Revealing the Dark Secrets of Extremely Large Kernel ConvNets on Robustness
ICML 2024(2024)
Abstract
Robustness is a vital aspect to consider when deploying deep learning models into the wild. Numerous studies have been dedicated to the study of the robustness of vision transformers (ViTs), which have dominated as the mainstream backbone choice for vision tasks since the dawn of 2020s. Recently, some large kernel convnets make a comeback with impressive performance and efficiency. However, it still remains unclear whether large kernel networks are robust and the attribution of their robustness. In this paper, we first conduct a comprehensive evaluation of large kernel convnets' robustness and their differences from typical small kernel counterparts and ViTs on six diverse robustness benchmark datasets. Then to analyze the underlying factors behind their strong robustness, we design experiments from both quantitative and qualitative perspectives to reveal large kernel convnets' intriguing properties that are completely different from typical convnets. Our experiments demonstrate for the first time that pure CNNs can achieve exceptional robustness comparable or even superior to that of ViTs. Our analysis on occlusion invariance, kernel attention patterns and frequency characteristics provide novel insights into the source of robustness. Code available at: https://github.com/Lauch1ng/LKRobust.
MoreTranslated text
AI Read Science
Must-Reading Tree
Example
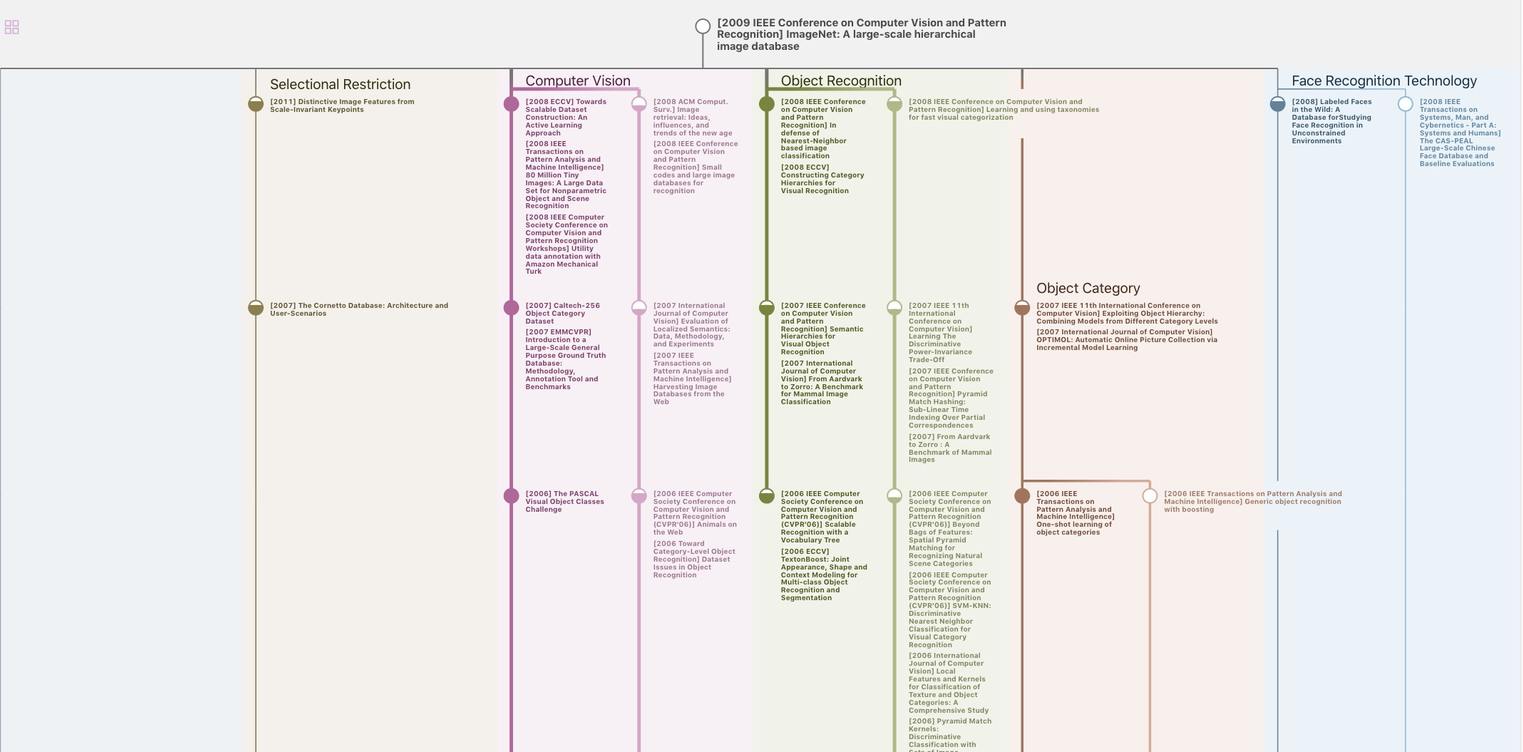
Generate MRT to find the research sequence of this paper
Chat Paper
Summary is being generated by the instructions you defined