Few-shot learning for structural health diagnosis of civil infrastructure
Advanced Engineering Informatics(2024)
Abstract
The successful development of deep learning and computer vision techniques has recently revolutionized structural health diagnosis (SHD) during life-cycle construction, inspection, maintenance, and disaster prevention for civil infrastructure. Multi-source big data of structural health monitoring (SHM) and inspection are projected into a high-level feature space, and data-driven models are established to map the relationships between inputs and outputs and dig out embedded structural behaviors and implicit physical mechanisms. However, the model performance highly relies on the extensive amount and diversity, intra-class completeness, and inter-class balance of training data, and the generalization ability on scarce data with specific features and particular patterns is challenging under real-world scenarios. To address the above challenges, few-shot learning (FSL) has emerged as a cutting-edge machine learning technique that designs training strategies using only a small amount of annotated data under a limited supervision regime to enhance effectiveness and generalization ability. This article systematically summarizes recent advances in FSL algorithms and the corresponding applications in SHD for civil infrastructure. A unified mathematical framework of FSL is formulated, and an FSL taxonomy is summarized according to intrinsic learning mechanisms and implementation principles, including metric learning-based, optimization-based, transfer learning-based, and generative model-based methods. Various applications of SHD for civil infrastructure under real-world scenarios are reviewed, including remote sensing monitoring, structural damage recognition, post-disaster safety evaluation, and construction risk assessment. Error analyses of approximation, generalization, and optimization errors corresponding to the four paradigms mentioned above are formulated for FSL-based data-driven modeling, which exactly acts as the logical connection with how new thoughts of FSL-based SHD should be designed. Finally, potential prospects of FSL-based SHD are outlined to design weakly supervised FSL considering low-quality data from SHM systems, develop multi-modal FSL for multi-source SHM data, establish cross-task FSL with high commonality and generality for various SHD tasks, and construct lightweight FSL framework with model compression for real-world applications of SHD considering the super-large stream of monitoring data.
MoreTranslated text
Key words
Structural Health Diagnosis,Few-Shot Learning,Limited Supervision Information,Error Analysis and Reduction,Civil Infrastructure
AI Read Science
Must-Reading Tree
Example
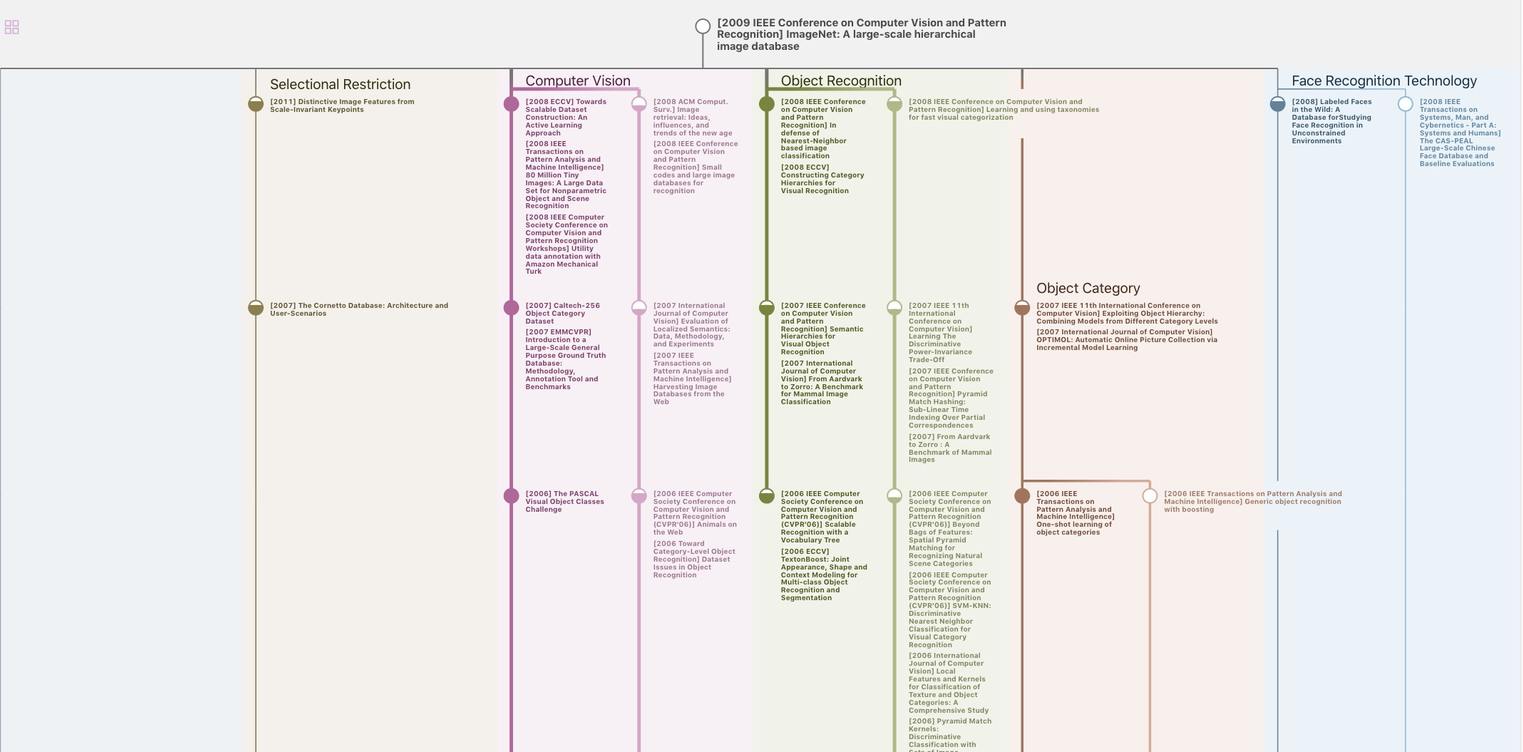
Generate MRT to find the research sequence of this paper
Chat Paper
Summary is being generated by the instructions you defined