Data-Driven Cycle Life Prediction of Lithium Metal-Based Rechargeable Battery Based on Discharge/Charge Capacity and Relaxation Features.
Advanced science (Weinheim, Baden-Wurttemberg, Germany)(2024)
Abstract
Achieving precise estimates of battery cycle life is a formidable challenge due to the nonlinear nature of battery degradation. This study explores an approach using machine learning (ML) methods to predict the cycle life of lithium-metal-based rechargeable batteries with high mass loading LiNi0.8Mn0.1Co0.1O2 electrode, which exhibits more complicated and electrochemical profile during battery operating conditions than typically studied LiFePO₄/graphite based rechargeable batteries. Extracting diverse features from discharge, charge, and relaxation processes, the intricacies of cell behavior without relying on specific degradation mechanisms are navigated. The best-performing ML model, after feature selection, achieves an R2 of 0.89, showcasing the application of ML in accurately forecasting cycle life. Feature importance analysis unveils the logarithm of the minimum value of discharge capacity difference between 100 and 10 cycle (Log(|min(ΔDQ 100-10(V))|)) as the most important feature. Despite the inherent challenges, this model demonstrates a remarkable 6.6% test error on unseen data, underscoring its robustness and potential for transformative advancements in battery management systems. This study contributes to the successful application of ML in the realm of cycle life prediction for lithium-metal-based rechargeable batteries with practically high energy density design.
MoreTranslated text
AI Read Science
Must-Reading Tree
Example
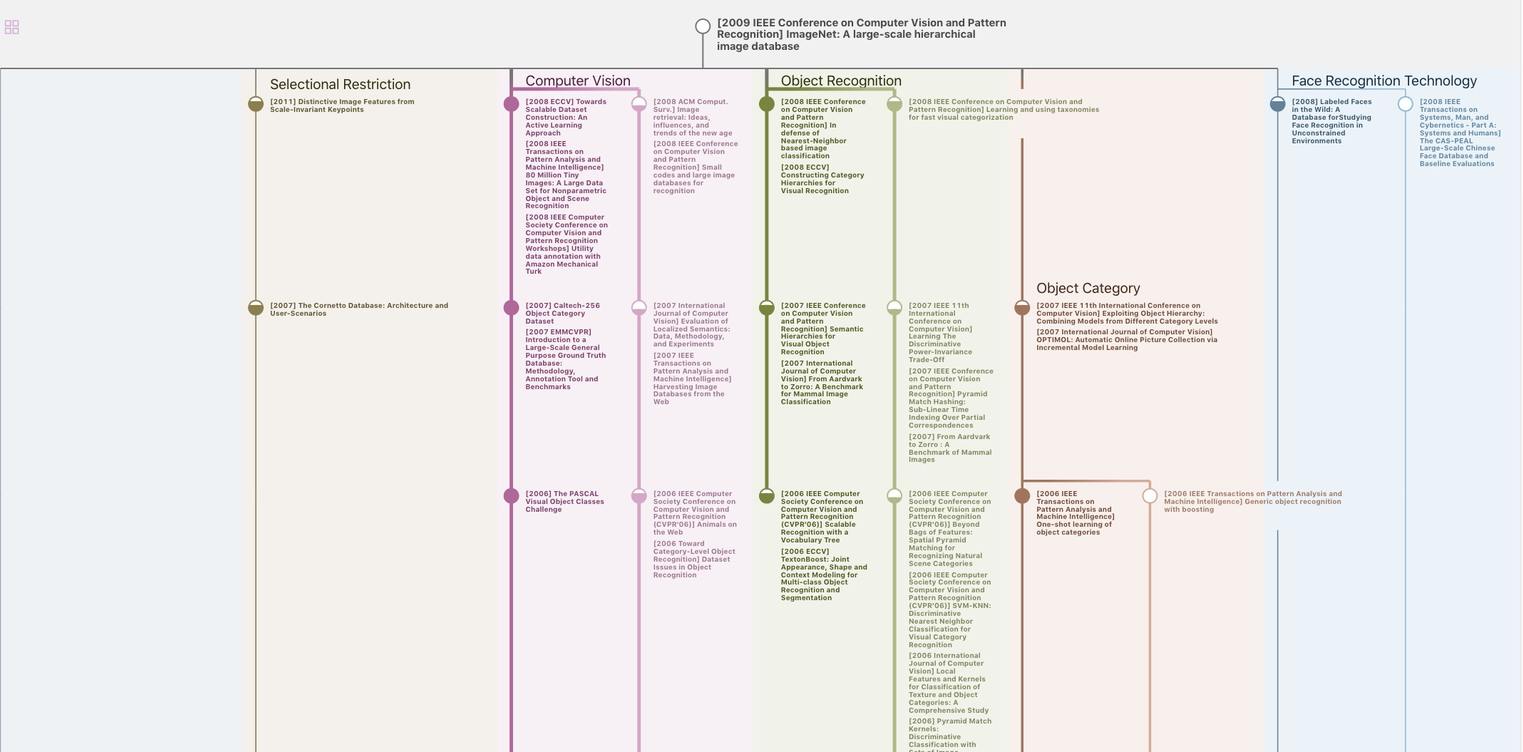
Generate MRT to find the research sequence of this paper
Chat Paper
Summary is being generated by the instructions you defined