Time sparse S-transform (TSST) and its applications
IEEE Transactions on Geoscience and Remote Sensing(2024)
摘要
In existing window time-frequency analysis methods, its resolution is commonly limited by the uncertainty principle. The time resolution and the frequency resolution restrict each other and cannot reach the maximum at the same time. For this reason, we employ the secondary processing technology of the time-frequency spectrum to enhance the time resolution of the S-transform. By performing multi-band filtering with a filter bank, the S-transform is capable of receiving the signal under different frequencies and its time-frequency coefficient. The window size is different, that is, the low-frequency time window is long, resulting in low time resolution and high-frequency resolution. The high-frequency time window is short, which makes the time resolution high and the frequency resolution low; however, the window size for the time component of each frequency is fixed. Based on the above idea, we perform "de-window" processing for each frequency component signal. We assume that the time-frequency spectrum is sparse without "windowing", so the window effect can be removed by sparse inversion. Based on the alternating direction method of multipliers (ADMM), we use the non-negative penalty terms and sparse terms for the joint constraints to solve the optimization problem, and obtain the sparse time-frequency spectrum to enhance the time resolution. This time sparse S-transform (TSST) obtained by the proposed method is suitable for improving the ability of thin-layer identification and also for the analysis of transient signals.
更多查看译文
关键词
Time-frequency analysis,S-transform (ST),Time sparse S-transform (TSST),Sparsity constraint
AI 理解论文
溯源树
样例
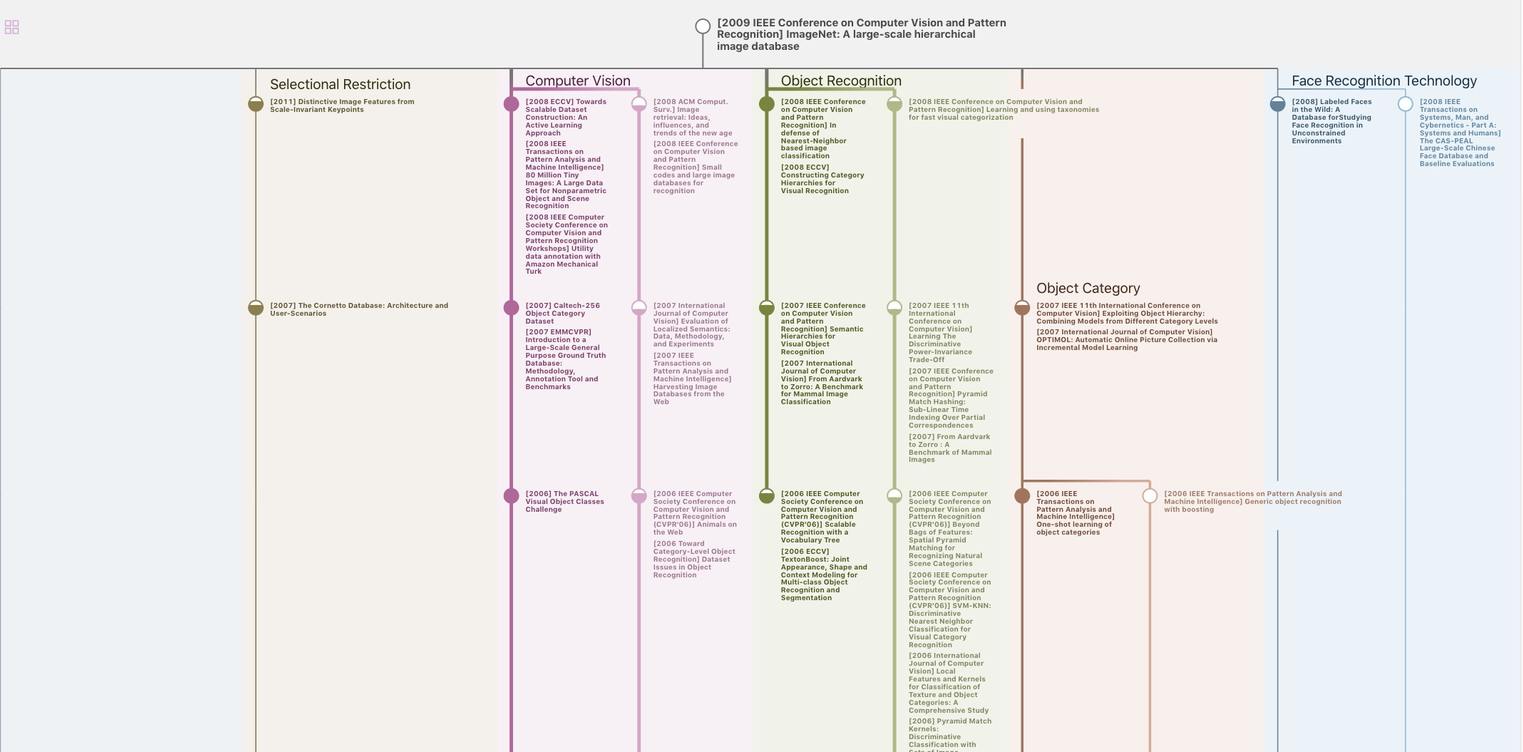
生成溯源树,研究论文发展脉络
Chat Paper
正在生成论文摘要