Beyond the Yield Barrier: Variational Importance Sampling Yield Analysis
arxiv(2024)
摘要
Optimal mean shift vector (OMSV)-based importance sampling methods have long
been prevalent in yield estimation and optimization as an industry standard.
However, most OMSV-based methods are designed heuristically without a rigorous
understanding of their limitations. To this end, we propose VIS, the first
variational analysis framework for yield problems, enabling a systematic
refinement for OMSV. For instance, VIS reveals that the classic OMSV is
suboptimal, and the optimal/true OMSV should always stay beyond the failure
boundary, which enables a free improvement for all OMSV-based methods
immediately. Using VIS, we show a progressive refinement for the classic OMSV
including incorporation of full covariance in closed form, adjusting for
asymmetric failure distributions, and capturing multiple failure regions, each
of which contributes to a progressive improvement of more than 2x. Inheriting
the simplicity of OMSV, the proposed method retains simplicity and robustness
yet achieves up to 29.03x speedup over the state-of-the-art (SOTA) methods. We
also demonstrate how the SOTA yield optimization, ASAIS, can immediately
benefit from our True OMSV, delivering a 1.20x and 1.27x improvement in
performance and efficiency, respectively, without additional computational
overhead.
更多查看译文
AI 理解论文
溯源树
样例
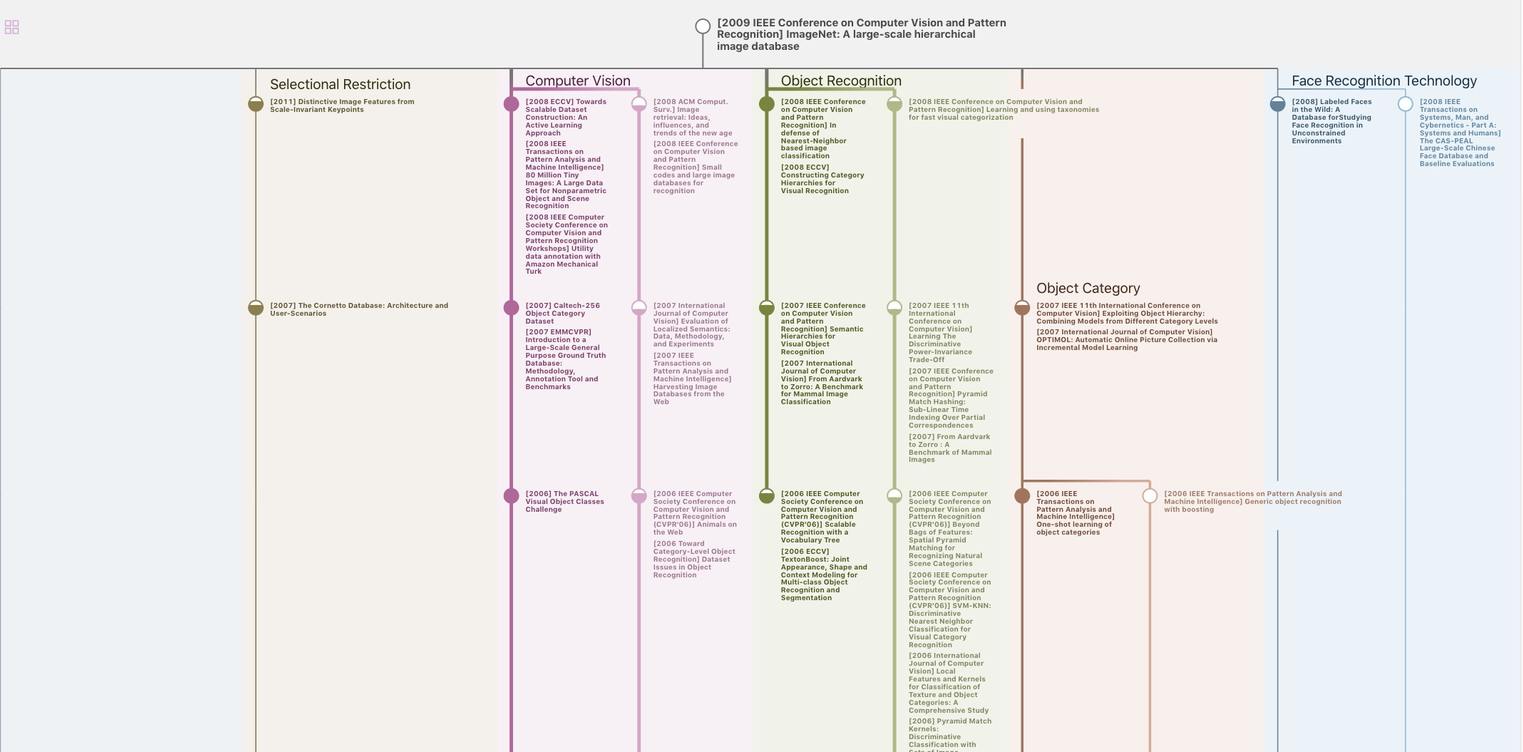
生成溯源树,研究论文发展脉络
Chat Paper
正在生成论文摘要