Novel camera self-calibration method with clustering prior and nonlinear optimization from an image sequence
Multimedia Tools and Applications(2024)
摘要
In many 3D reconstruction, Simultaneous Localization and Mapping (SLAM), AR, and VR systems, the camera focal length constantly changes slightly due to loose hardware, causing their performances to deteriorate. To address this problem, we propose a novel camera self-calibration method that incorporates clustering prior and nonlinear optimization. By leveraging the Kruppa equation, we roughly estimate multiple analytical solutions for the camera focal length from an image sequence. Then, robust clustering based on the Gaussian mixture model is introduced to obtain an initial camera focal length value. Finally, a nonlinear cost function is proposed to refine the initial camera focal length value. Extensive experimental results on simulation and real data demonstrate that the proposed method outperforms the state-of-the-art methods, exhibiting good robustness and high accuracy. Moreover, the proposed method can be used in real-time videos for SLAM systems.
更多查看译文
关键词
Camera self-calibration,Kruppa equation,Clustering prior,Nonlinear optimization
AI 理解论文
溯源树
样例
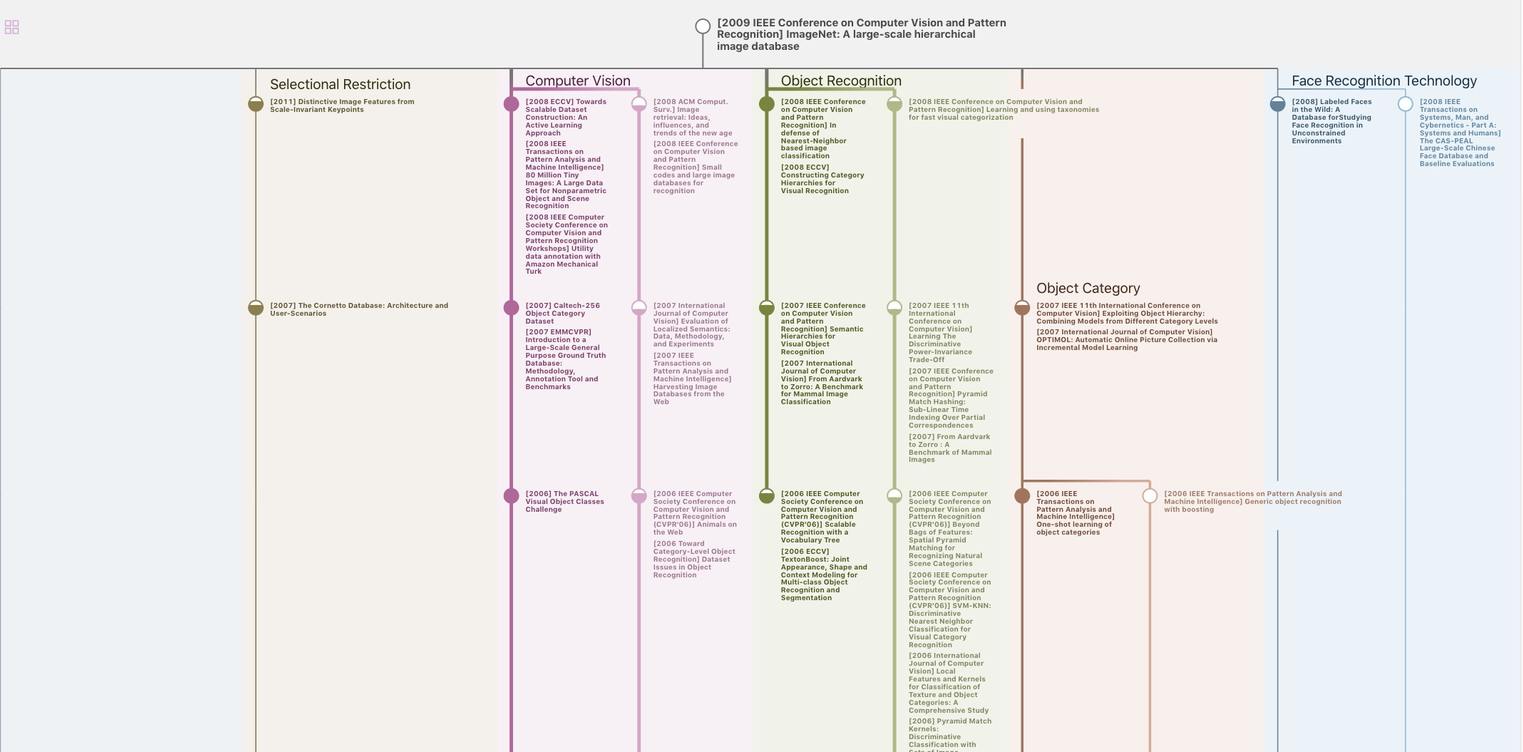
生成溯源树,研究论文发展脉络
Chat Paper
正在生成论文摘要