Wind Turbine Blade Fault Detection via Thermal Imaging Using Deep Learning
2024 Intermountain Engineering, Technology and Computing (IETC)(2024)
Abstract
This research focuses on leveraging fusion imaging, which combines thermal and RGB data, for the inspection of Wind Turbine Blades. We introduce a novel dataset comprising 1000 fused images of a Primus Air Max wind turbine and analyze it using advanced deep learning models including AlexNet, VGG, ResNet, Xception, and DenseNet. Among these, DenseNet emerges as the superior model, demonstrating exceptional capability in detecting defects in WTBs. DenseNet's architecture, characterized by its dense connectivity pattern that ensures each layer is directly connected to all subsequent layers, significantly enhances feature extraction and reduces false negative rates. This makes it an ideal choice for automating and improving the accuracy of wind turbine maintenance processes.
MoreTranslated text
Key words
deep learning,data fusion,thermal imaging,wind turbine blades,defect detection,image classification
AI Read Science
Must-Reading Tree
Example
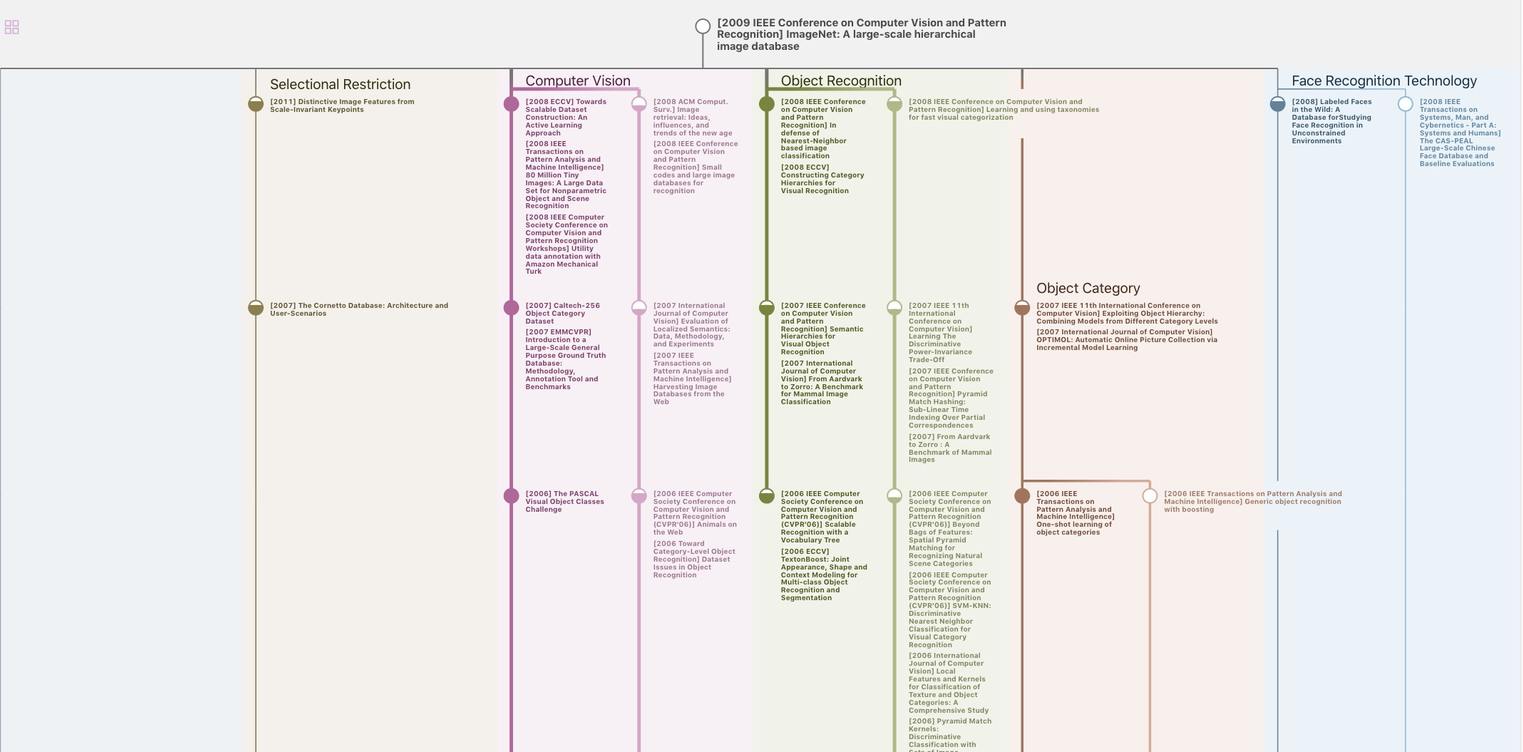
Generate MRT to find the research sequence of this paper
Chat Paper
Summary is being generated by the instructions you defined