Disentangled Cross-modal Fusion for Event-guided Image Super-Resolution
IEEE Transactions on Artificial Intelligence(2024)
摘要
Event cameras detect the intensity changes and produce asynchronous events with high dynamic range and no motion blur. Recently, several attempts have been made to super-resolve the intensity images guided by events. However, these methods directly fuse the event and image features without distinguishing the modality difference and achieve image super-resolution (SR) in multiple steps, leading to error-prone image SR results. Also, they lack quantitative evaluation of real-world data. In this paper, we present an
end-to-end
framework, called
EGI-SR
to narrow the modality gap and subtly integrate the event and RGB modality features for effective image SR. Specifically, EGI-SR employs three Cross-Modality Encoders (CME) to learn modality-specific and modality-shared features from the stacked events and the intensity image, respectively. As such, EGI-SR can better mitigate the negative impact of modality varieties and reduce the difference in the feature space between the events and the intensity image. Subsequently, a transformer-based decoder is deployed to reconstruct the SR image. Moreover, we collect a real-world dataset, with temporally and spatially aligned events and color image pairs. We conduct extensive experiments on the synthetic and real-world datasets, showing EGI-SR favorably surpassing the existing methods by a large margin.
更多查看译文
关键词
Event-based vision,feature fusion,image SR
AI 理解论文
溯源树
样例
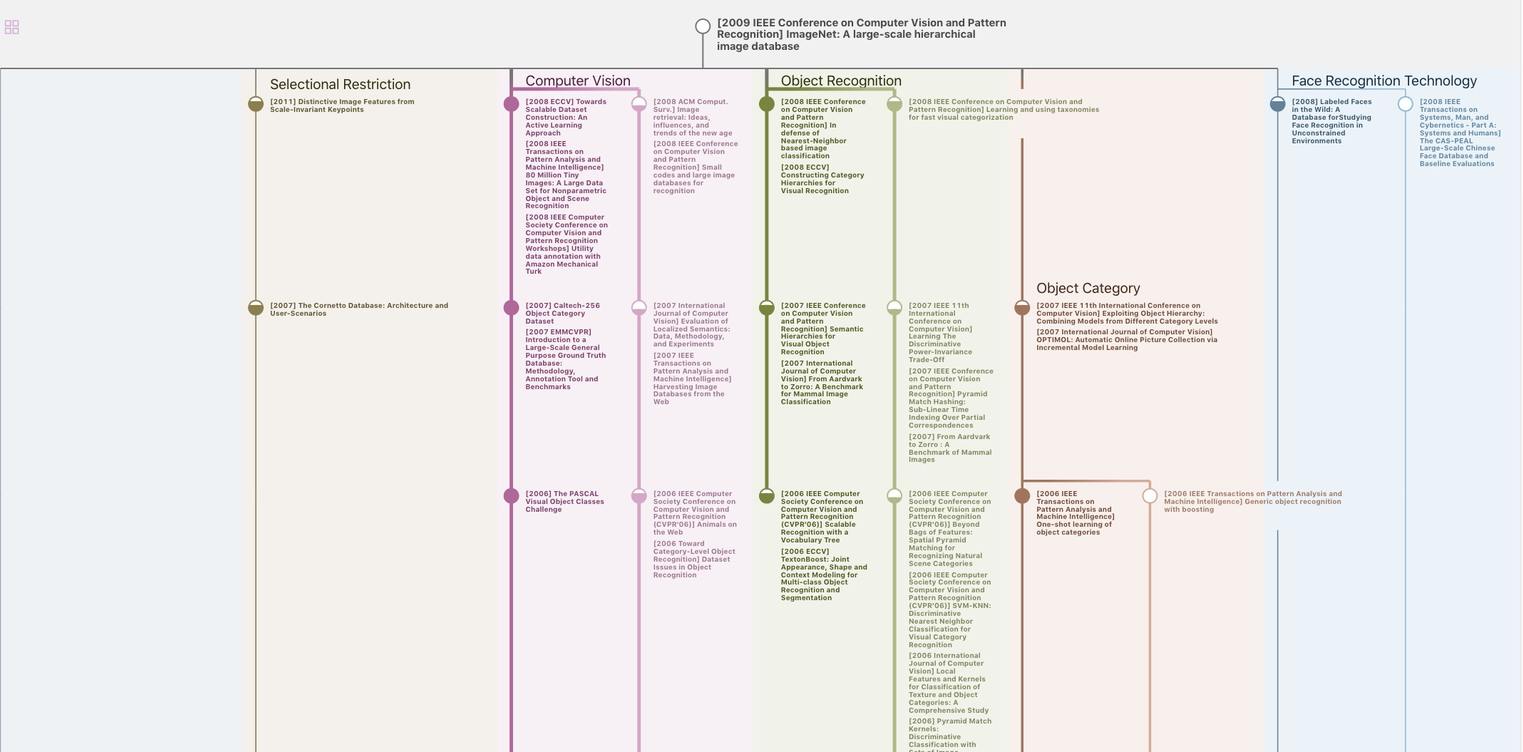
生成溯源树,研究论文发展脉络
Chat Paper
正在生成论文摘要