Probabilistic capacity energy-based machine learning models for soil liquefaction reliability analysis
Engineering Geology(2024)
Abstract
The energy-based method has been widely employed to evaluate soil liquefaction potential. A recent trend is to develop machine learning (ML) models to predict capacity energy. However, there is still a lack of statistical perspectives on energy-based ML models for liquefaction risk analysis. This study develops a rigorous Bayesian framework for characterizing uncertainties of two typical energy-based ML models and developing probabilistic versions. Performance of ML models is evaluated and compared from a statistical perspective using Bayesian model comparison approach. The effects of test data quality and data quantity are also comprehensively investigated. The probabilistic model is finally verified against real cases. Results show that the occurrence probability of artificial neural network model is higher than that of support vector machine model. The model uncertainty is more sensitive to uncertainties in soil textural properties and fines content than inter-granular contact density. The predicted liquefaction probabilities are in good agreement with field observations and superior to the traditional method. This study can extend the use of the energy-based methods and provide performance-based risk evaluation of soil liquefaction potential.
MoreTranslated text
Key words
Liquefaction,Capacity energy,Machine learning,Uncertainty,Reliability analysis
AI Read Science
Must-Reading Tree
Example
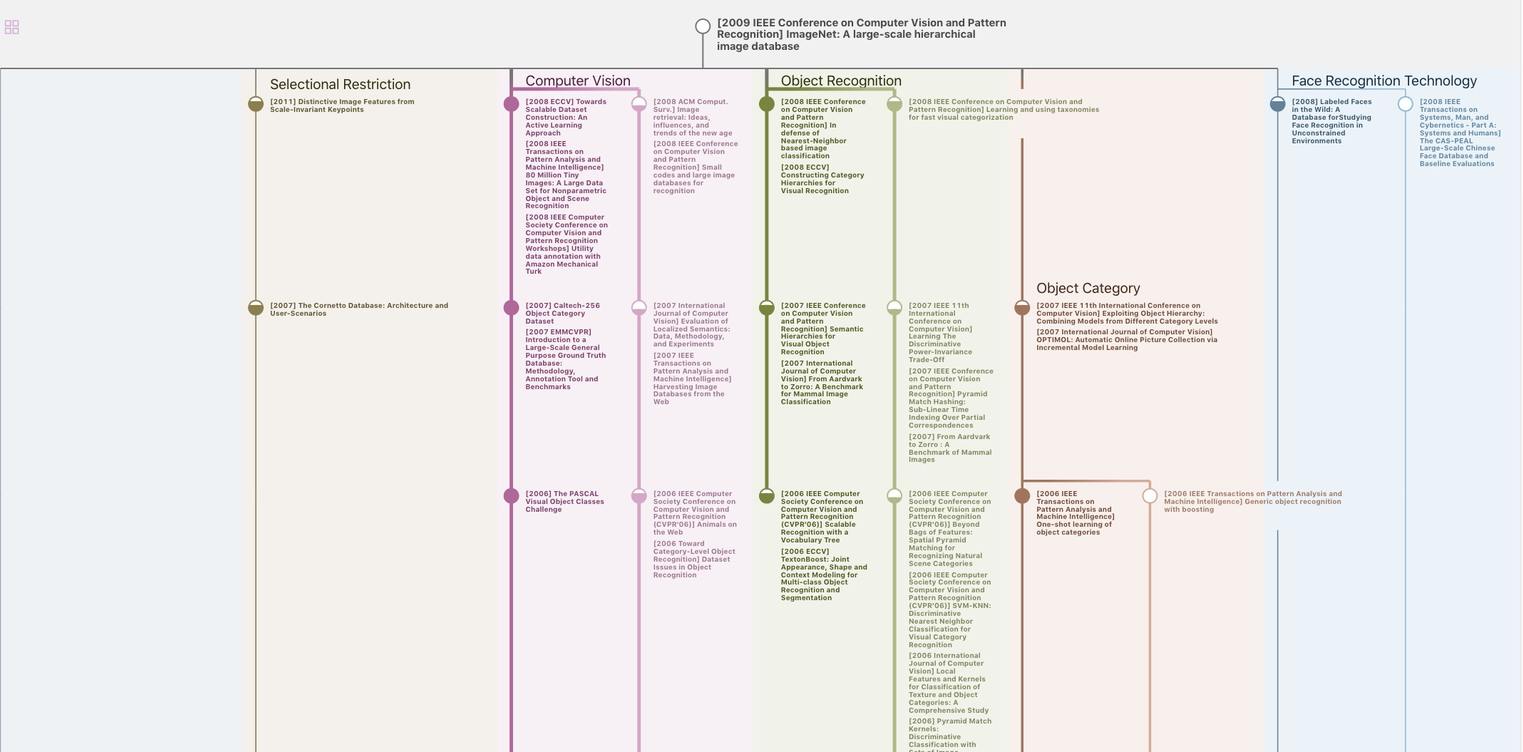
Generate MRT to find the research sequence of this paper
Chat Paper
Summary is being generated by the instructions you defined