Small but Fair! Fairness for Multimodal Human-Human and Robot-Human Mental Wellbeing Coaching
arxiv(2024)
Abstract
In recent years, the affective computing (AC) and human-robot interaction
(HRI) research communities have put fairness at the centre of their research
agenda. However, none of the existing work has addressed the problem of machine
learning (ML) bias in HRI settings. In addition, many of the current datasets
for AC and HRI are "small", making ML bias and debias analysis challenging.
This paper presents the first work to explore ML bias analysis and mitigation
of three small multimodal datasets collected within both a human-human and
robot-human wellbeing coaching settings. The contributions of this work
includes: i) being the first to explore the problem of ML bias and fairness
within HRI settings; and ii) providing a multimodal analysis evaluated via
modelling performance and fairness metrics across both high and low-level
features and proposing a simple and effective data augmentation strategy
(MixFeat) to debias the small datasets presented within this paper; and iii)
conducting extensive experimentation and analyses to reveal ML fairness
insights unique to AC and HRI research in order to distill a set of
recommendations to aid AC and HRI researchers to be more engaged with
fairness-aware ML-based research.
MoreTranslated text
AI Read Science
Must-Reading Tree
Example
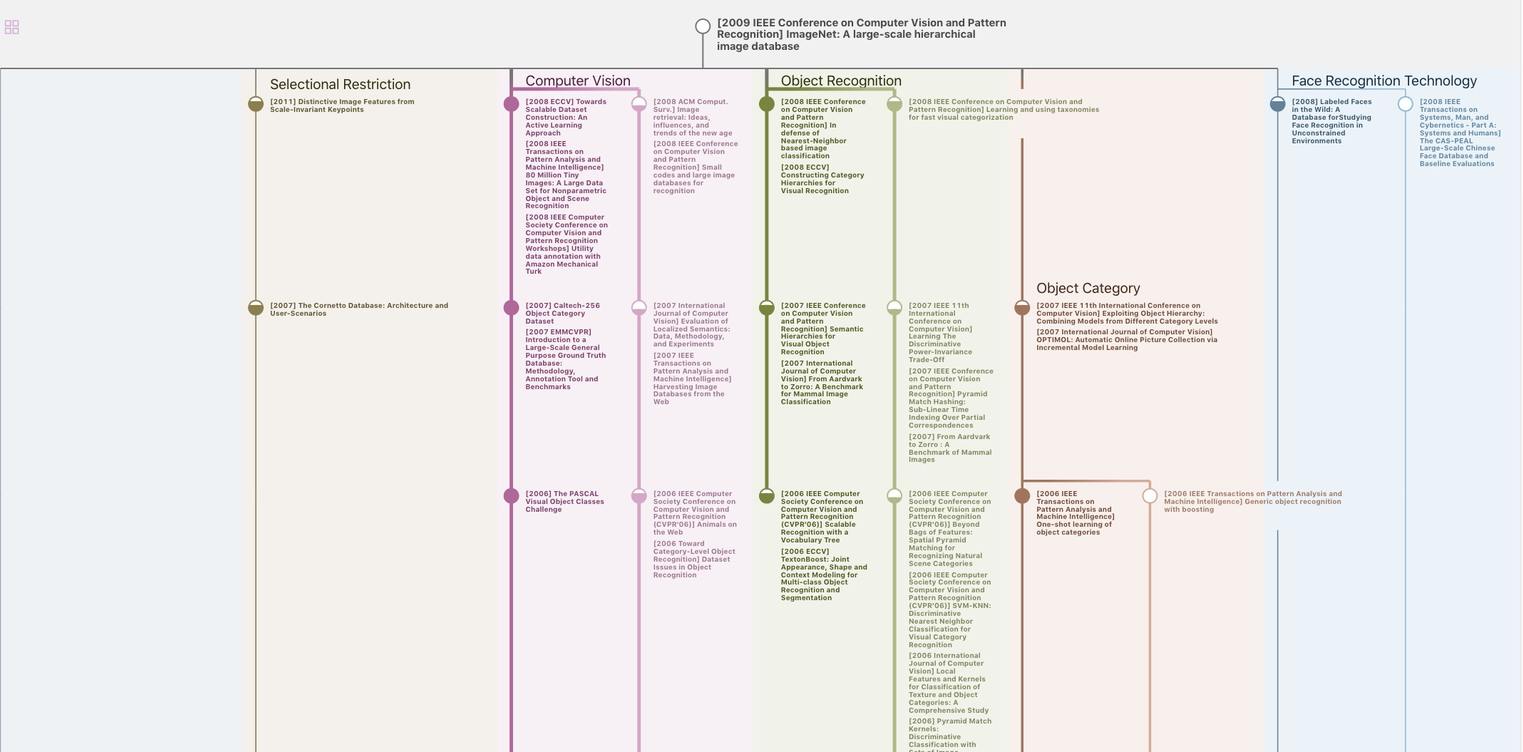
Generate MRT to find the research sequence of this paper
Chat Paper
Summary is being generated by the instructions you defined