Ego-Foresight: Agent Visuomotor Prediction As Regularization for RL
arxiv(2024)
摘要
Despite the significant advancements in Deep Reinforcement Learning (RL) observed in the last decade, the amount of training experience necessary to learn effective policies remains one of the primary concerns both in simulated and real environments. Looking to solve this issue, previous work has shown that improved training efficiency can be achieved by separately modeling agent and environment, but usually requiring a supervisory agent mask. In contrast to RL, humans can perfect a new skill from a very small number of trials and in most cases do so without a supervisory signal, making neuroscientific studies of human development a valuable source of inspiration for RL. In particular, we explore the idea of motor prediction, which states that humans develop an internal model of themselves and of the consequences that their motor commands have on the immediate sensory inputs. Our insight is that the movement of the agent provides a cue that allows the duality between agent and environment to be learned. To instantiate this idea, we present Ego-Foresight, a self-supervised method for disentangling agent and environment based on motion and prediction. Our main finding is that visuomotor prediction of the agent provides regularization to the RL algorithm, by encouraging the actions to stay within predictable bounds. To test our approach, we first study the ability of our model to visually predict agent movement irrespective of the environment, in real-world robotic interactions. Then, we integrate Ego-Foresight with a model-free RL algorithm to solve simulated robotic manipulation tasks, showing an average improvement of 23
更多查看译文
AI 理解论文
溯源树
样例
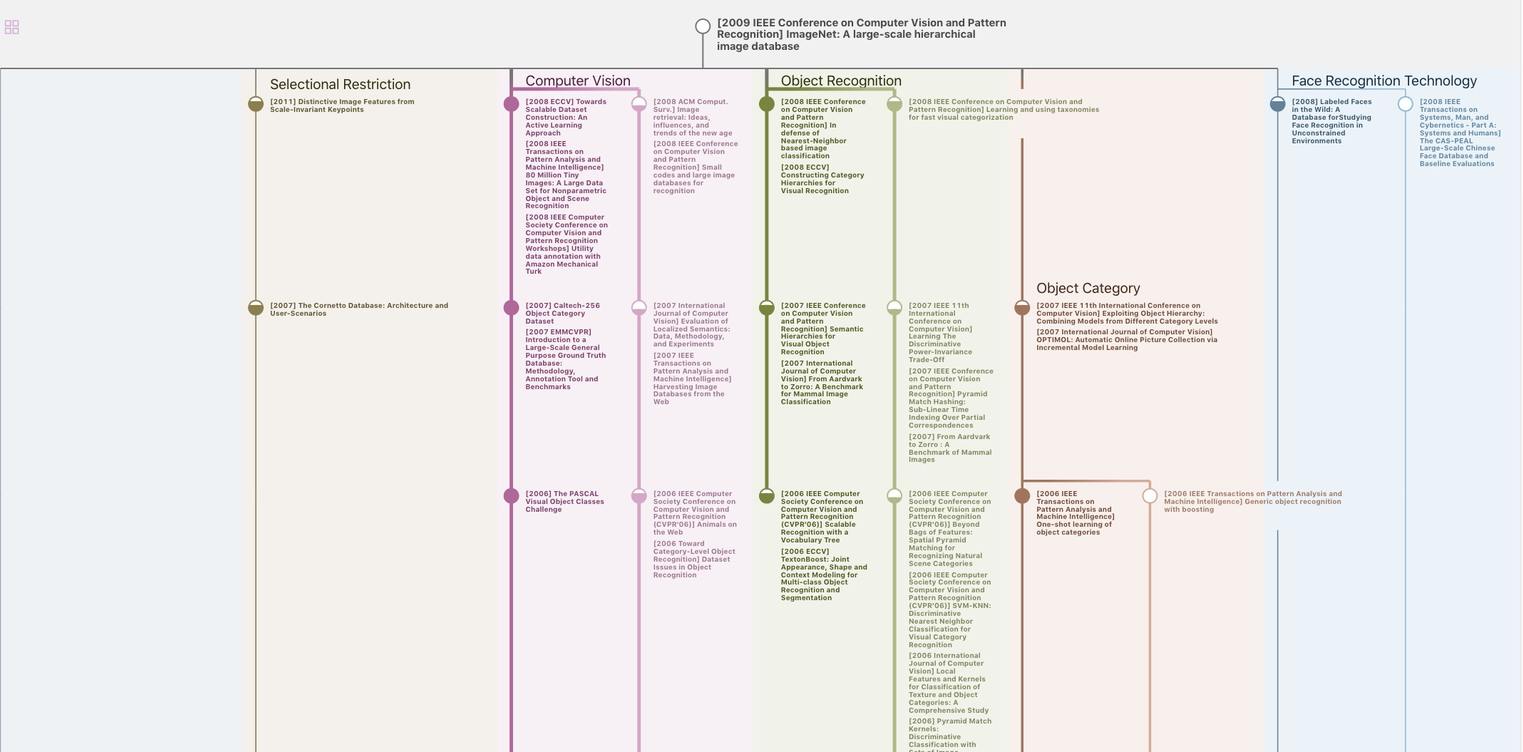
生成溯源树,研究论文发展脉络
Chat Paper
正在生成论文摘要