Evaluation of Classical and Deep Learning Approaches for Human Activity Recognition.
International Convention on Information and Communication Technology, Electronics and Microelectronics(2024)
摘要
The main idea of the internet of things is to have devices connected and working together for the benefits of human, and it is being realized more with each day, as the number of devices with inbuilt processors, sensors and wireless connectivity increases. One of the most widely spread applications is human activity recognition, which enables applications to make personalized recommendations about healthy living habits. In order to accomplish that, algorithms that are able to recognize human activities have evolved. Traditionally, this is done through feature extraction of handcrafted features for specific sets of activities and use-cases (classical approaches). Although these methods achieve great performance, expansion of neural networks is shifting this task away from being feature engineering towards being neural network engineering. In this paper we compare several already existing classical approaches, deep learning approaches, and a newly developed AutoPyTorch library that enables a combination of traditional and neural network approaches for human activity recognition tasks. The algorithms were not fine-tuned and results might vary depending on the hyperparameters, but they provide an insight about general patterns as well as upsides and downsides of using different approaches for human activity recognition.
更多查看译文
关键词
human activity recognition,feature extraction,neural networks,internet of things,classification
AI 理解论文
溯源树
样例
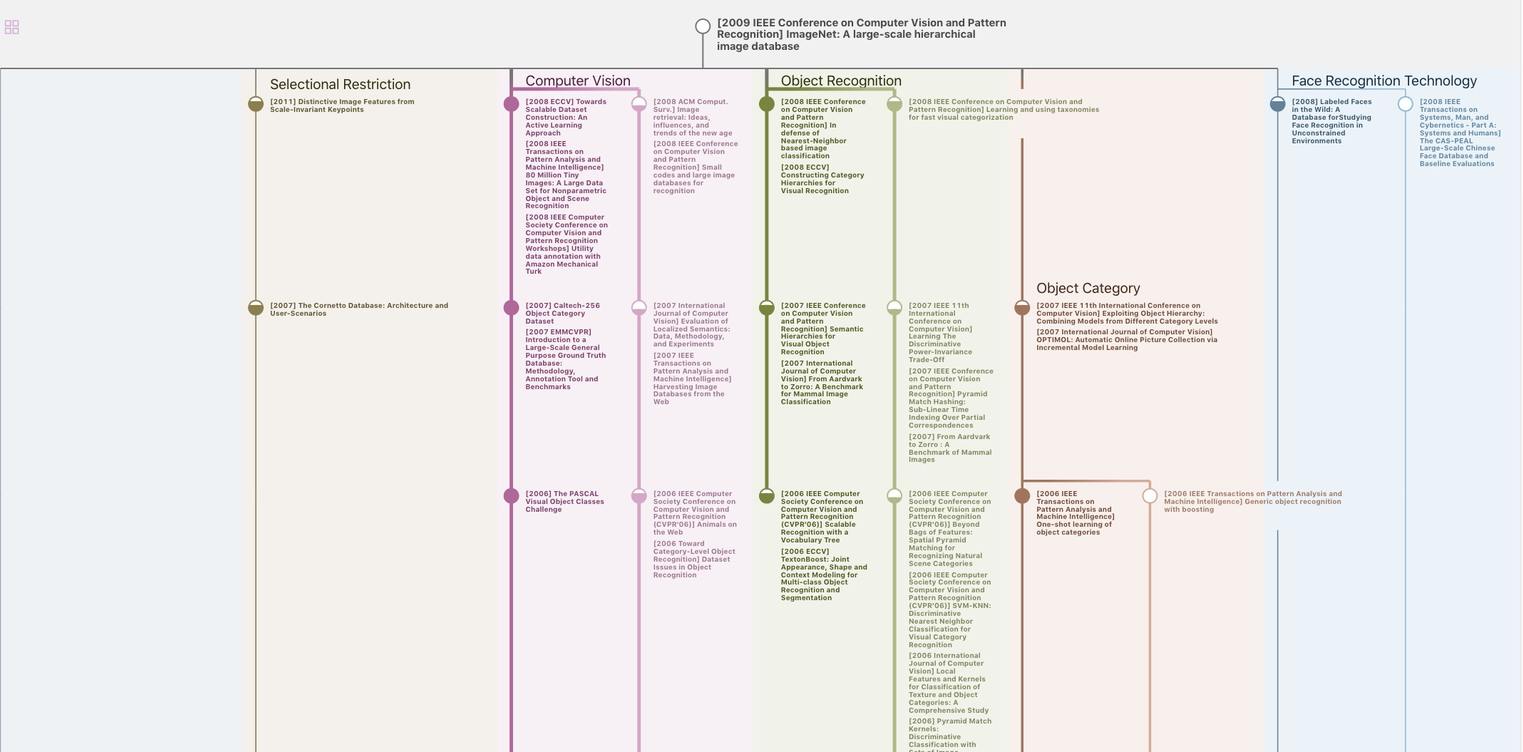
生成溯源树,研究论文发展脉络
Chat Paper
正在生成论文摘要