ACE: A Generative Cross-Modal Retrieval Framework with Coarse-To-Fine Semantic Modeling
CoRR(2024)
Abstract
Generative retrieval, which has demonstrated effectiveness in text-to-text
retrieval, utilizes a sequence-to-sequence model to directly generate candidate
identifiers based on natural language queries. Without explicitly computing the
similarity between queries and candidates, generative retrieval surpasses
dual-tower models in both speed and accuracy on large-scale corpora, providing
new insights for cross-modal retrieval. However, constructing identifiers for
multimodal data remains an untapped problem, and the modality gap between
natural language queries and multimodal candidates hinders retrieval
performance due to the absence of additional encoders. To this end, we propose
a pioneering generAtive Cross-modal rEtrieval framework (ACE), which is a
comprehensive framework for end-to-end cross-modal retrieval based on
coarse-to-fine semantic modeling. We propose combining K-Means and RQ-VAE to
construct coarse and fine tokens, serving as identifiers for multimodal data.
Correspondingly, we design the coarse-to-fine feature fusion strategy to
efficiently align natural language queries and candidate identifiers. ACE is
the first work to comprehensively demonstrate the feasibility of generative
approach on text-to-image/audio/video retrieval, challenging the dominance of
the embedding-based dual-tower architecture. Extensive experiments show that
ACE achieves state-of-the-art performance in cross-modal retrieval and
outperforms the strong baselines on Recall@1 by 15.27
MoreTranslated text
AI Read Science
Must-Reading Tree
Example
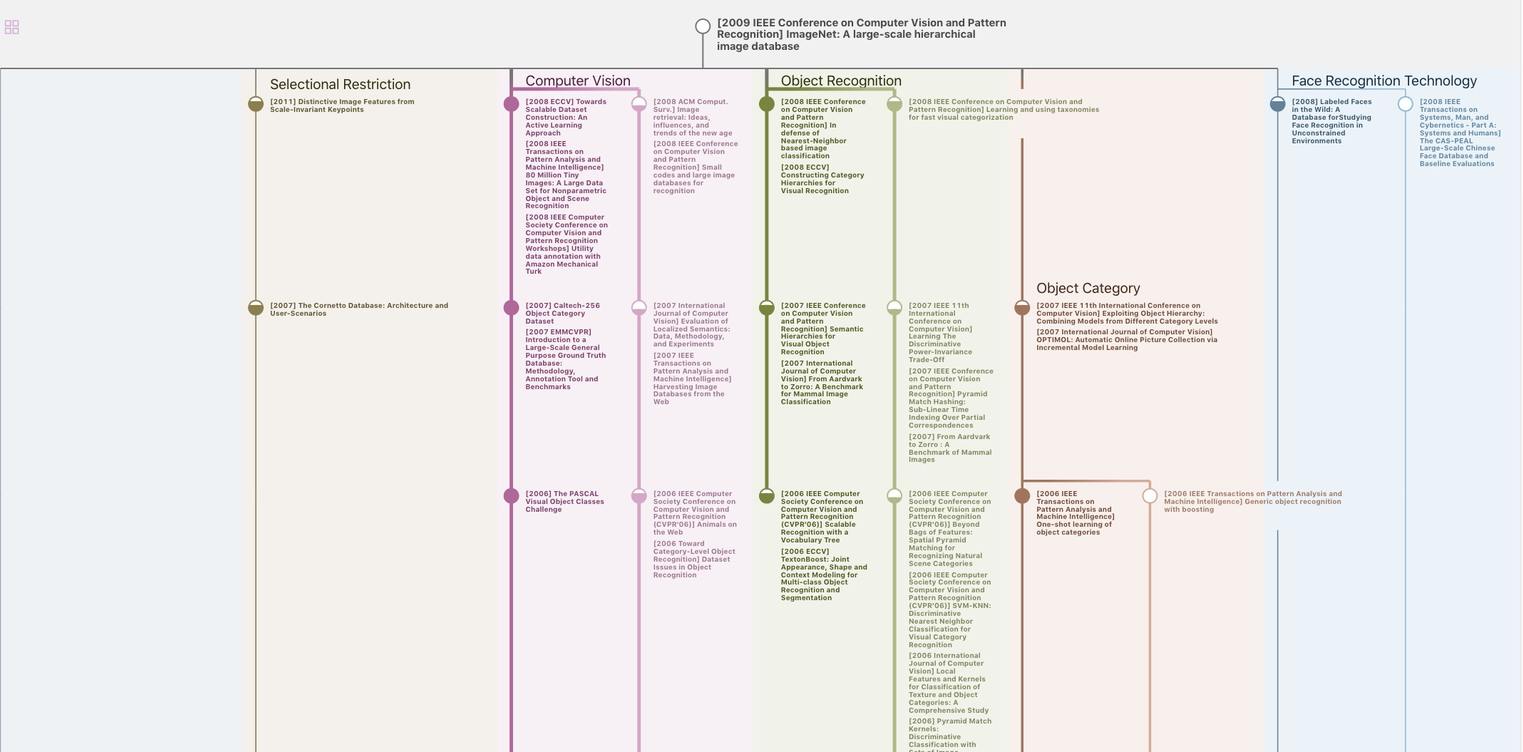
Generate MRT to find the research sequence of this paper
Chat Paper
Summary is being generated by the instructions you defined