Private Heterogeneous Federated Learning Without a Trusted Server Revisited: Error-Optimal and Communication-Efficient Algorithms for Convex Losses
ICML 2024(2024)
Abstract
We revisit the problem of federated learning (FL) with private data from people who do not trust the server or other silos/clients. In this context, every silo (e.g. hospital) has data from several people (e.g. patients) and needs to protect the privacy of each person's data (e.g. health records), even if the server and/or other silos try to uncover this data. Inter-Silo Record-Level Differential Privacy (ISRL-DP) prevents each silo's data from being leaked, by requiring that silo $i$'s *communications* satisfy item-level differential privacy. Prior work (Lowy & Razaviyayn, 2023a) characterized the optimal excess risk bounds for ISRL-DP algorithms with *homogeneous* (i.i.d.) silo data and convex loss functions. However, two important questions were left open: 1) Can the same excess risk bounds be achieved with *heterogeneous* (non-i.i.d.) silo data? 2) Can the optimal risk bounds be achieved with *fewer communication rounds*? In this paper, we give positive answers to both questions. We provide novel ISRL-DP FL algorithms that achieve the optimal excess risk bounds in the presence of heterogeneous silo data. Moreover, our algorithms are more *communication-efficient* than the prior state-of-the-art. For smooth loss functions, our algorithm achieves the *optimal* excess risk bound and has *communication complexity that matches the non-private lower bound*. Additionally, our algorithms are more *computationally efficient* than the previous state-of-the-art.
MoreTranslated text
AI Read Science
Must-Reading Tree
Example
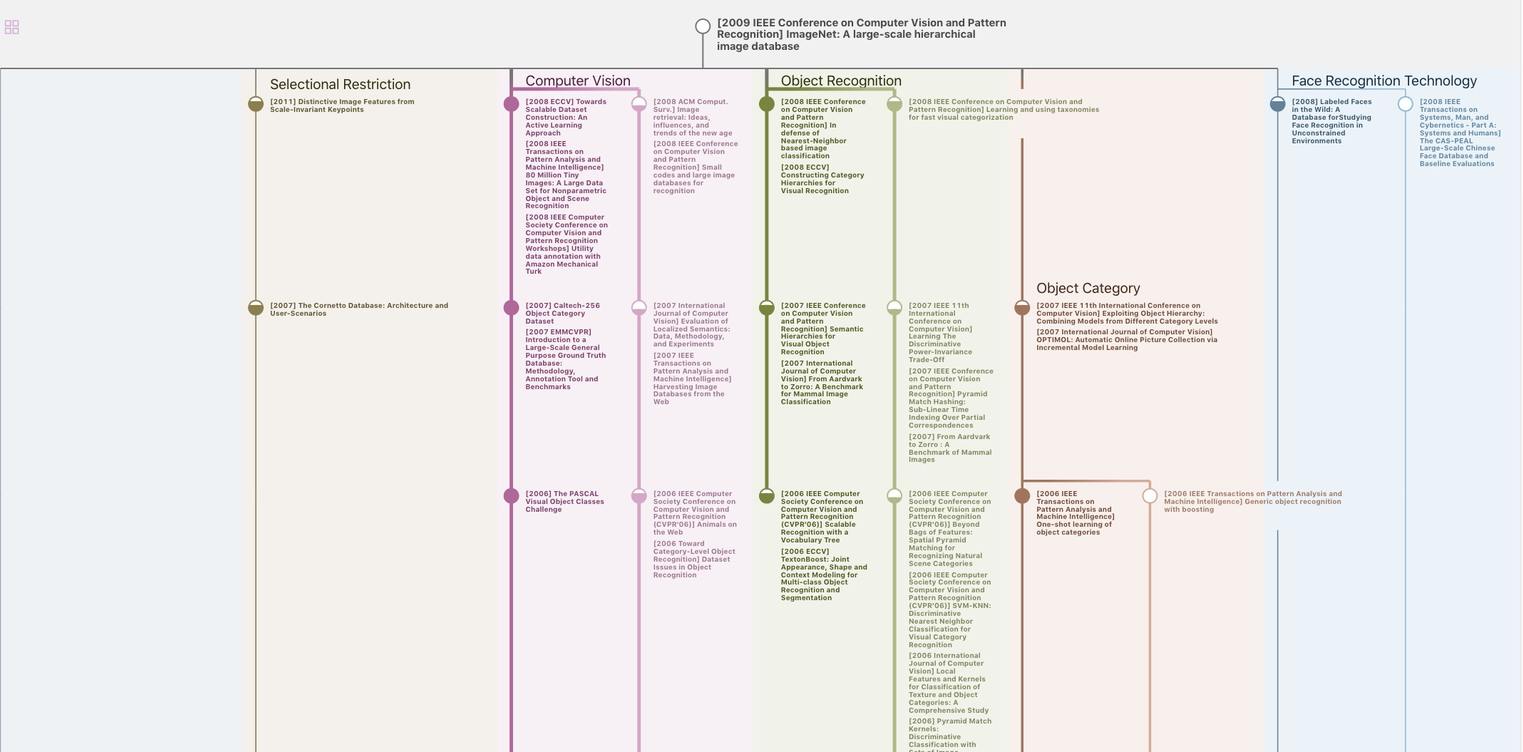
Generate MRT to find the research sequence of this paper
Chat Paper
Summary is being generated by the instructions you defined