Interaction Difference Hypothesis Test for Prediction Models
Machine Learning and Knowledge Extraction(2024)
摘要
Machine learning research focuses on the improvement of prediction performance. Progress was made with black-box models that flexibly adapt to the given data. However, due to their increased complexity, black-box models are more difficult to interpret. To address this issue, techniques for interpretable machine learning have been developed, yet there is still a lack of methods to reliably identify interaction effects between predictors under uncertainty. In this work, we present a model-agnostic hypothesis test for the identification of interaction effects in black-box machine learning models. The test statistic is based on the difference between the variance of the estimated prediction function and a version of the estimated prediction function without interaction effects derived via partial dependence functions. The properties of the proposed hypothesis test were explored in simulations of linear and nonlinear models. The proposed hypothesis test can be applied to any black-box prediction model, and the null hypothesis of the test can be flexibly specified according to the research question of interest. Furthermore, the test is computationally fast to apply, as the null distribution does not require the resampling or refitting of black-box prediction models.
更多查看译文
关键词
prediction models,interpretable machine learning,model-agnostic,hypothesis tests,interaction effects
AI 理解论文
溯源树
样例
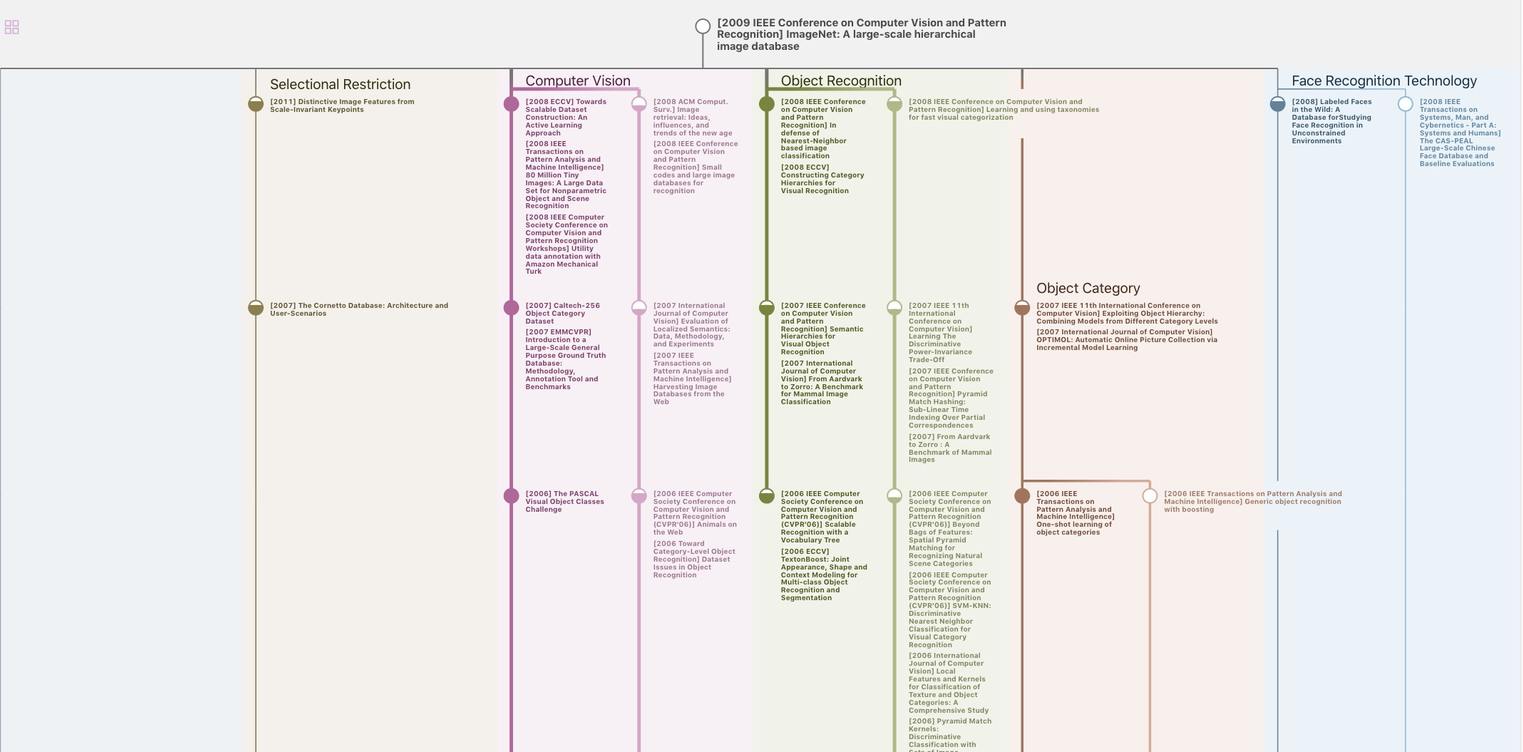
生成溯源树,研究论文发展脉络
Chat Paper
正在生成论文摘要