL, respectively. Finally, we present
numerical results to solve Lasso and support vector machine problems appearing
in, e.g., machine learning and statistics, which demonstrate the efficiency of
the proposed algorithms.
更多查看译文
AI 理解论文
溯源树
样例
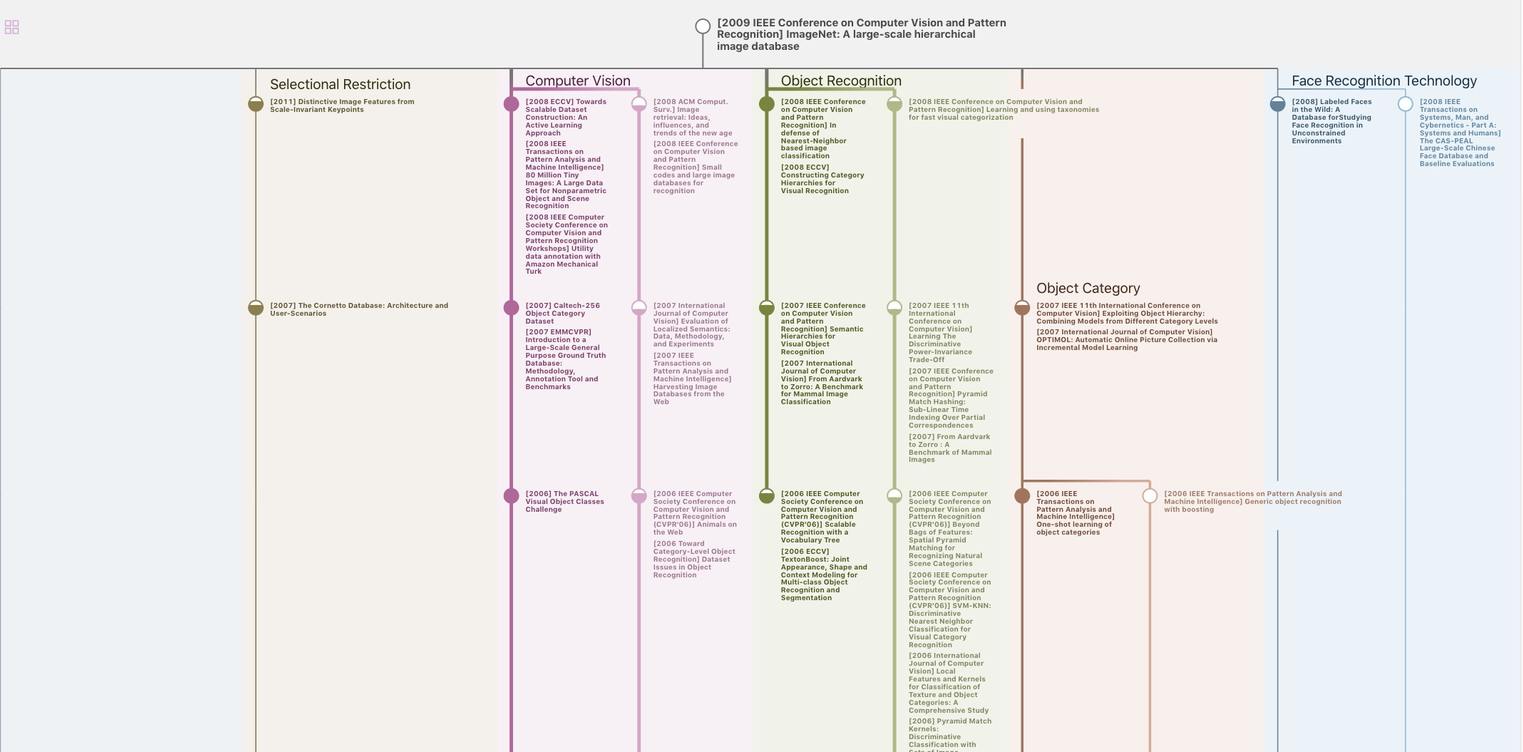
生成溯源树,研究论文发展脉络
Chat Paper
正在生成论文摘要