Mitigating Racial Biases for Machine Learning Based Skin Cancer Detection
PROCEEDINGS OF THE 2023 INTERNATIONAL SYMPOSIUM ON THEORY, ALGORITHMIC FOUNDATIONS, AND PROTOCOL DESIGN FOR MOBILE NETWORKS AND MOBILE COMPUTING, MOBIHOC 2023(2023)
Abstract
Machine learning (ML) based skin cancer detection tools are an example of a transformative medical technology that could potentially democratize early detection for skin cancer cases for everyone. However, due to the dependency of datasets for training, ML based skin cancer detection always suffers from a systemic racial bias. Racial communities and ethnicity not well represented within the training datasets will not be able to use these tools, leading to health disparities being amplified. Based on empirical observations we posit that skin cancer training data is biased as it's dataset represents mostly communities of lighter skin tones, despite skin cancer being far more lethal for people of color. In this paper we use domain adaptation techniques by employing CycleGANs to mitigate racial biases existing within state of the art machine learning based skin cancer detection tools by adapting minority images to appear as the majority. Using our domain adaptation techniques to augment our minority datasets, we are able to improve the accuracy, precision, recall, and F1 score of typical image classification machine learning models for skin cancer classification from the biased 50% accuracy rate to a 79% accuracy rate when testing on minority skin tone images. We evaluate and demonstrate a proof-of-concept smartphone application.
MoreTranslated text
Key words
skin cancer,machine learning,domain adaptation,racial,skin tones,artificial intelligence,bias,computer vision,app,generative adversarial networks
AI Read Science
Must-Reading Tree
Example
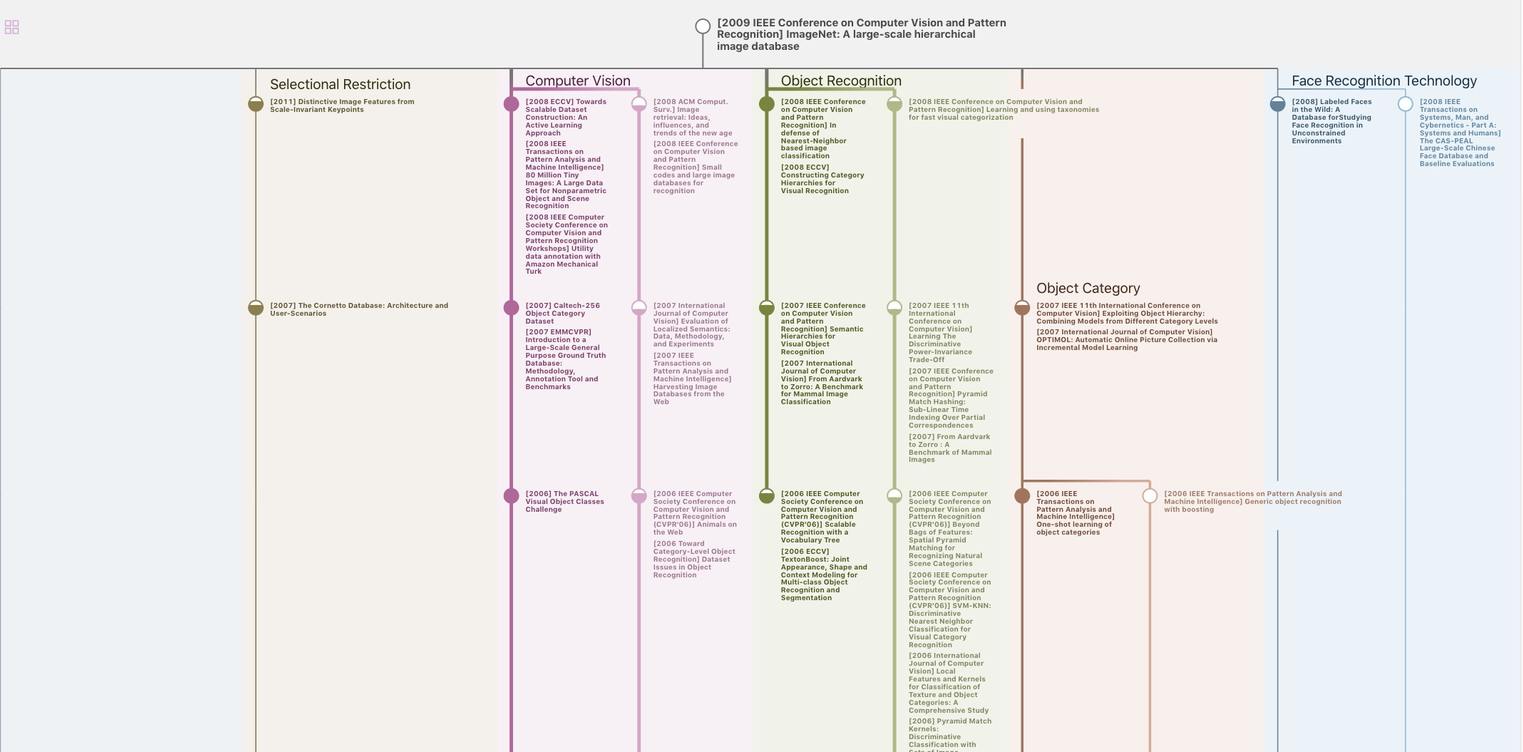
Generate MRT to find the research sequence of this paper
Chat Paper
Summary is being generated by the instructions you defined