Zero-One Attack: Degrading Closed-Loop Neural Network Control Systems using State-Time Perturbations.
International Conference on Cyber-Physical Systems(2024)
摘要
Autonomous cyber-physical systems with deep-learning components have shown great promise but have so far enjoyed limited adoption. Part of the problem is that, beyond average-case analysis, guaranteeing robustness and reasoning about worst-case behaviors in these systems is difficult. Previous research has developed attacks that can degrade a system’s performance using small perturbations on observed states, as well as ways to retrain the networks that appear to make them robust to such attacks. In this work, we advance the state of the art by developing a new method called the Zero-One Attack, which is able to bypass the current strongest defense.The Zero-One Attack minimizes reward by combining an outer loop zeroth-order gradient-free optimization with an inner loop, first-order gradient-based method. This setup both reduces the dimensionality of the zeroth-order optimization problem and leverages efficient gradient-based search methods for neural networks, such as projected gradient descent. In addition to state observation noise, we consider a new attack model with bounded perturbations to the execution time instant of the control policy, as real-time schedulers usually guarantee execution once per period, which may not be strictly periodic. On the Mujoco Half Cheetah system with the best current defense, the Zero-One Attack degrades the performance 195% beyond the state-of-the-art, which increases to 522% more degradation when also attacking timing jitter.
更多查看译文
关键词
CPS,deep-learning,sensor noise,optimization
AI 理解论文
溯源树
样例
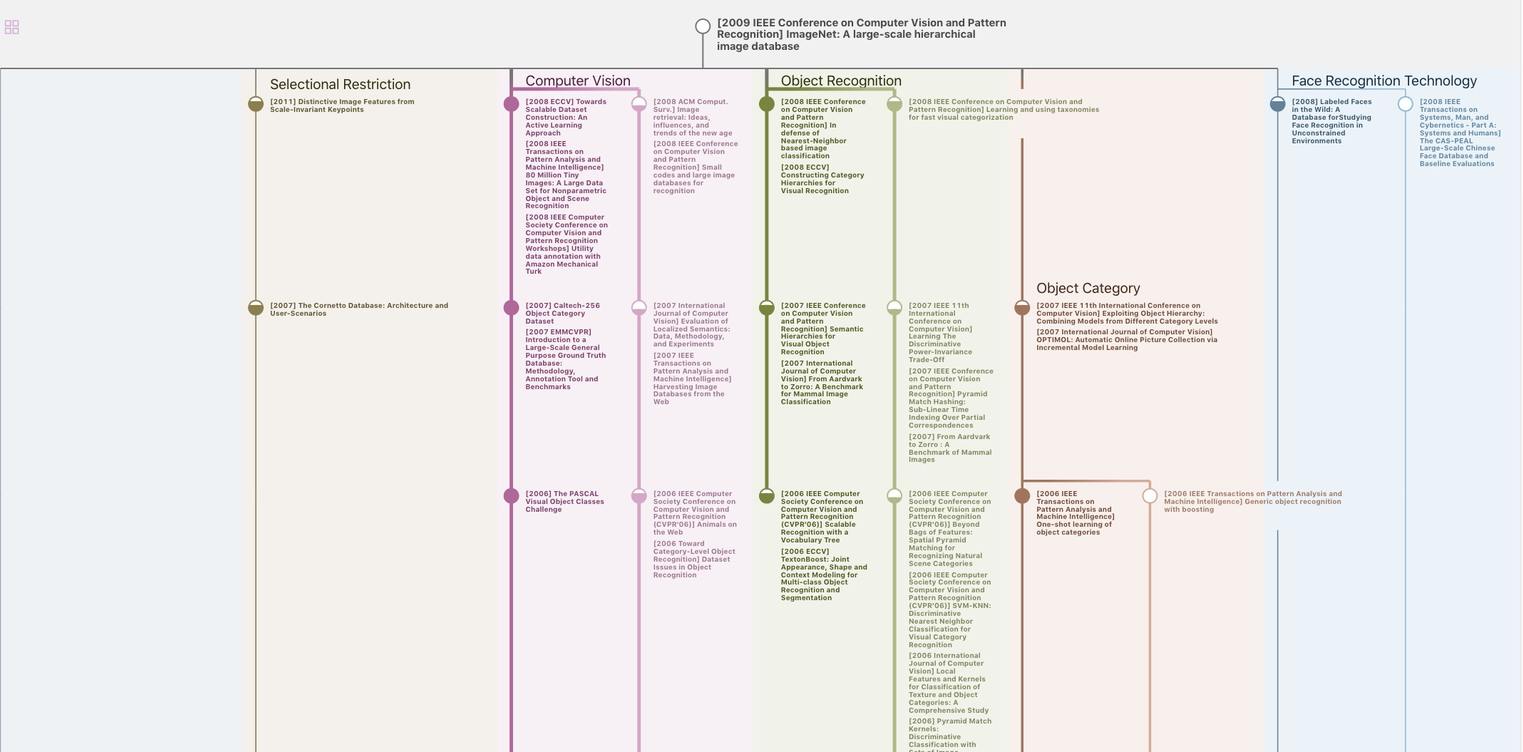
生成溯源树,研究论文发展脉络
Chat Paper
正在生成论文摘要