Offline Transition Modeling via Contrastive Energy Learning
ICML 2024(2024)
Abstract
Learning a high-quality transition model is of great importance for sequential decision-making tasks, especially in offline settings. Nevertheless, the complex behaviors of transition dynamics in real-world environments pose challenges for the standard forward models because of their inductive bias towards smooth regressors, conflicting with the inherent nature of transitions such as discontinuity or large curvature. In this work, we propose to model the transition probability implicitly through a scalar-value energy function, which enables not only flexible distribution prediction but also capturing complex transition behaviors. The Energy-based Transition Models (ETM) are shown to accurately fit the discontinuous transition functions and better generalize to out-of-distribution transition data. Furthermore, we demonstrate that energy-based transition models improve the evaluation accuracy and significantly outperform other off-policy evaluation methods in DOPE benchmark. Finally, we show that energy-based transition models also benefit reinforcement learning and outperform prior offline RL algorithms in D4RL Gym-Mujoco tasks.
MoreTranslated text
AI Read Science
Must-Reading Tree
Example
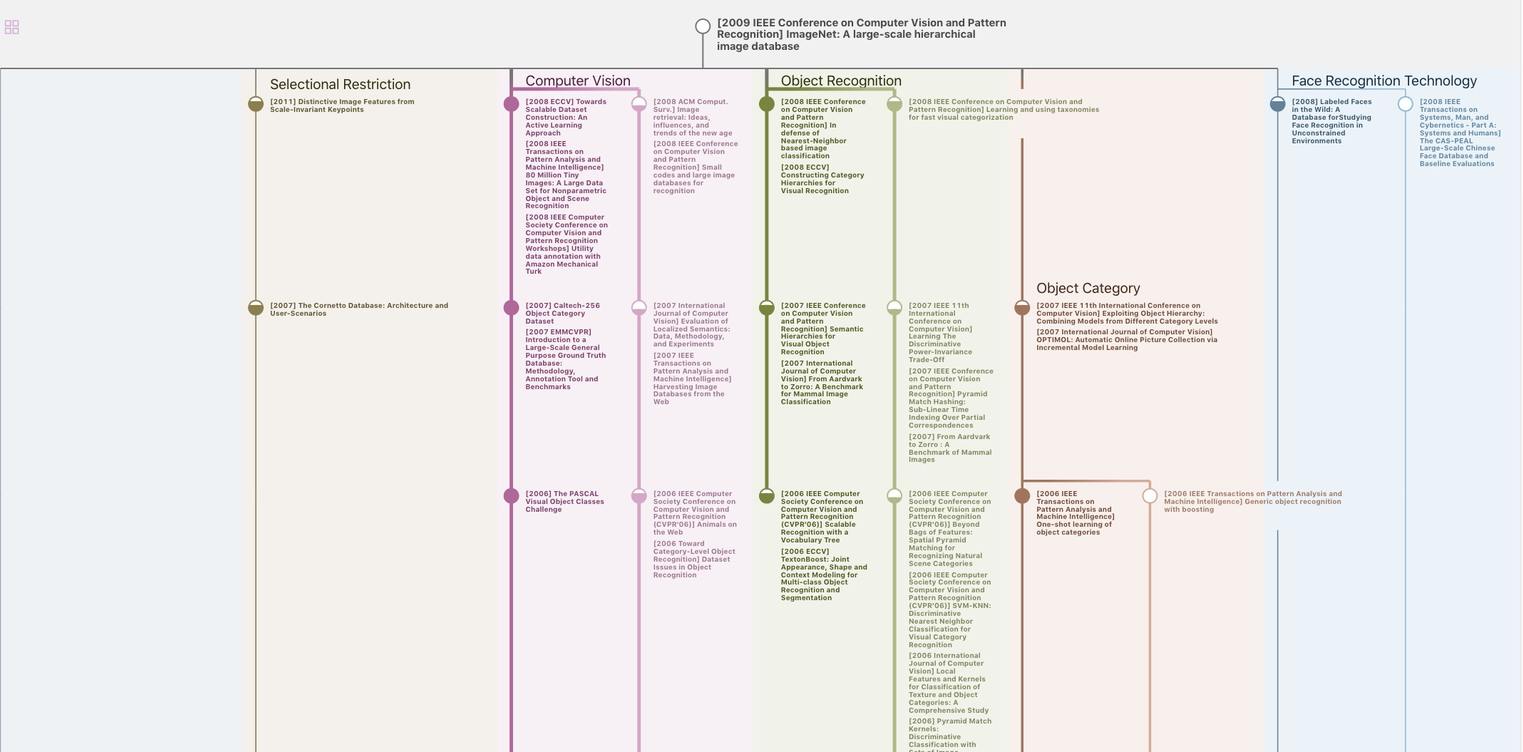
Generate MRT to find the research sequence of this paper
Chat Paper
Summary is being generated by the instructions you defined