Unaligning Everything: or Aligning Any Text to Any Image in Multimodal Models
arxiv(2024)
摘要
Utilizing a shared embedding space, emerging multimodal models exhibit unprecedented zero-shot capabilities. However, the shared embedding space could lead to new vulnerabilities if different modalities can be misaligned. In this paper, we extend and utilize a recently developed effective gradient-based procedure that allows us to match the embedding of a given text by minimally modifying an image. Using the procedure, we show that we can align the embeddings of distinguishable texts to any image through unnoticeable adversarial attacks in joint image-text models, revealing that semantically unrelated images can have embeddings of identical texts and at the same time visually indistinguishable images can be matched to the embeddings of very different texts. Our technique achieves 100% success rate when it is applied to text datasets and images from multiple sources. Without overcoming the vulnerability, multimodal models cannot robustly align inputs from different modalities in a semantically meaningful way. Warning: the text data used in this paper are toxic in nature and may be offensive to some readers.
更多查看译文
AI 理解论文
溯源树
样例
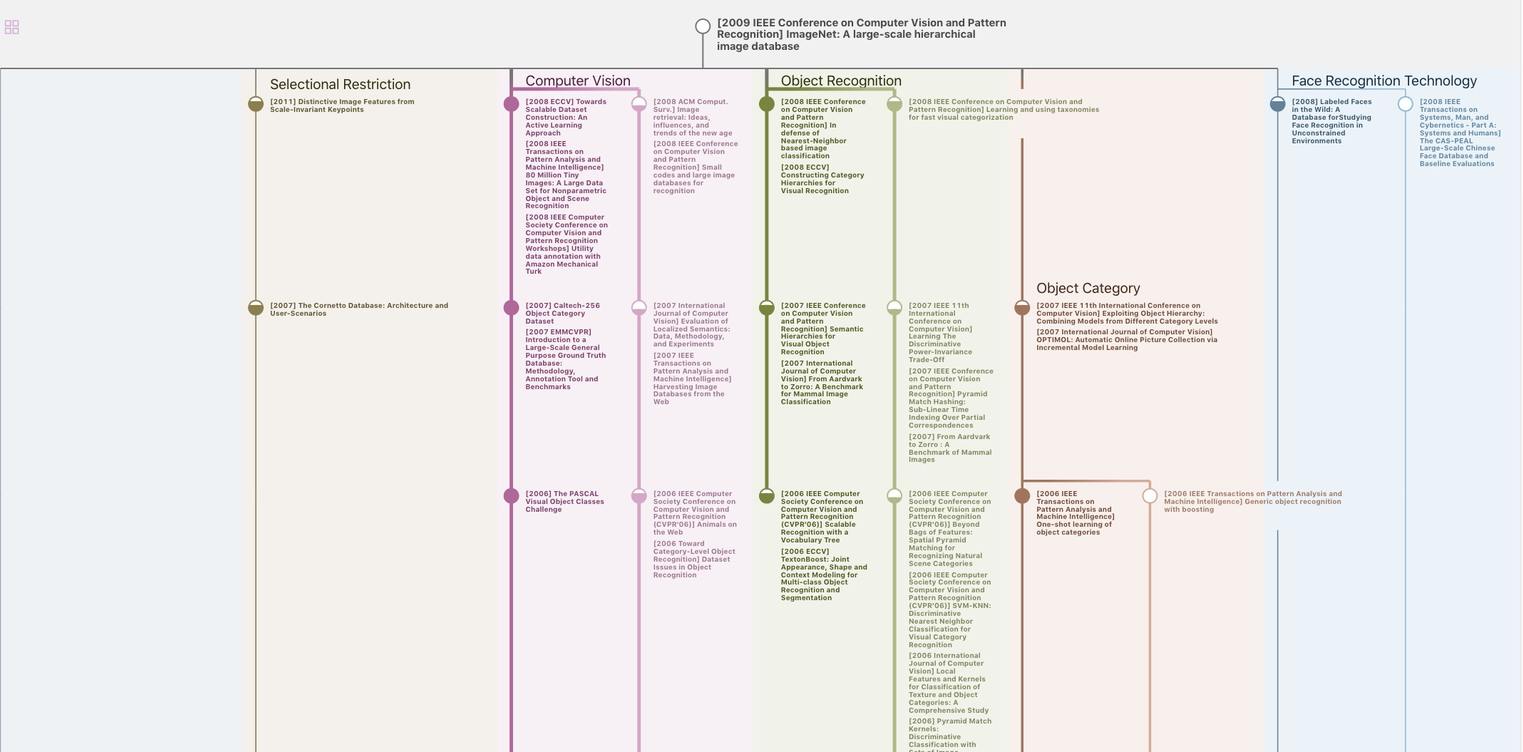
生成溯源树,研究论文发展脉络
Chat Paper
正在生成论文摘要