Multimodal Graph Benchmark
CoRR(2024)
Abstract
Associating unstructured data with structured information is crucial for
real-world tasks that require relevance search. However, existing graph
learning benchmarks often overlook the rich semantic information associate with
each node. To bridge such gap, we introduce the Multimodal Graph Benchmark
(MM-GRAPH), the first comprehensive multi-modal graph benchmark that
incorporates both textual and visual information. MM-GRAPH surpasses previous
efforts, which have primarily focused on text-attributed graphs with various
connectivity patterns. MM-GRAPH consists of five graph learning datasets of
various scales that are appropriate for different learning tasks. Their
multimodal node features, enabling a more comprehensive evaluation of graph
learning algorithms in real-world scenarios. To facilitate research on
multimodal graph learning, we further provide an extensive study on the
performance of various graph neural networks in the presence of features from
various modalities. MM-GRAPH aims to foster research on multimodal graph
learning and drive the development of more advanced and robust graph learning
algorithms. By providing a diverse set of datasets and benchmarks, MM-GRAPH
enables researchers to evaluate and compare their models in realistic settings,
ultimately leading to improved performance on real-world applications that rely
on multimodal graph data.
MoreTranslated text
AI Read Science
Must-Reading Tree
Example
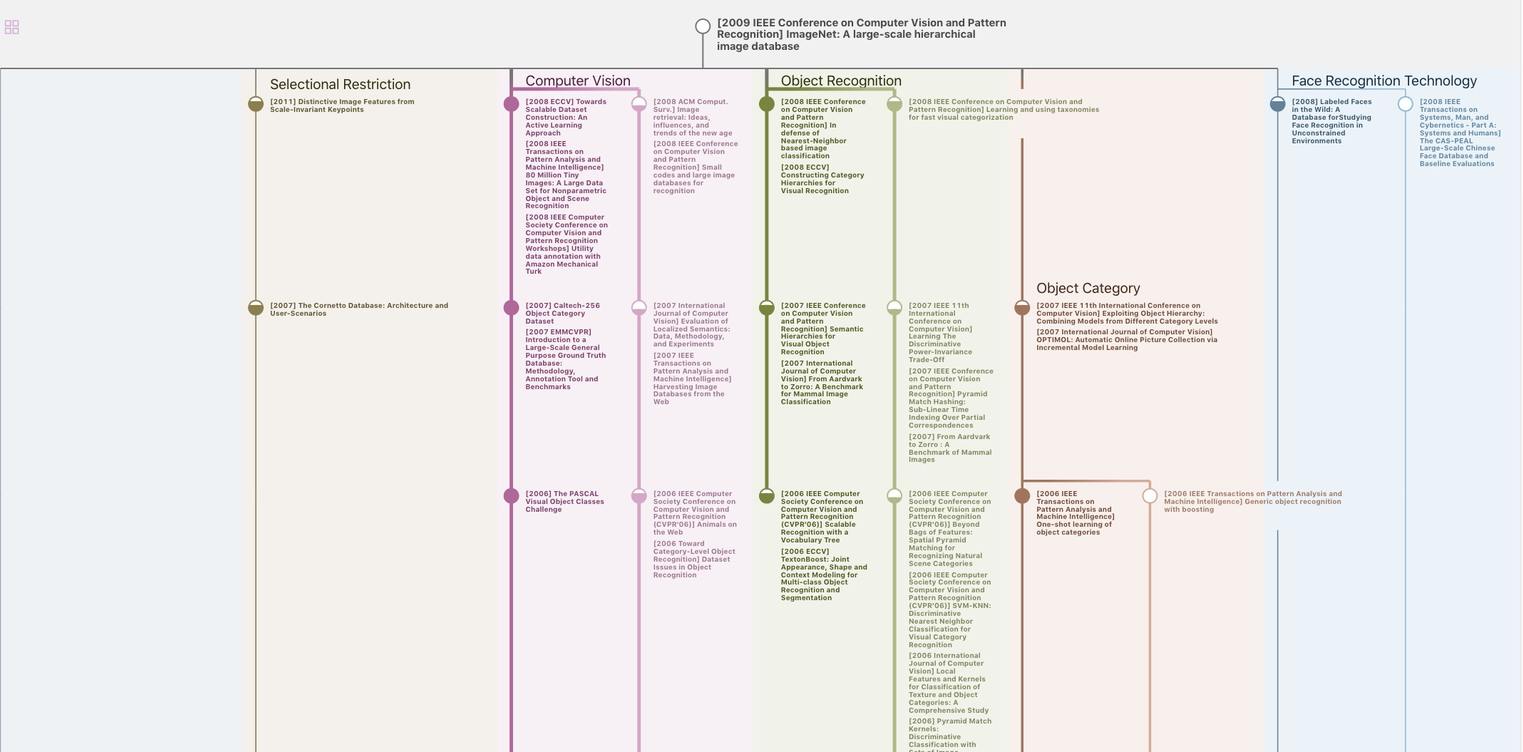
Generate MRT to find the research sequence of this paper
Chat Paper
Summary is being generated by the instructions you defined